Subtidal Natural Hard Substrate Quantitative Habitat Mapping: Interlinking Underwater Acoustics and Optical Imagery with Machine Learning
REMOTE SENSING(2021)
摘要
Subtidal natural hard substrates (SNHS) promote occupancy by rich benthic communities that provide irreplaceable and fundamental ecosystem functions, representing a global priority target for nature conservation and recognised in most European environmental legislation. However, scientifically validated methodologies for their quantitative spatial demarcation, including information on species occupancy and fine-scale environmental drivers (e.g., the effect of stone size on colonisation) are rare. This is, however, crucial information for sound ecological management. In this investigation, high-resolution (1 m) multibeam echosounder (MBES) depth and backscatter data and derivates, underwater imagery (UI) by video drop-frame, and grab sediment samples, all acquired within 32 km(2) of seafloor in offshore Belgian waters, were integrated to produce a random forest (RF) spatial model, predicting the continuous distribution of the seafloor areal cover/m(2) of the stones' grain sizes promoting colonisation by sessile epilithic organisms. A semi-automated UI acquisition, processing, and analytical workflow was set up to quantitatively study the colonisation proportion of different grain sizes, identifying the colonisation potential to begin at stones with grain sizes o & GE; 2 cm. This parameter (i.e., % areal cover of stones o & GE; 2 cm/m(2)) was selected as the response variable for spatial predictive modelling. The model output is presented along with a protocol of error and uncertainty estimation. RF is confirmed as an accurate, versatile, and transferable mapping methodology, applicable to area-wide mapping of SNHS. UI is confirmed as an essential aid to acoustic seafloor classification, providing spatially representative numerical observations needed to carry out quantitative seafloor modelling of ecologically relevant parameters. This contribution sheds innovative insights into the ecologically relevant delineation of subtidal natural reef habitat, exploiting state-of-the-art underwater remote sensing and acoustic seafloor classification approaches.
更多查看译文
关键词
underwater imagery, multibeam echosounder, random forest, subtidal natural hard substrate, stone colonisation, epilithic fauna
AI 理解论文
溯源树
样例
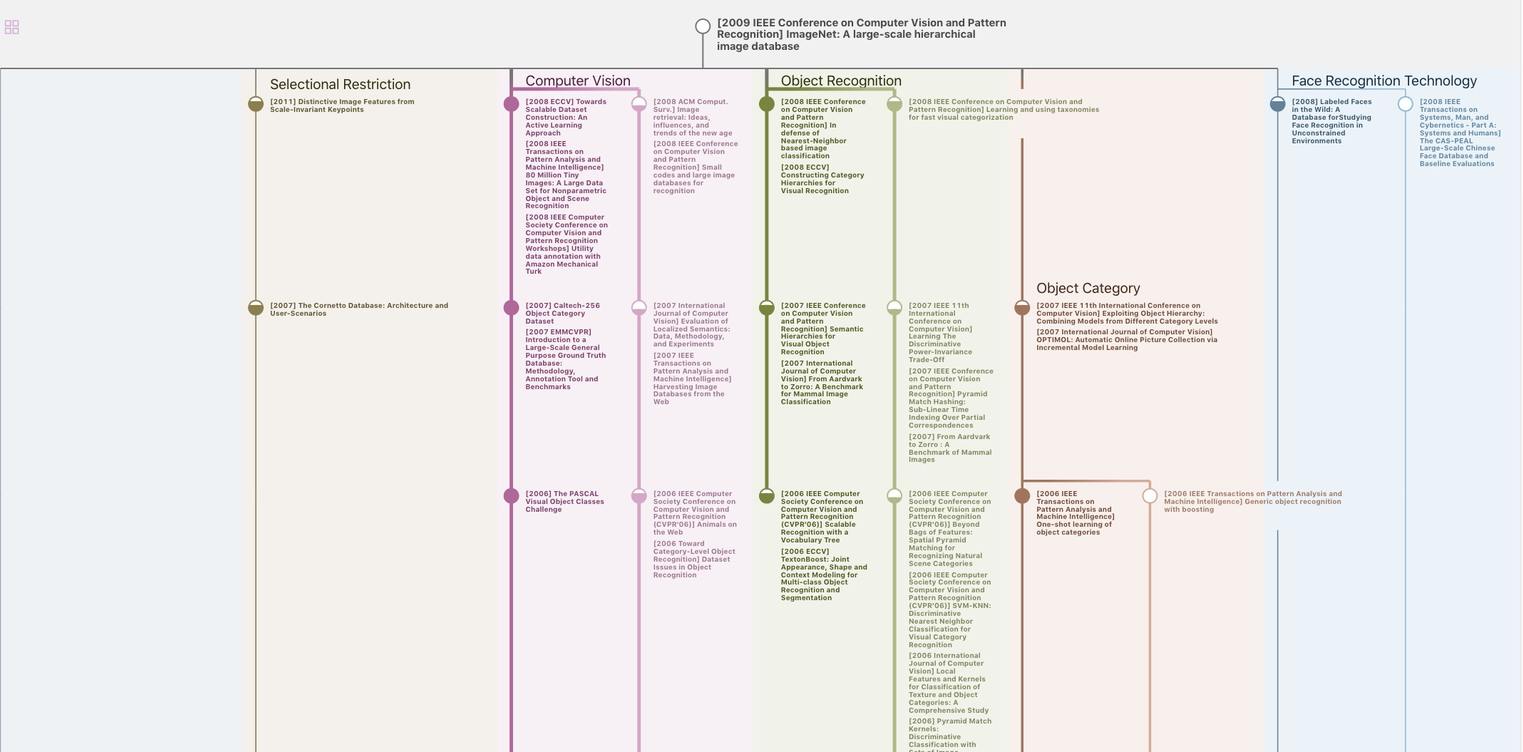
生成溯源树,研究论文发展脉络
Chat Paper
正在生成论文摘要