Improved finite-time solutions to time-varying Sylvester tensor equation via zeroing neural networks
APPLIED MATHEMATICS AND COMPUTATION(2022)
摘要
Based on the standard method of zeroing neural network (ZNN) for time-varying matrix problems, three improved ZNN models are proposed and extended to solve the time-varying Sylvester tensor equation (TV-STE) in finite-time. These presented ZNN models, which mainly use classical sign-bi-power (S-B-P) activation function and varying parameters, are S-B-P based ZNN (S-ZNN), S-B-P function based linear varying parameter ZNN (LVP-ZNN) and S-B-P function based exponential varying parameter ZNN (EVP-ZNN) models. Due to the applications of S-B-P activation function and varying parameters, the S-ZNN, LVP-ZNN and EVP-ZNN models can accomplish finite-time convergence, i.e., the state solutions generated by these models can converge to the analytical solutions of the TV-STE problem in finite-time. Further, based on the relationship between error, design parameter and convergence property, a dynamic varying parameter adapted to the error variation is designed and applied to the S-ZNN model. Then an error-adaptive dynamic varying parameter ZNN (DVP-ZNN) model is obtained for the TV-STE, which possesses faster finite-time convergence. These four models are proved to be stable and their upper bounds of convergence time are derived and analyzed. Theoretical analyses and comparison experiments demonstrate that the proposed ZNN models can solve the TV-STE in finite-time and the convergence of the DVP-ZNN model is the best. (C) 2021 Elsevier Inc. All rights reserved.
更多查看译文
关键词
Time-varying Sylvester tensor equation, Zeroing neural networks, Varying parameter, Finite-time convergence
AI 理解论文
溯源树
样例
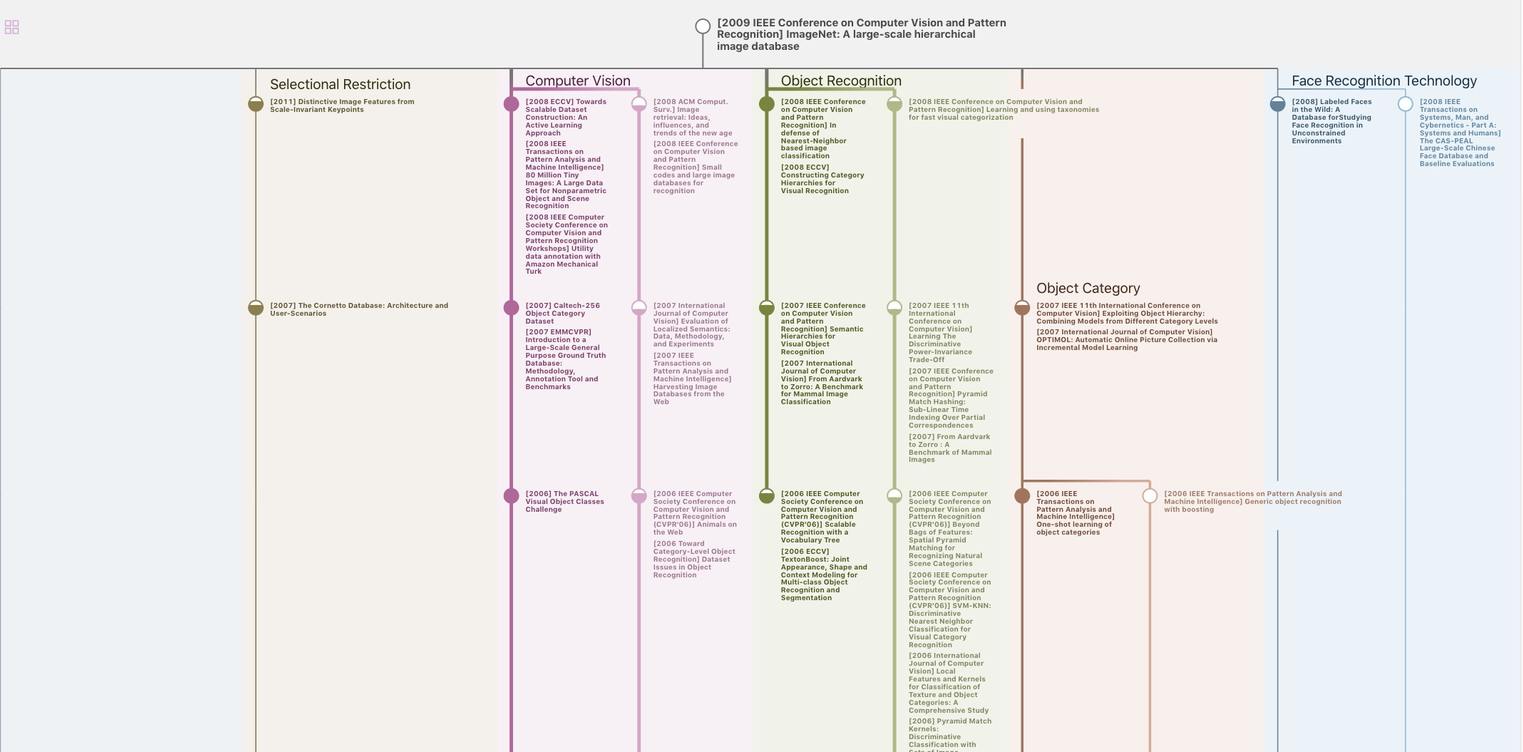
生成溯源树,研究论文发展脉络
Chat Paper
正在生成论文摘要