Deep node ranking for neuro-symbolic structural node embedding and classification
INTERNATIONAL JOURNAL OF INTELLIGENT SYSTEMS(2022)
摘要
Network node embedding is an active research subfield of complex network analysis. This paper contributes a novel approach to learning network node embeddings and direct node classification using a node ranking scheme, coupled with an autoencoder-based neural network architecture. The main advantages of the proposed Deep Node Ranking (DNR) algorithm are competitive or better classification performance, significantly higher learning speed and lower space requirements when compared to state-of-the-art approaches on 15 real-life structural node classification benchmarks. It also enables exploration of the relationship between symbolic and the derived sub-symbolic node representations, offering insights into the learned node space structure. To avoid the space complexity bottleneck in a direct node classification setting, DNR, if needed, computes stationary distributions of personalized random walks from given nodes in mini-batches, scaling seamlessly to larger networks. The scaling laws associated with DNR were also investigated by considering 1,488 synthetic Erdos-Renyi networks, demonstrating its scalability to tens of millions of links.
更多查看译文
关键词
complex networks, deep learning, network node embedding, node classification
AI 理解论文
溯源树
样例
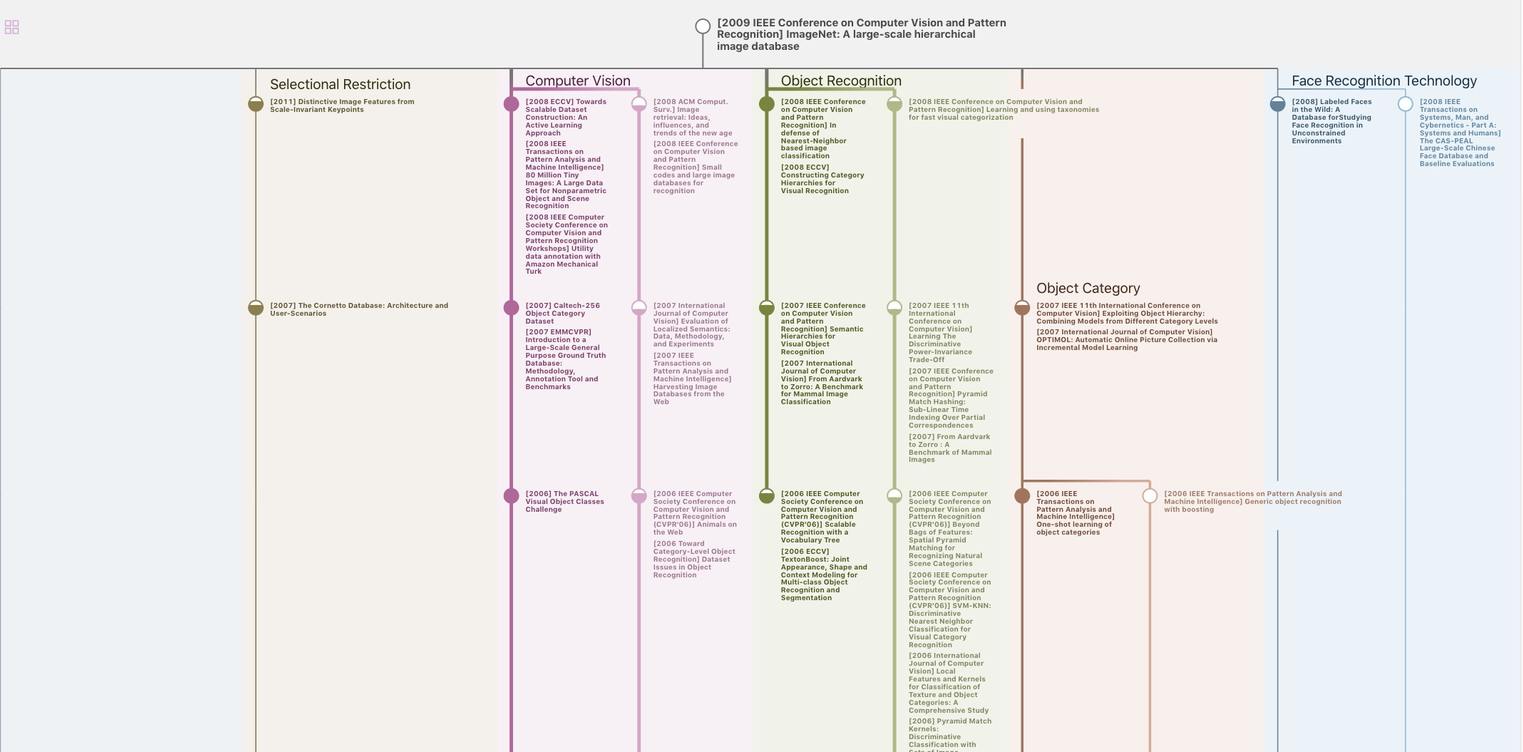
生成溯源树,研究论文发展脉络
Chat Paper
正在生成论文摘要