Global and local information aggregation network for edge-aware salient object detection
Journal of Visual Communication and Image Representation(2021)
摘要
Aggregation of local and global contextual information by exploiting multi-level features in a fully convolutional network is a challenge for the pixel-wise salient object detection task. Most existing methods still suffer from inaccurate salient regions and blurry boundaries. In this paper, we propose a novel edge-aware global and local information aggregation network (GLNet) to fully exploit the integration of side-output local features and global contextual information and utilization of contour information of salient objects. The global guidance module (GGM) is proposed to learn discriminative multi-level information with the direct guidance of global semantic knowledge for more accurate saliency prediction. Specifically, the GGM consists of two key components, where the global feature discrimination module exploits the inter-channel relationship of global semantic features to boost representation power, and the local feature discrimination module enables different side-output local features to selectively learn informative locations by fusing with global attentive features. Besides, we propose an edge-aware aggregation module (EAM) to employ the correlation between salient edge information and salient object information for generating estimated saliency maps with explicit boundaries. We evaluate our proposed GLNet on six widely-used saliency detection benchmark datasets by comparing with 17 state-of-the-art methods. Experimental results show the effectiveness and superiority of our proposed method on all the six benchmark datasets.
更多查看译文
关键词
Salient object detection,Saliency,Multi-level feature aggregation,Attention,Salient edge
AI 理解论文
溯源树
样例
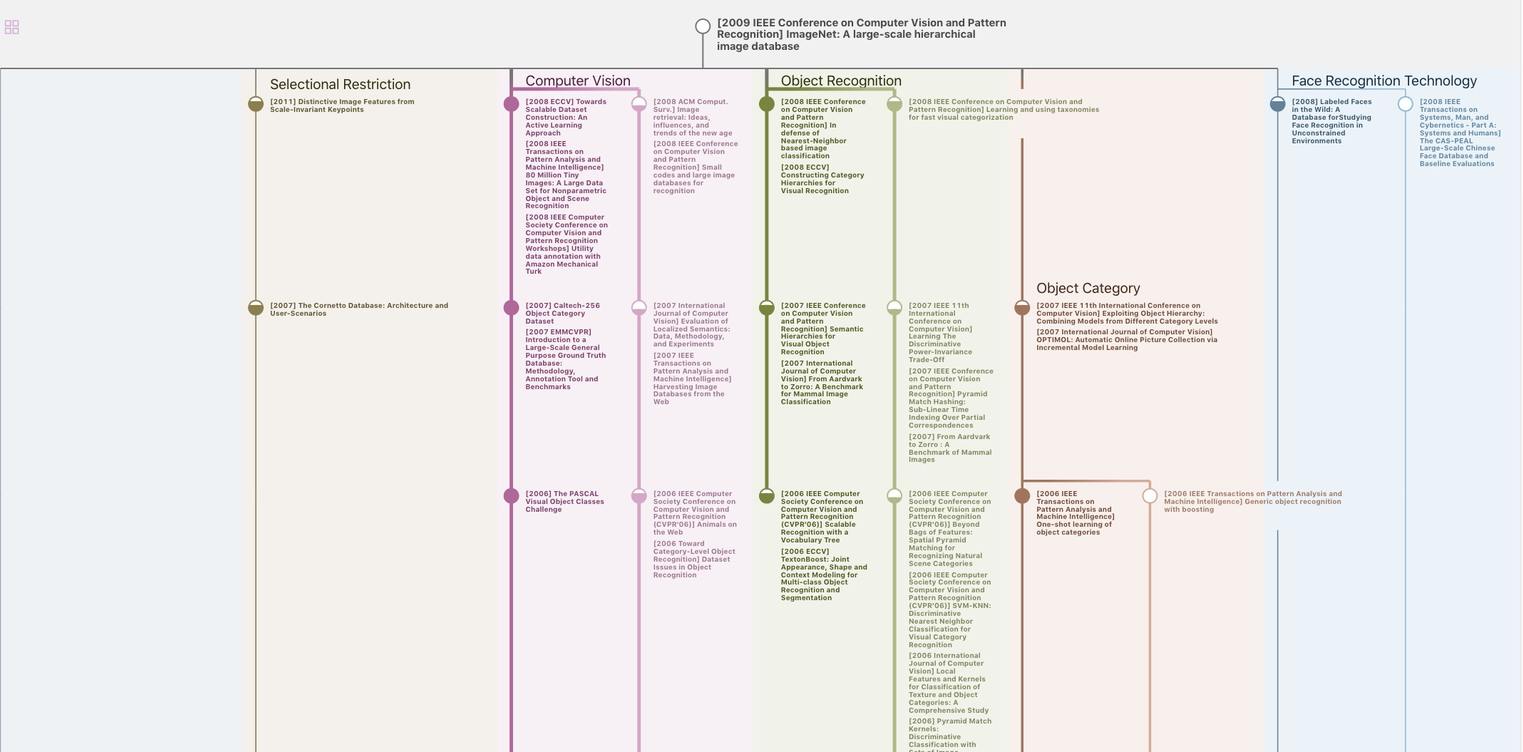
生成溯源树,研究论文发展脉络
Chat Paper
正在生成论文摘要