Deep Learning Approaches and Applications in Toxicologic Histopathology: Current Status and Future Perspectives.
Journal of pathology informatics(2021)
摘要
Whole slide imaging enables the use of a wide array of digital image analysis tools that are revolutionizing pathology. Recent advances in digital pathology and deep convolutional neural networks have created an enormous opportunity to improve workflow efficiency, provide more quantitative, objective, and consistent assessments of pathology datasets, and develop decision support systems. Such innovations are already making their way into clinical practice. However, the progress of machine learning - in particular, deep learning (DL) - has been rather slower in nonclinical toxicology studies. Histopathology data from toxicology studies are critical during the drug development process that is required by regulatory bodies to assess drug-related toxicity in laboratory animals and its impact on human safety in clinical trials. Due to the high volume of slides routinely evaluated, low-throughput, or narrowly performing DL methods that may work well in small-scale diagnostic studies or for the identification of a single abnormality are tedious and impractical for toxicologic pathology. Furthermore, regulatory requirements around good laboratory practice are a major hurdle for the adoption of DL in toxicologic pathology. This paper reviews the major DL concepts, emerging applications, and examples of DL in toxicologic pathology image analysis. We end with a discussion of specific challenges and directions for future research.
更多查看译文
关键词
Deep learning,digital image analysis,histopathology,machine learning,preclinical safety,toxicologic pathology,whole slide imaging
AI 理解论文
溯源树
样例
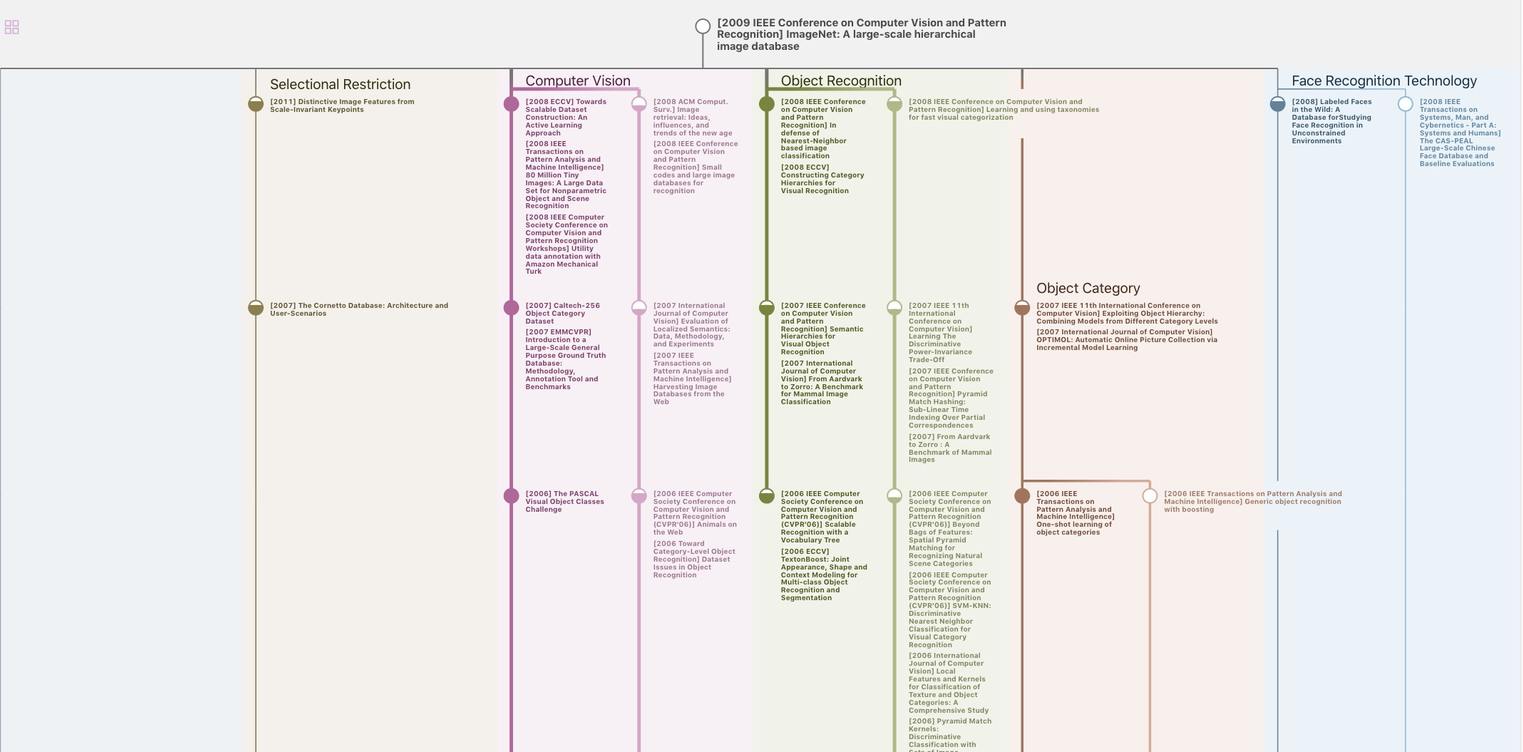
生成溯源树,研究论文发展脉络
Chat Paper
正在生成论文摘要