Generalization error bounds for iterative recovery algorithms unfolded as neural networks
arxiv(2023)
摘要
Motivated by the learned iterative soft thresholding algorithm (LISTA), we introduce a general class of neural networks suitable for sparse reconstruction from few linear measurements. By allowing a wide range of degrees of weight-sharing between the flayers, we enable a unified analysis for very different neural network types, ranging from recurrent ones to networks more similar to standard feedforward neural networks. Based on training samples, via empirical risk minimization, we aim at learning the optimal network parameters and thereby the optimal network that reconstructs signals from their low-dimensional linear measurements. We derive generalization bounds by analyzing the Rademacher complexity of hypothesis classes consisting of such deep networks, that also take into account the thresholding parameters. We obtain estimates of the sample complexity that essentially depend only linearly on the number of parameters and on the depth. We apply our main result to obtain specific generalization bounds for several practical examples, including different algorithms for (implicit) dictionary learning, and convolutional neural networks.
更多查看译文
关键词
compressive sensing, neural networks, iterative soft thresholding, generalization, Rademacher complexity
AI 理解论文
溯源树
样例
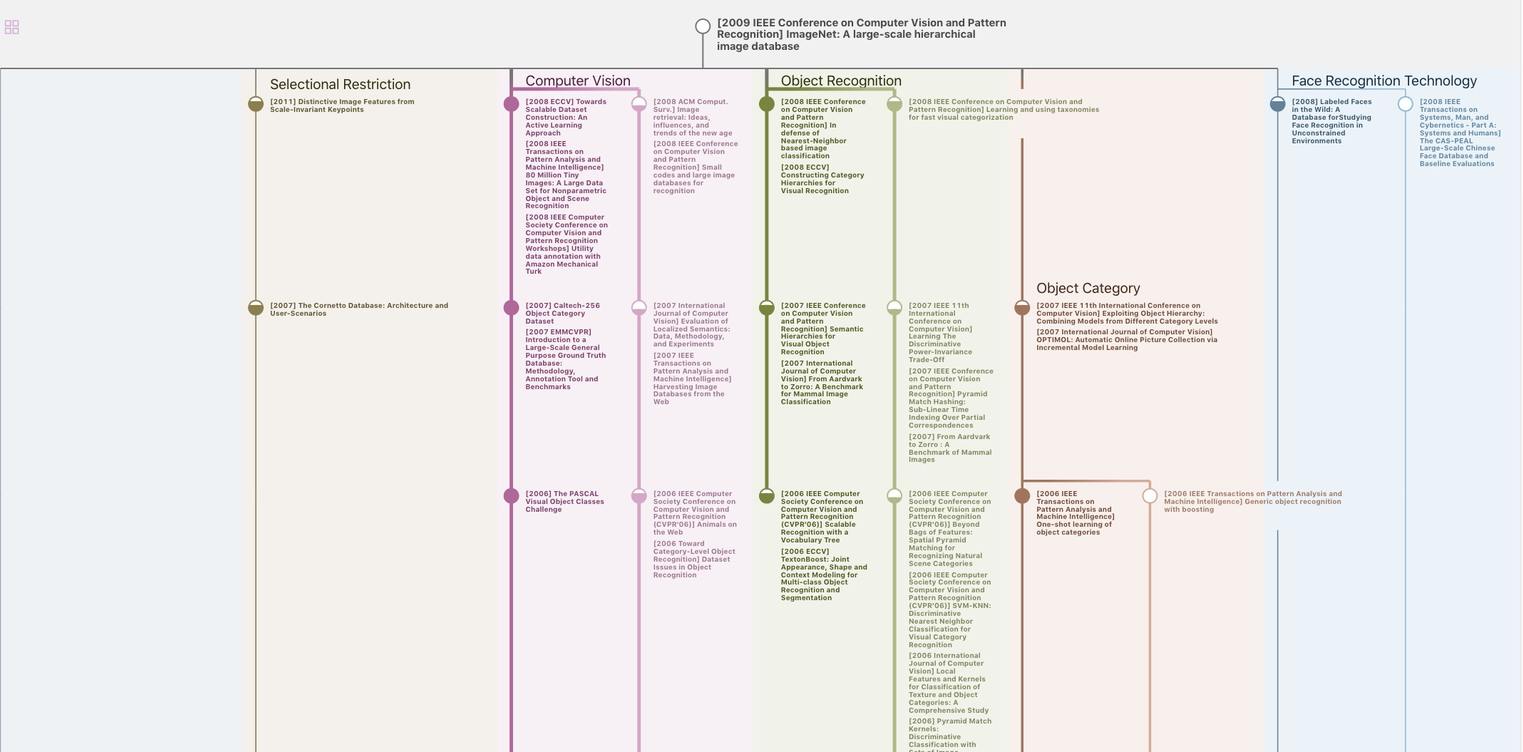
生成溯源树,研究论文发展脉络
Chat Paper
正在生成论文摘要