Combining multi-target regression deep neural networks and kinetic modeling to predict relative fluxes in reaction systems
Information and Computation(2021)
摘要
The strong nonlinearity of large and highly connected reaction systems, such as metabolic networks, hampers the determination of variations in reaction fluxes from variations in species abundances, when comparing different steady states of a given system. We hypothesize that patterns in species abundance variations exist that mainly depend on the kernel of the stoichiometric matrix and allow for predictions of flux variations. To investigate this hypothesis, we applied a multi-target regression Deep Neural Network (DNN) to data generated via numerical simulations of an Ordinary Differential Equation (ODE) model of yeast metabolism, upon Monte Carlo sampling of the kinetic parameters. For each parameter configuration, we compared two steady states corresponding to different environmental conditions. We show that DNNs can predict relative fluxes impressively well even when a random subspace of input features is supplied, supporting the existence of recurrent variation patterns in abundances of chemical species, which can be recognized automatically.
更多查看译文
关键词
ODE-based modelling,Monte Carlo sampling,Deep neural networks,Metabolomics,Metabolic fluxes
AI 理解论文
溯源树
样例
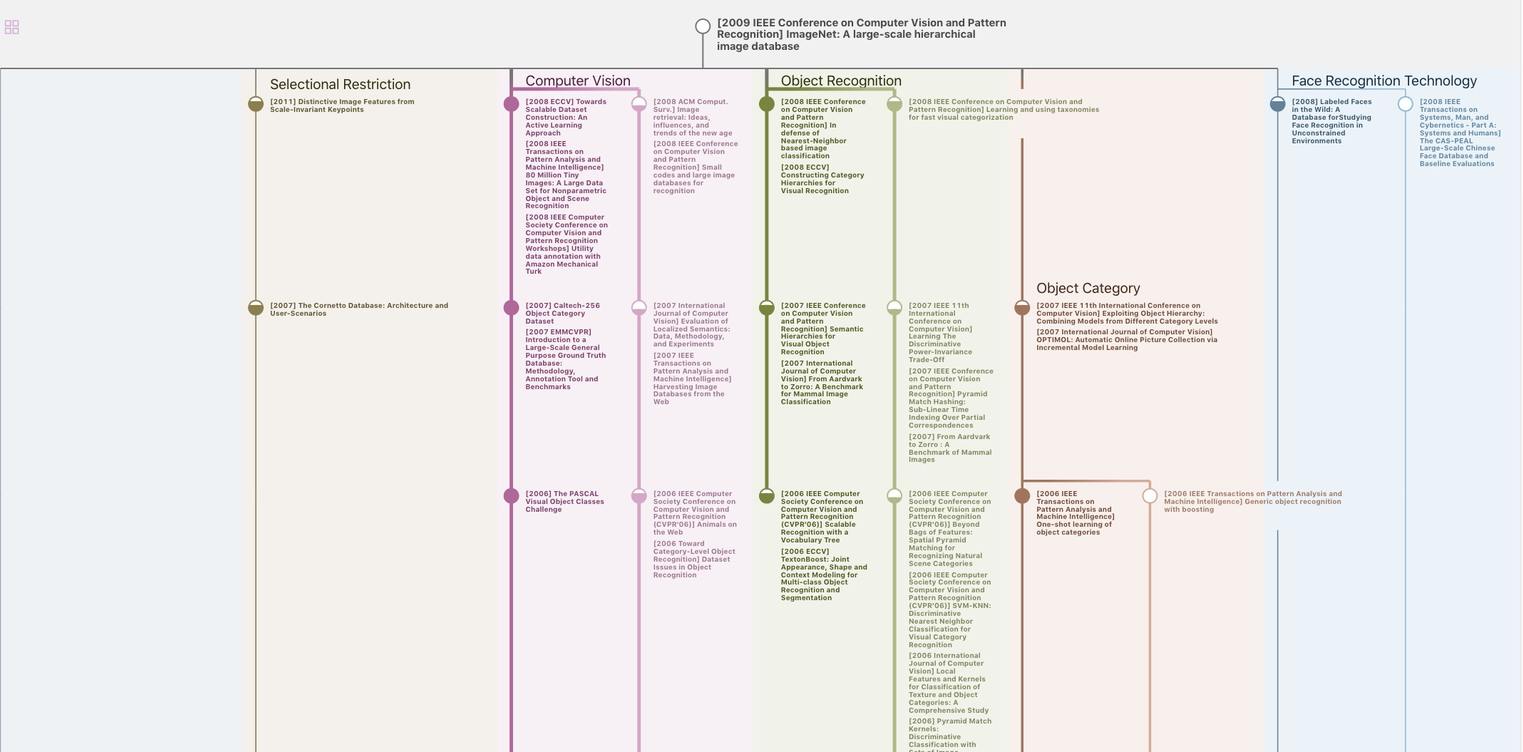
生成溯源树,研究论文发展脉络
Chat Paper
正在生成论文摘要