Rethinking the Authorship Verification Experimental Setups
arxiv(2022)
摘要
One of the main drivers of the recent advances in authorship verification is the PAN large-scale authorship dataset. Despite generating significant progress in the field, inconsistent performance differences between the closed and open test sets have been reported. To this end, we improve the experimental setup by proposing five new public splits over the PAN dataset, specifically designed to isolate and identify biases related to the text topic and to the author's writing style. We evaluate several BERT-like baselines on these splits, showing that such models are competitive with authorship verification state-of-the-art methods. Furthermore, using explainable AI, we find that these baselines are biased towards named entities. We show that models trained without the named entities obtain better results and generalize better when tested on DarkReddit, our new dataset for authorship verification.
更多查看译文
关键词
authorship verification experimental setups
AI 理解论文
溯源树
样例
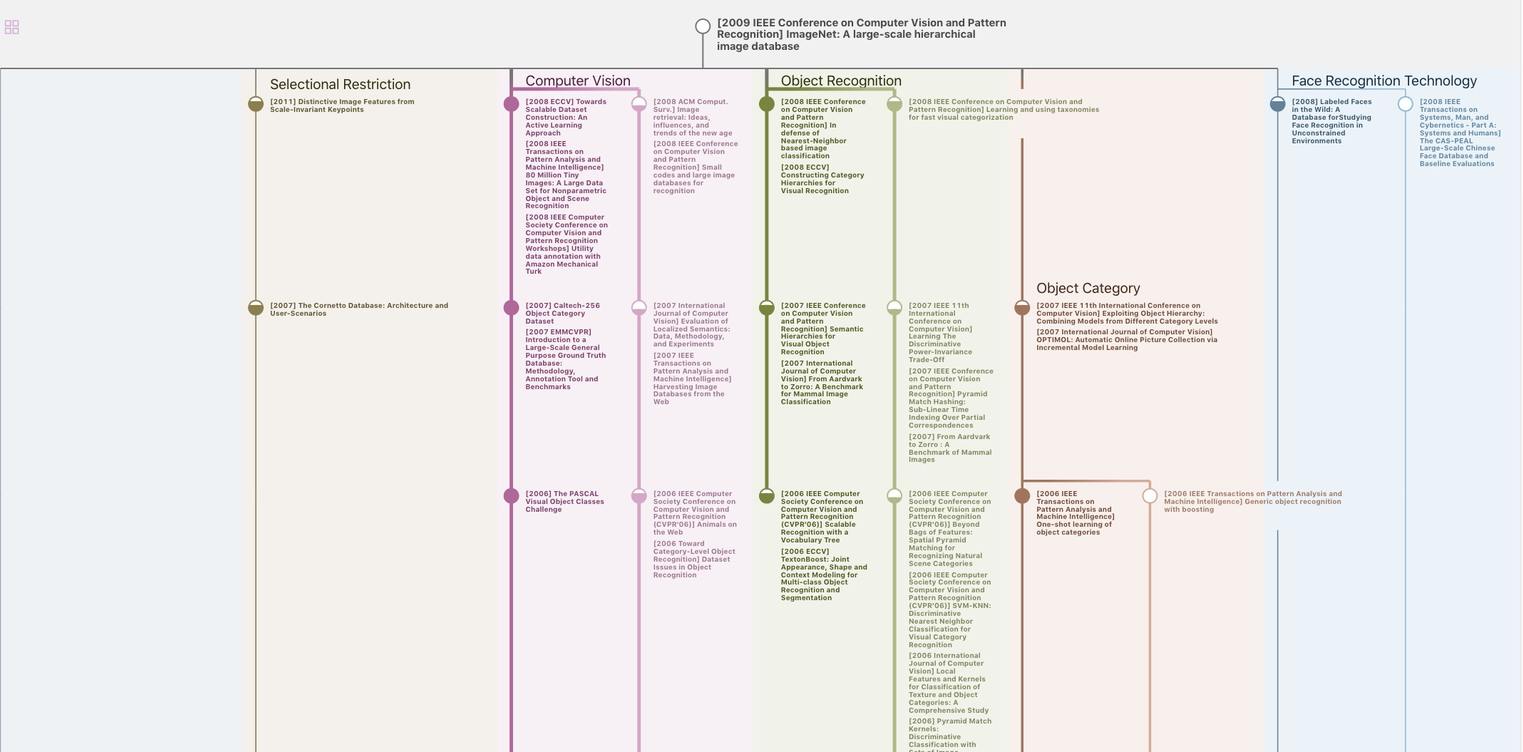
生成溯源树,研究论文发展脉络
Chat Paper
正在生成论文摘要