The Perils of Being Unhinged: On the Accuracy of Classifiers Minimizing a Noise-Robust Convex Loss
Neural Computation(2022)
摘要
van Rooyen, Menon, and Williamson (2015) introduced a notion of convex loss functions being robust to random classification noise and established that the “unhinged” loss function is robust in this sense. In this letter, we study the accuracy of binary classifiers obtained by minimizing the unhinged loss and observe that even for simple linearly separable data distributions, minimizing the unhinged loss may only yield a binary classifier with accuracy no better than random guessing.
更多查看译文
关键词
classifiers,accuracy,loss,noise-robust
AI 理解论文
溯源树
样例
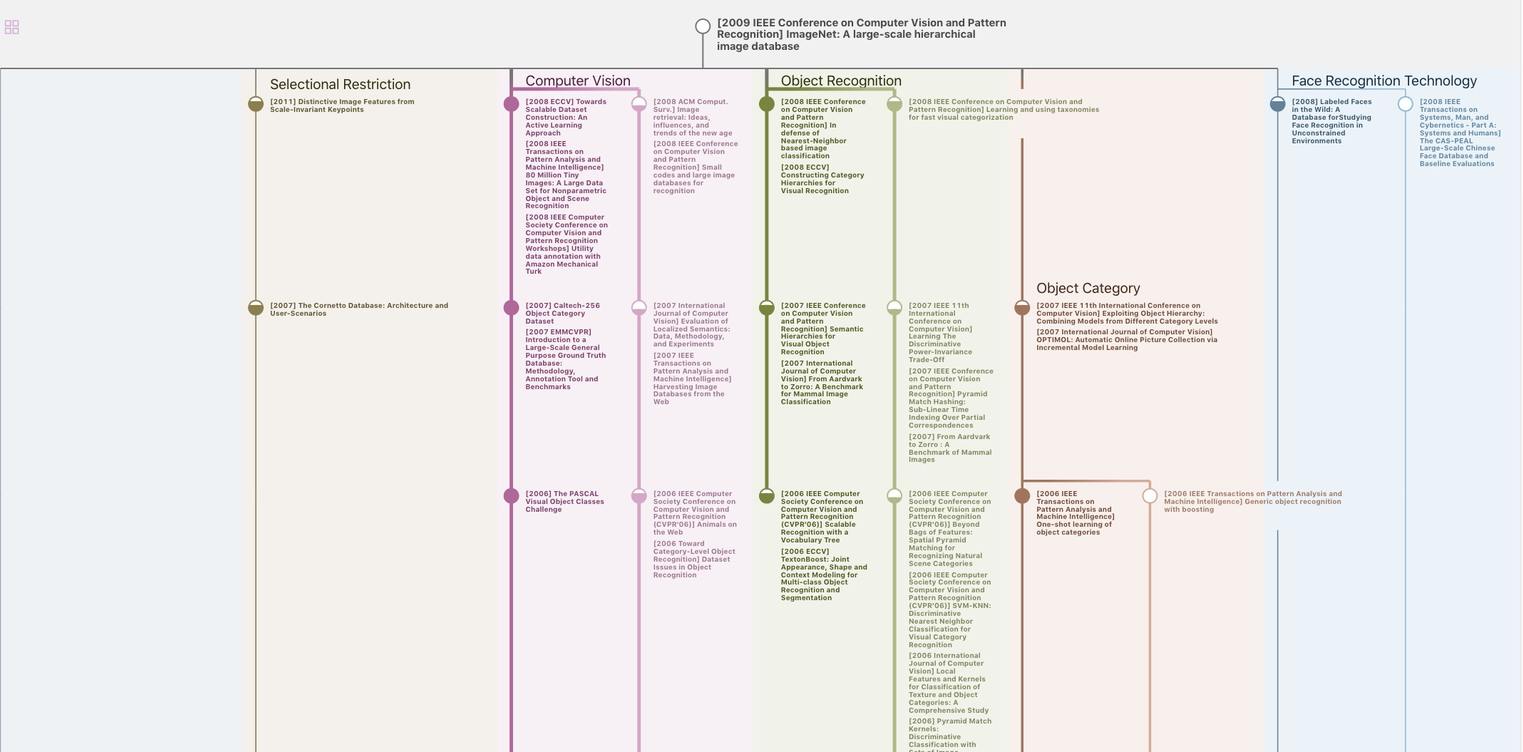
生成溯源树,研究论文发展脉络
Chat Paper
正在生成论文摘要