Gaussian Process Constraint Learning for Scalable Chance-Constrained Motion Planning From Demonstrations
IEEE ROBOTICS AND AUTOMATION LETTERS(2022)
摘要
We propose a method for learning constraints represented as Gaussian processes (GPs) from locally-optimal demonstrations. Our approach uses the Karush-Kuhn-Tucker (KKT) optimality conditions to determine where on the demonstrations the constraint is tight, and a scaling of the constraint gradient at those states. We then train a GP representation of the constraint which is consistent with and which generalizes this information. We further show that the GP uncertainty can be used within a kinodynamic RRT to plan probabilistically-safe trajectories, and that we can exploit the GP structure within the planner to exactly achieve a specified safety probability. We demonstrate our method can learn complex, nonlinear constraints demonstrated on a 5D nonholonomic car, a 12D quadrotor, and a 3-link planar arm, all while requiring minimal prior information on the constraint. Our results suggest the learned GP constraint is accurate, outperforming previous constraint learning methods that require more a priori knowledge.
更多查看译文
关键词
Task analysis, Uncertainty, Trajectory, Planning, Safety, Gaussian processes, Cost function, Learning from demonstration, planning under uncertainty, machine learning for robot control, robot safety
AI 理解论文
溯源树
样例
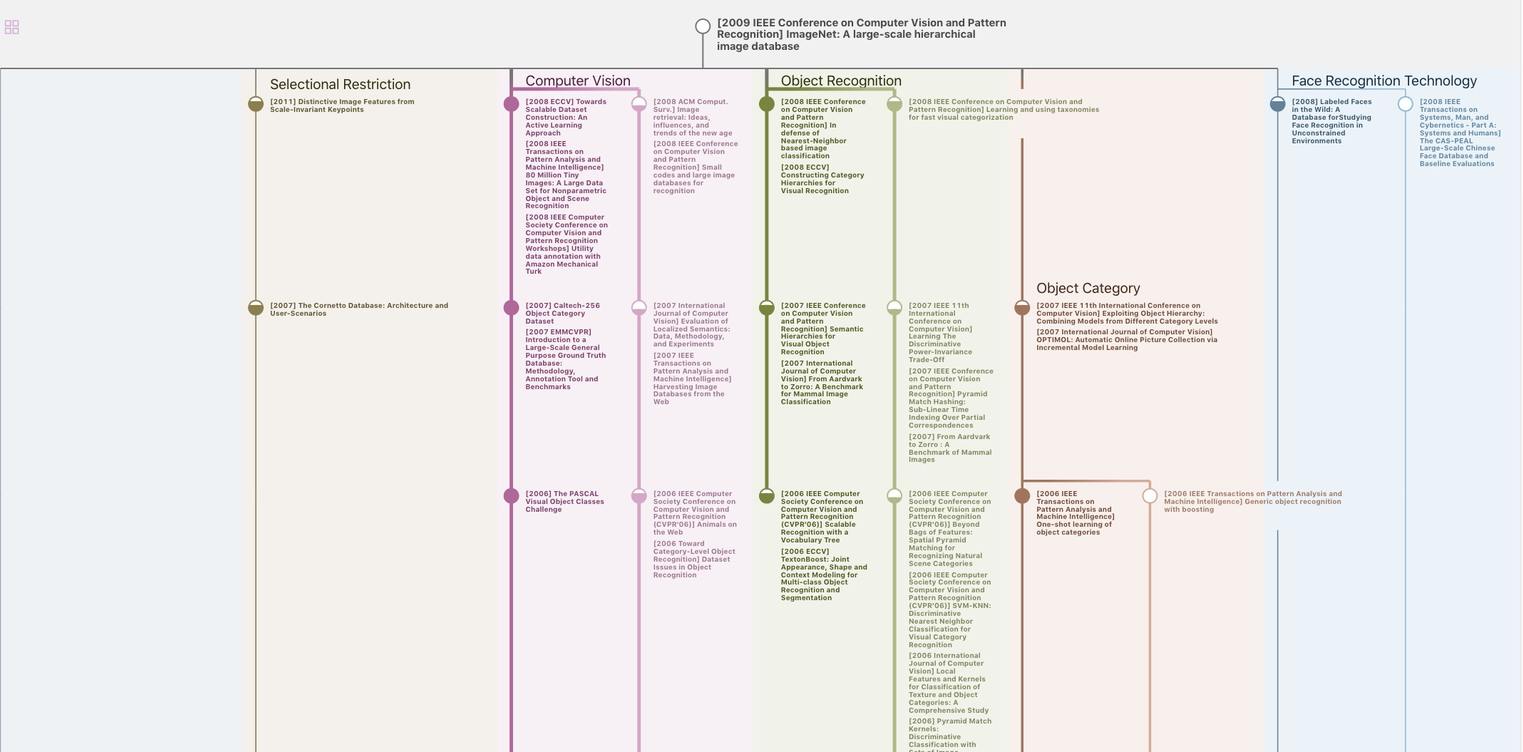
生成溯源树,研究论文发展脉络
Chat Paper
正在生成论文摘要