Integrating Multiple Datasets and Machine Learning Algorithms for Satellite-Based Bathymetry in Seaports
REMOTE SENSING(2021)
摘要
Water depth estimation in seaports is essential for effective port management. This paper presents an empirical approach for water depth determination from satellite imagery through the integration of multiple datasets and machine learning algorithms. The implementation details of the proposed approach are provided and compared against different existing machine learning algorithms with a single training set. For a single training set and a single machine learning method, our analysis shows that the proposed depth estimation method provides a better root-mean-square error (RMSE) and a higher coefficient of determination (R-2) under turbid water conditions, with overall RMSE and R-2 improvements of 1 cm and 0.7, respectively. The developed method may be employed in monitoring dredging activities, especially in areas with polluted water, mud and/or a high sediment content.
更多查看译文
关键词
ensemble learning, classifier fusion, support vector machine, random forest, multi-adaptive regression spline, neural networks, port, bathymetry
AI 理解论文
溯源树
样例
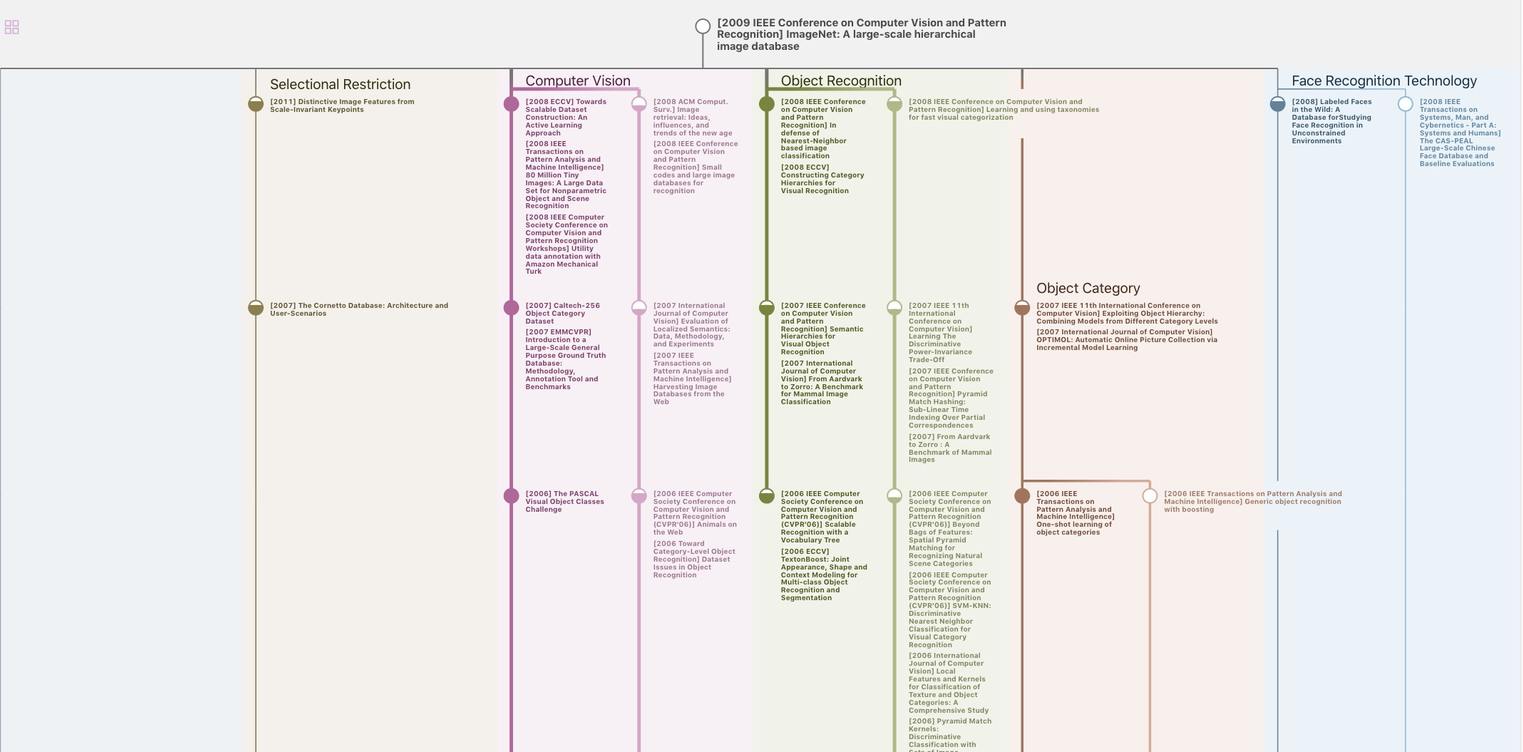
生成溯源树,研究论文发展脉络
Chat Paper
正在生成论文摘要