A semantically driven self-supervised algorithm for detecting anomalies in image sets
Computer Vision and Image Understanding(2021)
摘要
Humans can readily detect when an image does not belong in a set by comparing semantic information between images to derive meaning and relationships from the colors, shapes, sizes, locations, and textures within them. Current self-supervised anomaly detection algorithms in computer vision do not possess this ability. Most algorithms learn to detect anomalies through a training objective that only optimizes for machine-level features and do not evaluate semantic features. To fill this gap, we propose a novel self-supervised algorithm that detects anomalies by learning and modeling semantic information within a set of images. This is accomplished by first training our algorithm to be sensitive or invariant to targeted semantic information, and then modeling the semantic relationships learned so that we can detect anomalies by measuring how far images deviate from the model. Experimenting with our algorithm, we show that different datasets can have different semantic sensitivities, those sensitivities can fluctuate between and within sets depending on different aspects of the images, and directly targeting those sensitivities can improve anomaly detection performance. Using our algorithm, we were able to achieve a AUROC of 0.7146 on the CIFAR-10 dataset, which is an improvement of 0.0741 over another current leading self-supervised anomaly detection algorithm.
更多查看译文
关键词
Anomaly detection,Self-supervised learning,Representation learning,Remote sensing,Computer vision,Land use classification,Pretext learning,Feature extraction,Dimensionality reduction,Multivariate statistics
AI 理解论文
溯源树
样例
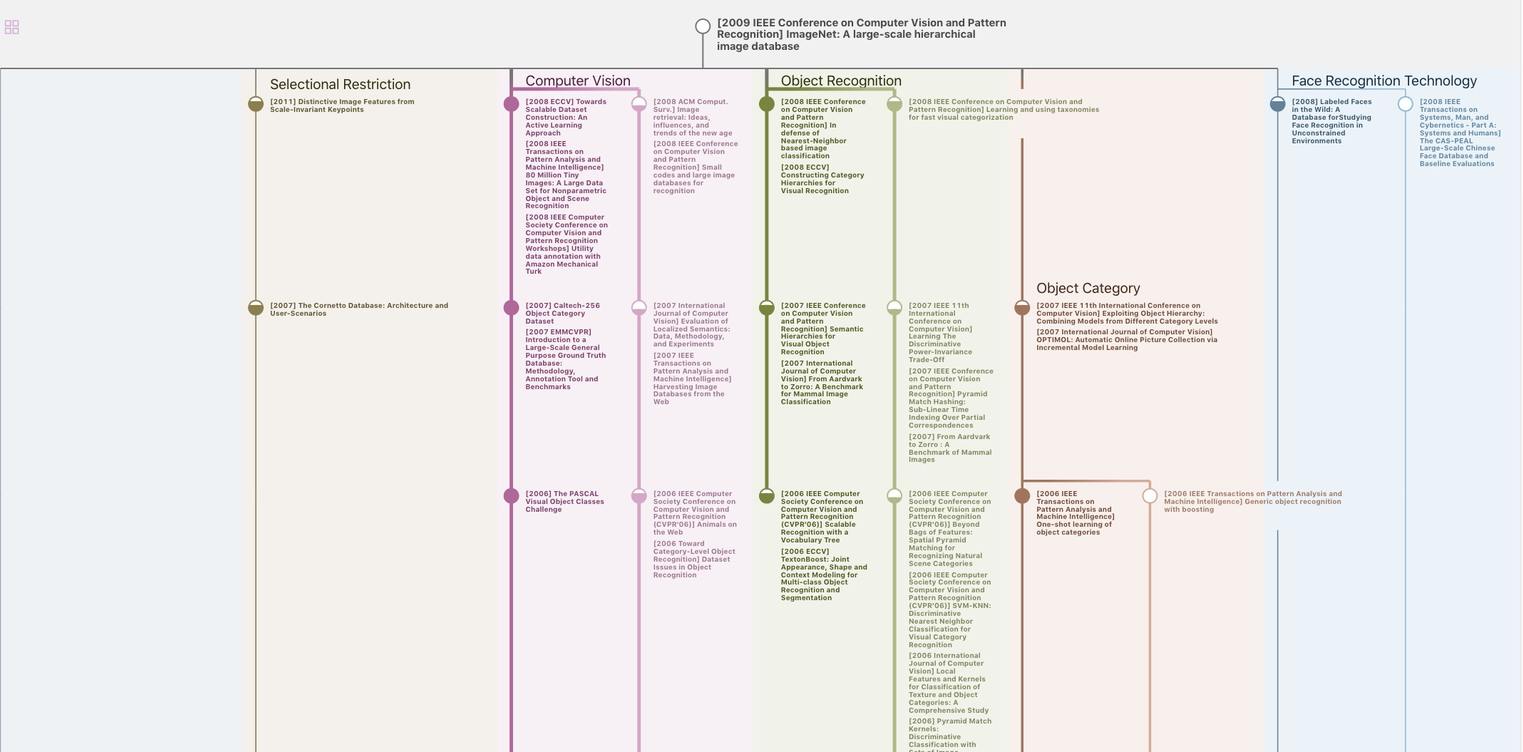
生成溯源树,研究论文发展脉络
Chat Paper
正在生成论文摘要