Towards deep entity resolution via soft schema matching
Neurocomputing(2022)
摘要
Entity resolution (ER) leads a key role in data preprocessing. ER identifies records corresponding to the same real-world entity. Recent years have witnessed a growing trend of deep learning based ER (deep ER). However, previous deep ER works do not fully utilize schema semantics, since they either use hard schema matching or disregard schema matching. In this work, we flexibly exploit schema matching to enhance deep ER. We define and implement soft schema matching, where attributes are flexibly associated in probabilities. Attribute associations are generated by aggregating token connections in coarse deep ER. Then we incorporate soft schema matching into hierarchical attention networks for ER, which tremendously improves resolution quality, especially for complex data and corrupted data. Different attentions are utilized for particular sub-tasks in ER networks, such as self-attention for contextualization, inter-attention for alignment and intra-attention for weighting. Finally comprehensive experiments are run over common data, complex data and corrupted data. Evaluation results show that our approach surpasses previous works.
更多查看译文
关键词
Entity resolution,Soft schema matching,Deep learning,Attention network,Data preprocessing
AI 理解论文
溯源树
样例
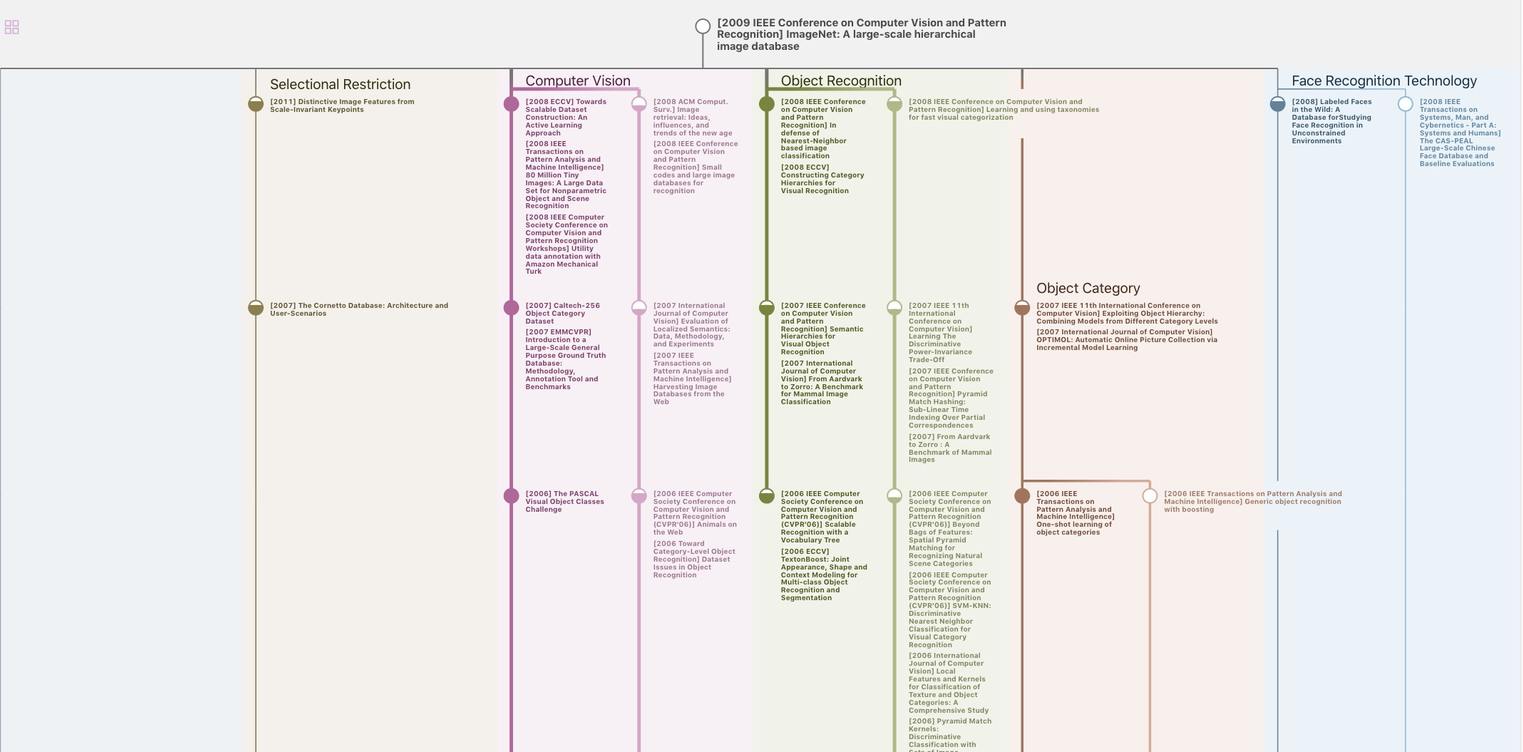
生成溯源树,研究论文发展脉络
Chat Paper
正在生成论文摘要