Revisiting Contrastive Learning through the Lens of Neighborhood Component Analysis: an Integrated Framework
International Conference on Machine Learning(2022)
摘要
As a seminal tool in self-supervised representation learning, contrastive learning has gained unprecedented attention in recent years. In essence, contrastive learning aims to leverage pairs of positive and negative samples for representation learning, which relates to exploiting neighborhood information in a feature space. By investigating the connection between contrastive learning and neighborhood component analysis (NCA), we provide a novel stochastic nearest neighbor viewpoint of contrastive learning and subsequently propose a series of contrastive losses that outperform the existing ones. Under our proposed framework, we show a new methodology to design integrated contrastive losses that could simultaneously achieve good accuracy and robustness on downstream tasks. With the integrated framework, we achieve up to 6% improvement on the standard accuracy and 17% improvement on the robust accuracy.
更多查看译文
关键词
contrastive learning,component analysis,neighborhood
AI 理解论文
溯源树
样例
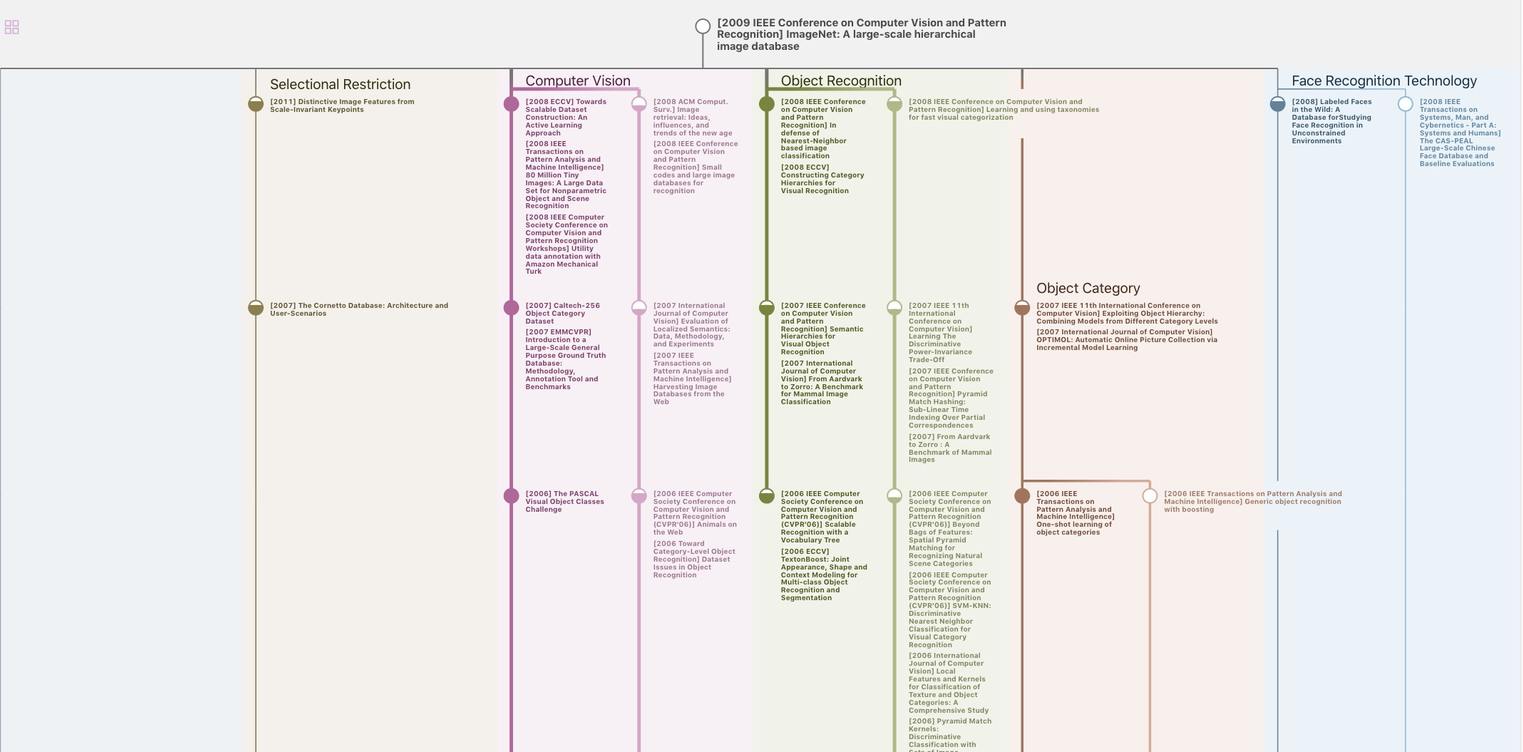
生成溯源树,研究论文发展脉络
Chat Paper
正在生成论文摘要