Burn After Reading: Online Adaptation for Cross-domain Streaming Data.
European Conference on Computer Vision(2022)
摘要
In the context of online privacy, many methods propose complex security preserving measures to protect sensitive data. In this paper we note that: not storing any sensitive data is the best form of security. We propose an online framework called “Burn After Reading”, i.e. each online sample is permanently deleted after it is processed. Our framework utilizes the labels from the public data and predicts on the unlabeled sensitive private data. To tackle the inevitable distribution shift from the public data to the private data, we propose a novel unsupervised domain adaptation algorithm that aims at the fundamental challenge of this online setting–the lack of diverse source-target data pairs. We design a Cross-Domain Bootstrapping approach, named CroDoBo, to increase the combined data diversity across domains. To fully exploit the valuable discrepancies among the diverse combinations, we employ the training strategy of multiple learners with co-supervision. CroDoBo achieves state-of-the-art online performance on four domain adaptation benchmarks. Code is available here.
更多查看译文
关键词
Domain adaptation,Online learning,Privacy preserving
AI 理解论文
溯源树
样例
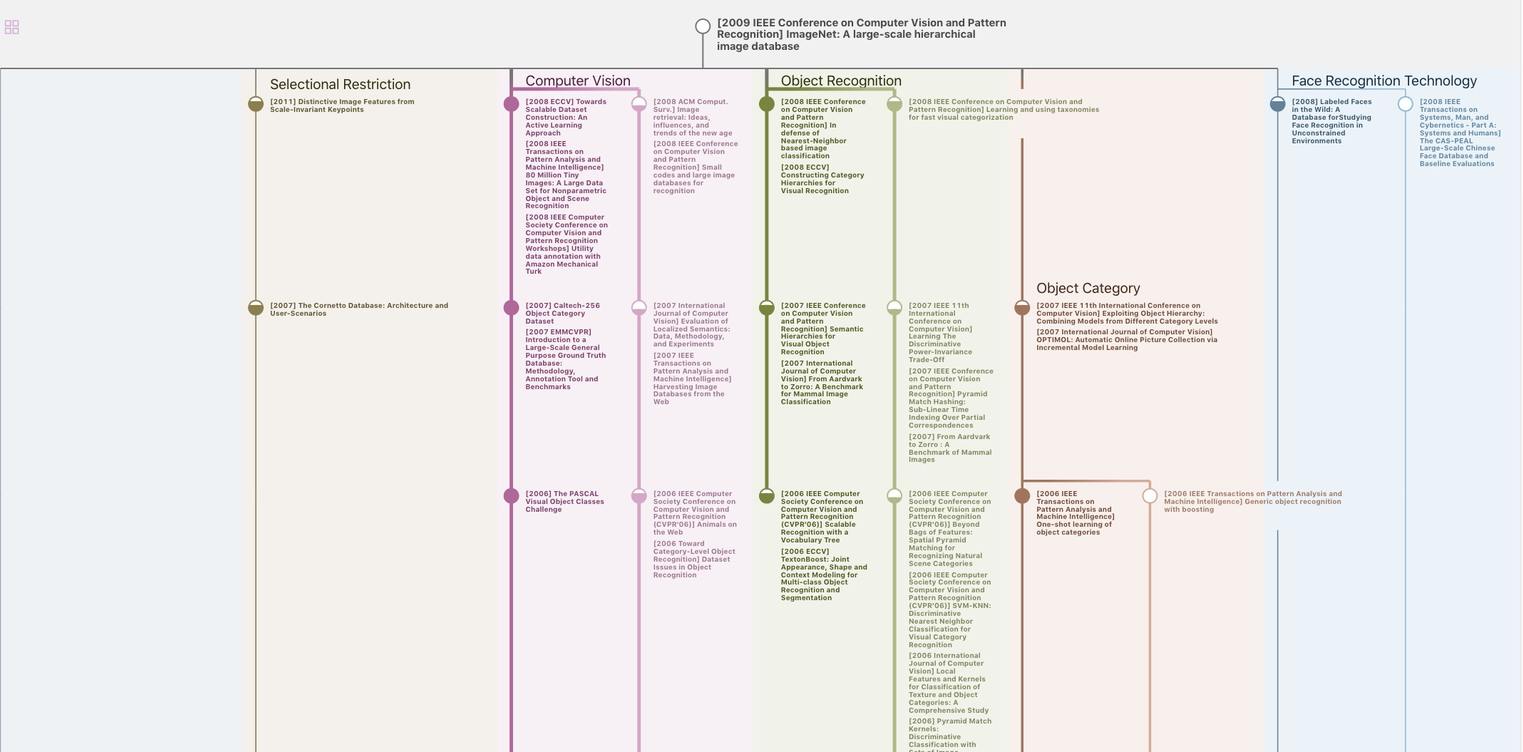
生成溯源树,研究论文发展脉络
Chat Paper
正在生成论文摘要