Session introduction: AI-driven Advances in Modeling of Protein Structure.
Pacific Symposium on Biocomputing. Pacific Symposium on Biocomputing(2022)
摘要
The last few years mark dramatic improvements in modeling of protein structure. Progress was initially due to breakthroughs in residue-residue contact prediction, first with global statistical models and later with deep learning. These advancements were then followed by an even broader application of the deep learning techniques to the protein structure modeling itself, first using Convolutional Neural Networks (CNNs) and then switching to Natural Language Processing (NLP), including Attention models, and to Geometric Deep Learning (GDL). The accuracy of protein structure models generated with current state-of-the-art methods rivals that of experimental structures, while models themselves are used to solve structures or to make them more accurate.Looking at the near future of machine learning applications in structural biology, we ask the following questions: Which specific problems should we expect to be solved next? Which new methods will prove to be the most effective? Which actions are likely to stimulate further progress the most? In addressing these questions, we invite the 2022 PSB attendees to actively participate in session discussions.The AI-driven Advances in Modeling of Protein Structure session includes five papers specifically dedicated to:Evaluating the significance of training data selection in machine learning.Geometric pattern transferability, from protein self-interactions to protein-ligand interactions.Supervised versus unsupervised sequence to contact learning, using attention models.Side chain packing using SE(3) transformers.Feature detection in electrostatic representations of ligand binding sites.
更多查看译文
关键词
protein structure,modeling,ai-driven
AI 理解论文
溯源树
样例
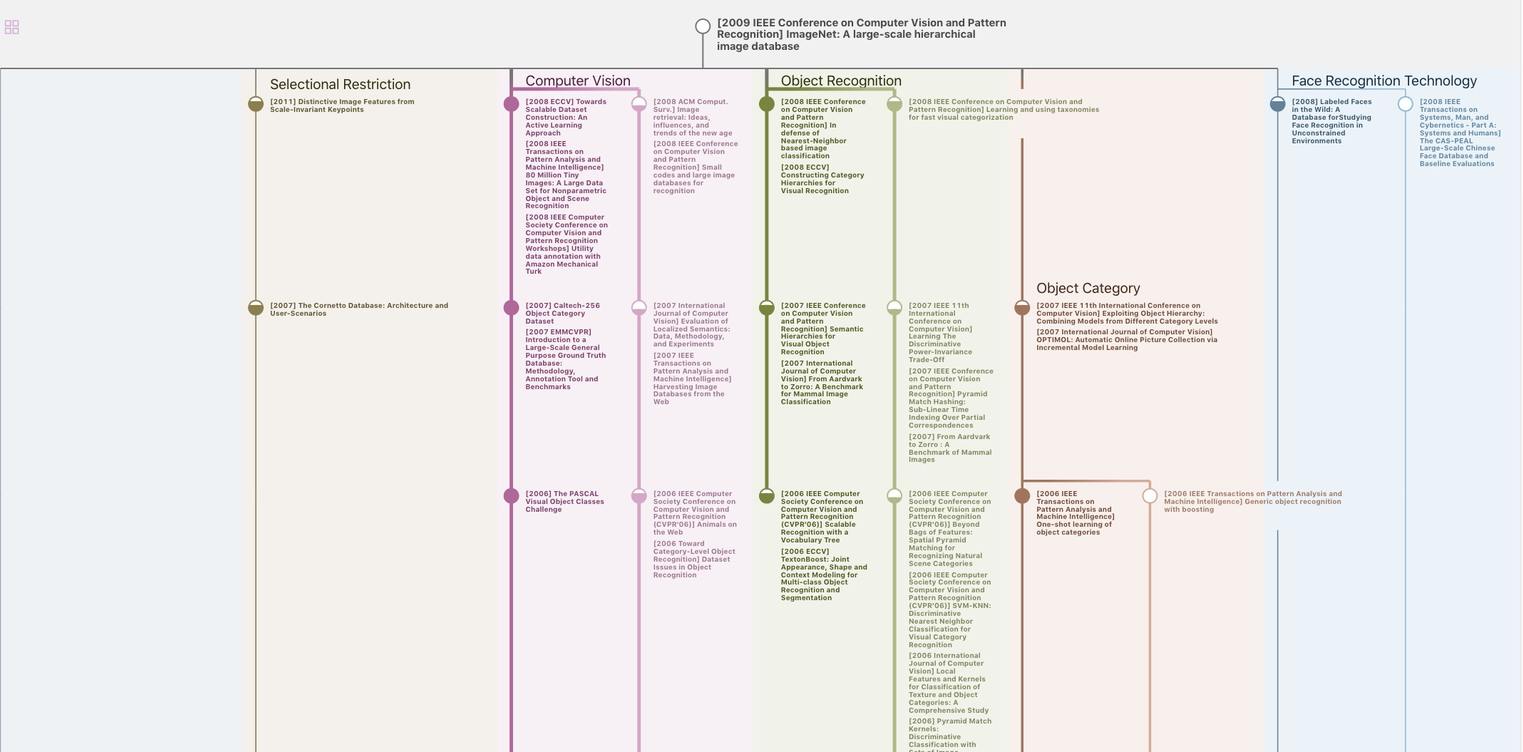
生成溯源树,研究论文发展脉络
Chat Paper
正在生成论文摘要