RQCSNet: A deep learning approach to quantized compressed sensing of remote sensing images
EXPERT SYSTEMS(2021)
摘要
In this article, a deep neural network is proposed that can be used to perform a quantized compressed sensing of remote sensing images. This network, dubbed RQCSNet consists of three parts: sensing, nonlinear quantization, and reconstruction. All network's parts are trained simultaneously according to the input data. RQCSNet has fully convolutional architecture, and it can work with different resolutions of the input images without the need for large initial fully connected layers. Furthermore, its structure reduces the blocky artefacts in the final reconstructed images by considering the effects of different image blocks in the reconstruction process. The proposed model has the ability to learn the nonlinear quantization function according to the input data and sending the measured values from the transmitter to the receiver with a fewer bit budget. Also, RQCSNet can learn the compressed sensing reconstruction algorithm without initial image reconstruction (that is usually the first step of reconstruction in other models), which reduces network flops. The results are presented on standard datasets of UCMerced as a remote sensing data that is the main concentration of this article, and as well as the set11 which is a dataset widely used for the benchmark of the state-of-the-art models. The experiments show that RQCSNet has a better performance than existing models in terms of the quality of image reconstruction and time complexity. As a benchmark, our network has a mean PSNR improvement of about 1.2 dB in reconstructed images compared to available techniques, at a measurement rate of 0.25. The effect of adding noise to the quantized measured data from the transmitter to the receiver at the time of transmission is also investigated in this article, and it is shown that the quality of image reconstruction will be robust to the noise once adding a noise layer to the network during training.
更多查看译文
关键词
deconvolutional layers, deep learning, quantized compressed sensing, remote sensing
AI 理解论文
溯源树
样例
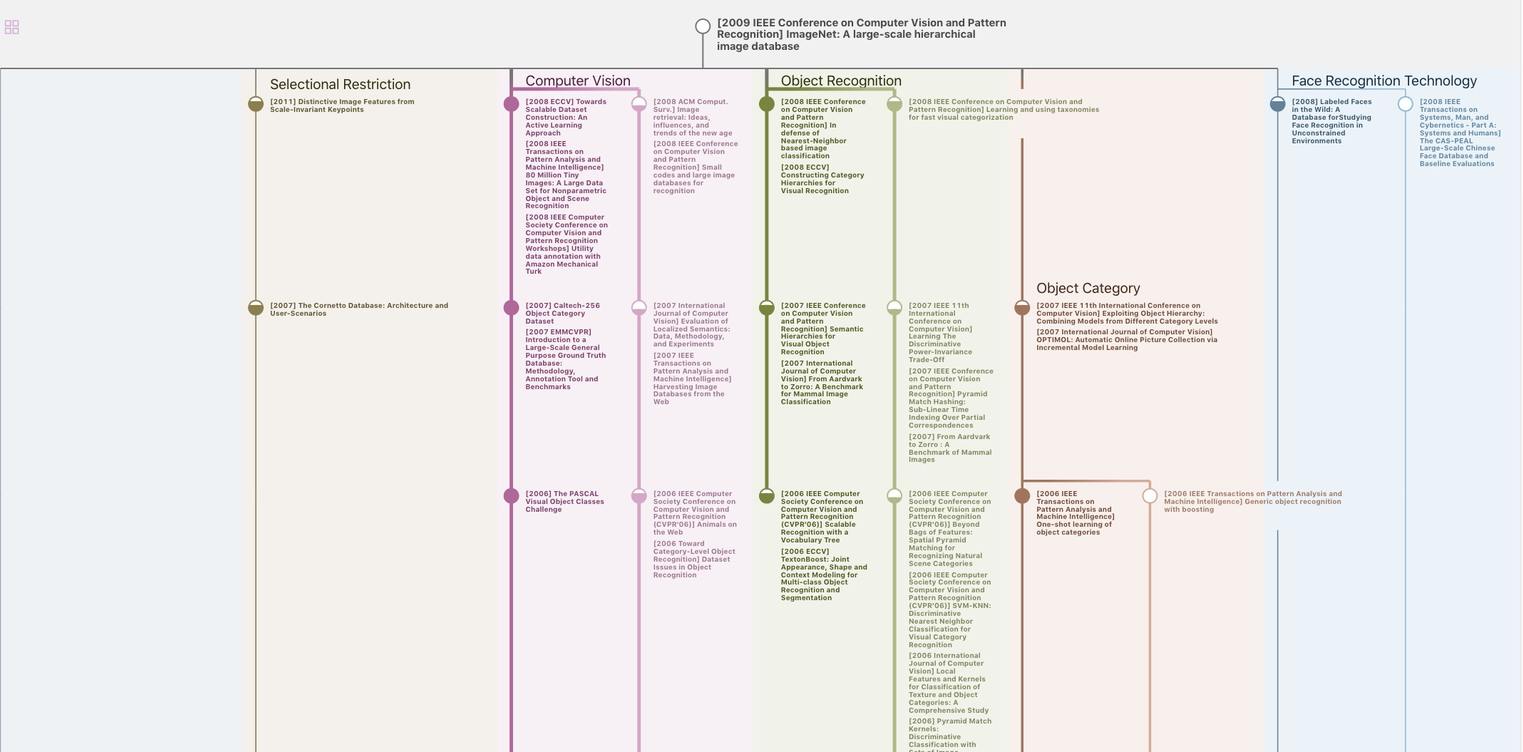
生成溯源树,研究论文发展脉络
Chat Paper
正在生成论文摘要