A Novel 2D-3D CNN with Spectral-Spatial Multi-Scale Feature Fusion for Hyperspectral Image Classification
REMOTE SENSING(2021)
摘要
Multifarious hyperspectral image (HSI) classification methods based on convolutional neural networks (CNN) have been gradually proposed and achieve a promising classification performance. However, hyperspectral image classification still suffers from various challenges, including abundant redundant information, insufficient spectral-spatial representation, irregular class distribution, and so forth. To address these issues, we propose a novel 2D-3D CNN with spectral-spatial multi-scale feature fusion for hyperspectral image classification, which consists of two feature extraction streams, a feature fusion module as well as a classification scheme. First, we employ two diverse backbone modules for feature representation, that is, the spectral feature and the spatial feature extraction streams. The former utilizes a hierarchical feature extraction module to capture multi-scale spectral features, while the latter extracts multi-stage spatial features by introducing a multi-level fusion structure. With these network units, the category attribute information of HSI can be fully excavated. Then, to output more complete and robust information for classification, a multi-scale spectral-spatial-semantic feature fusion module is presented based on a Decomposition-Reconstruction structure. Last of all, we innovate a classification scheme to lift the classification accuracy. Experimental results on three public datasets demonstrate that the proposed method outperforms the state-of-the-art methods.
更多查看译文
关键词
hyperspectral image classification, 2D-3D CNN, multi-scale features, multi-level features, attention module
AI 理解论文
溯源树
样例
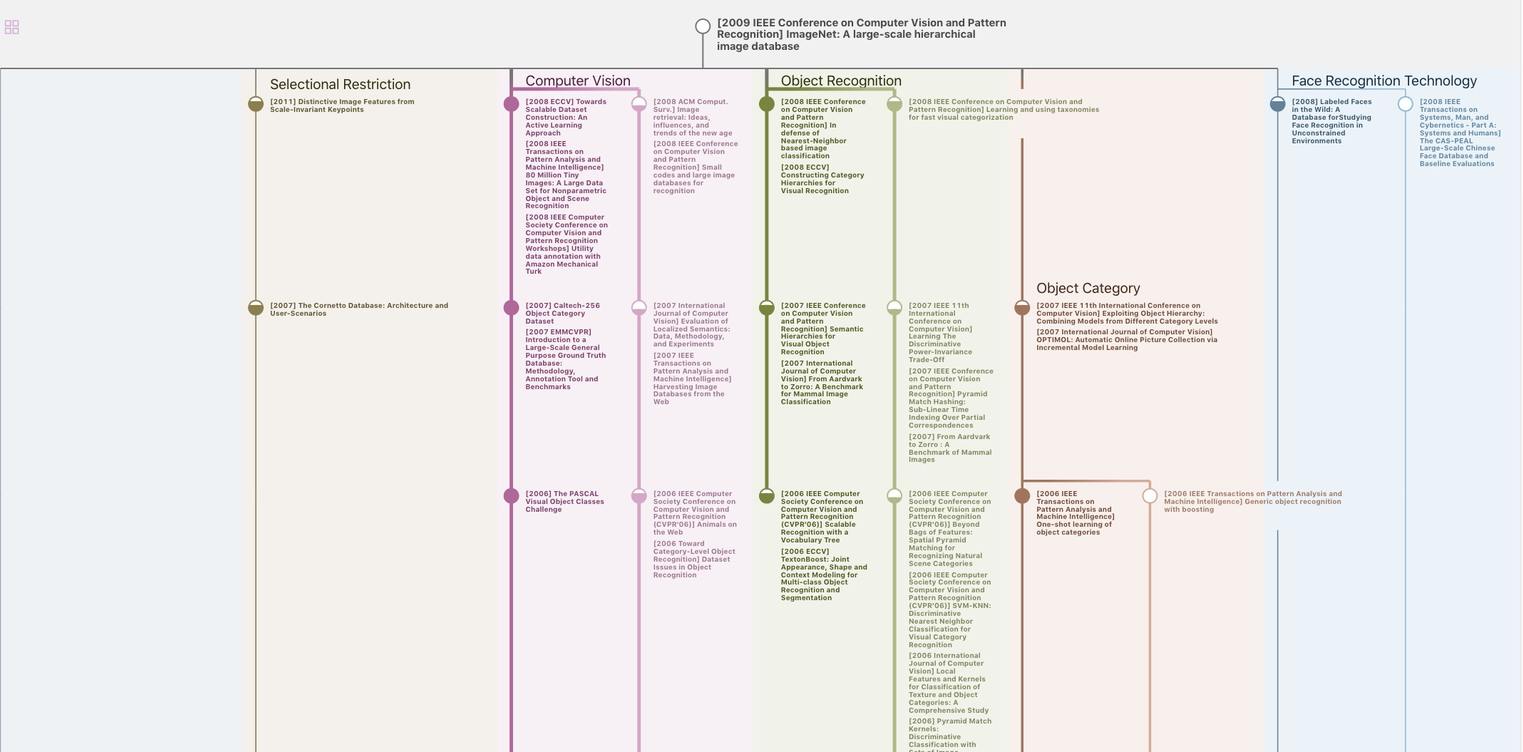
生成溯源树,研究论文发展脉络
Chat Paper
正在生成论文摘要