Hybrid interpretable predictive machine learning model for air pollution prediction
Neurocomputing(2022)
摘要
Air pollution prediction is a burning issue, as pollutants can harm human health. Traditional machine learning models usually aim to improve the overall prediction accuracy but neglect the accuracy for peak values. Moreover, these models are not interpretable. They fail to explain the interactions between various determining factors and their impacts on air pollution. In this paper, we propose a new Hybrid Interpretable Predictive Machine Learning model for the Particulate Matter 2.5 prediction, which carries two novelties. First, a hybrid model structure is constructed with deep neural network and Nonlinear Auto Regressive Moving Average with Exogenous Input model. Second, automatic feature generation and feature selection procedures are integrated into this hybrid model. The experimental results demonstrate the superiority of our model over other models in prediction accuracy for peak values and model interpretability. The proposed model reveals how PM2.5 prediction is estimated by historical PM2.5, weather, and season. The accuracies (measured by correlation coefficients) of 1, 3 and 6-hour-ahead prediction are 0.9870, 0.9332 and 0.8587, respectively. More importantly, the proposed approach presents a new interpretable machine learning framework for time series data, enabling to explain complex dependence of multimode inputs, and to build reliable predictive models.
更多查看译文
关键词
Air pollution prediction,Interpretable machine learning,Neural network,NARMAX model
AI 理解论文
溯源树
样例
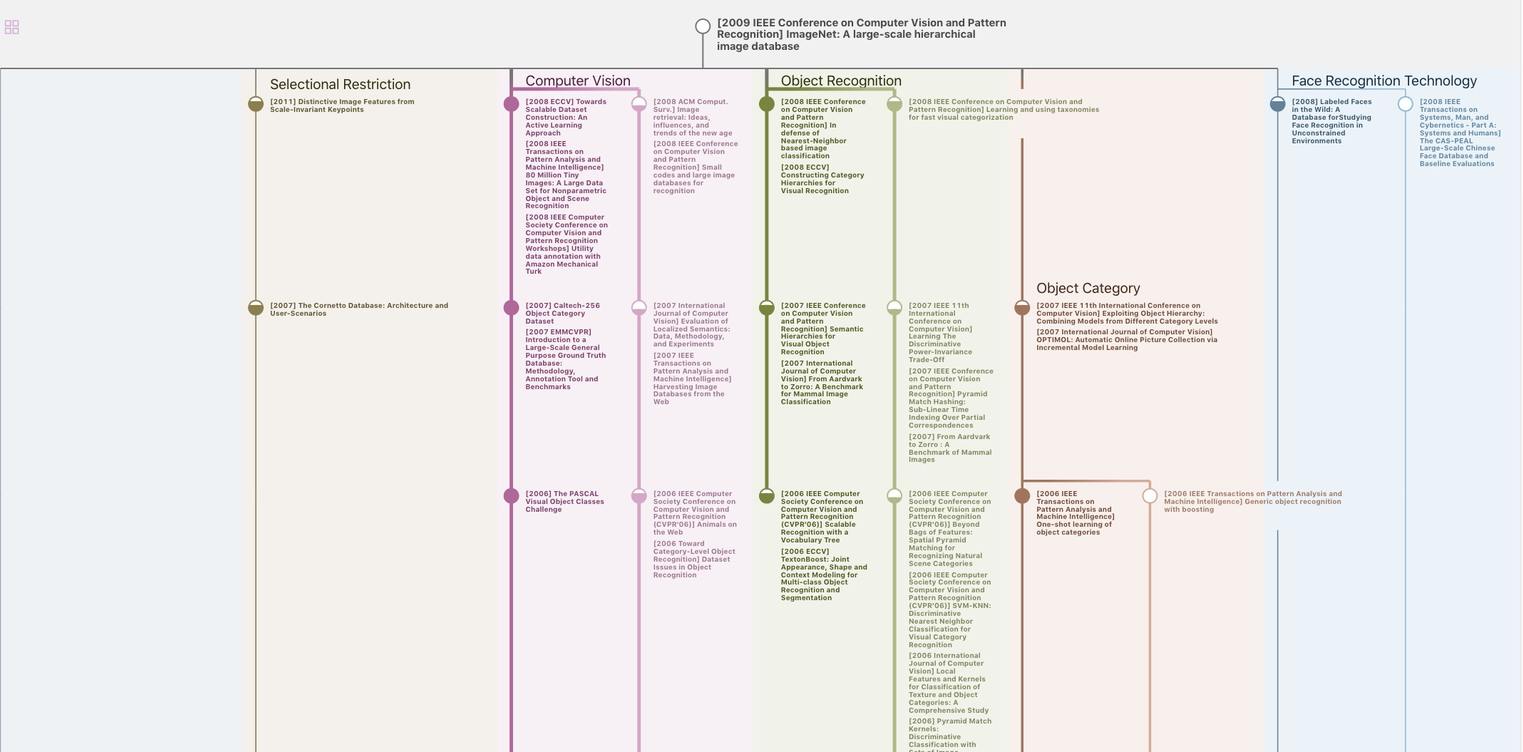
生成溯源树,研究论文发展脉络
Chat Paper
正在生成论文摘要