Unimodal Face Classification with Multimodal Training
2021 16TH IEEE INTERNATIONAL CONFERENCE ON AUTOMATIC FACE AND GESTURE RECOGNITION (FG 2021)(2021)
摘要
Face recognition is a crucial task in various multimedia applications such as security check, credential access and motion sensing games. However, the task is challenging when an input face is noisy (e.g. poor-condition RGB image) or lacks certain information (e.g. 3D face without color). In this work, we propose a Multimodal Training Unimodal Test (MTUT) framework for robust face classification, which exploits the cross-modality relationship during training and applies it as a complementary of the imperfect single modality input during testing. Technically, during training, the framework (1) builds both intra-modality and cross-modality autoencoders with the aid of facial attributes to learn latent embeddings as multimodal descriptors, (2) proposes a novel multimodal embedding divergence loss to align the heterogeneous features from different modalities, which also adaptively avoids the useless modality (if any) from confusing the model. This way, the learned autoencoders can generate robust embeddings in single-modality face classification on test stage. We evaluate our framework in two face classification datasets and two kinds of testing input: (1) poor-condition image and (2) point cloud or 3D face mesh, when both 2D and 3D modalities are available for training. We experimentally show that our MTUT framework consistently outperforms ten baselines on 2D and 3D settings of both datasets(1).
更多查看译文
关键词
multimodal training,face,classification
AI 理解论文
溯源树
样例
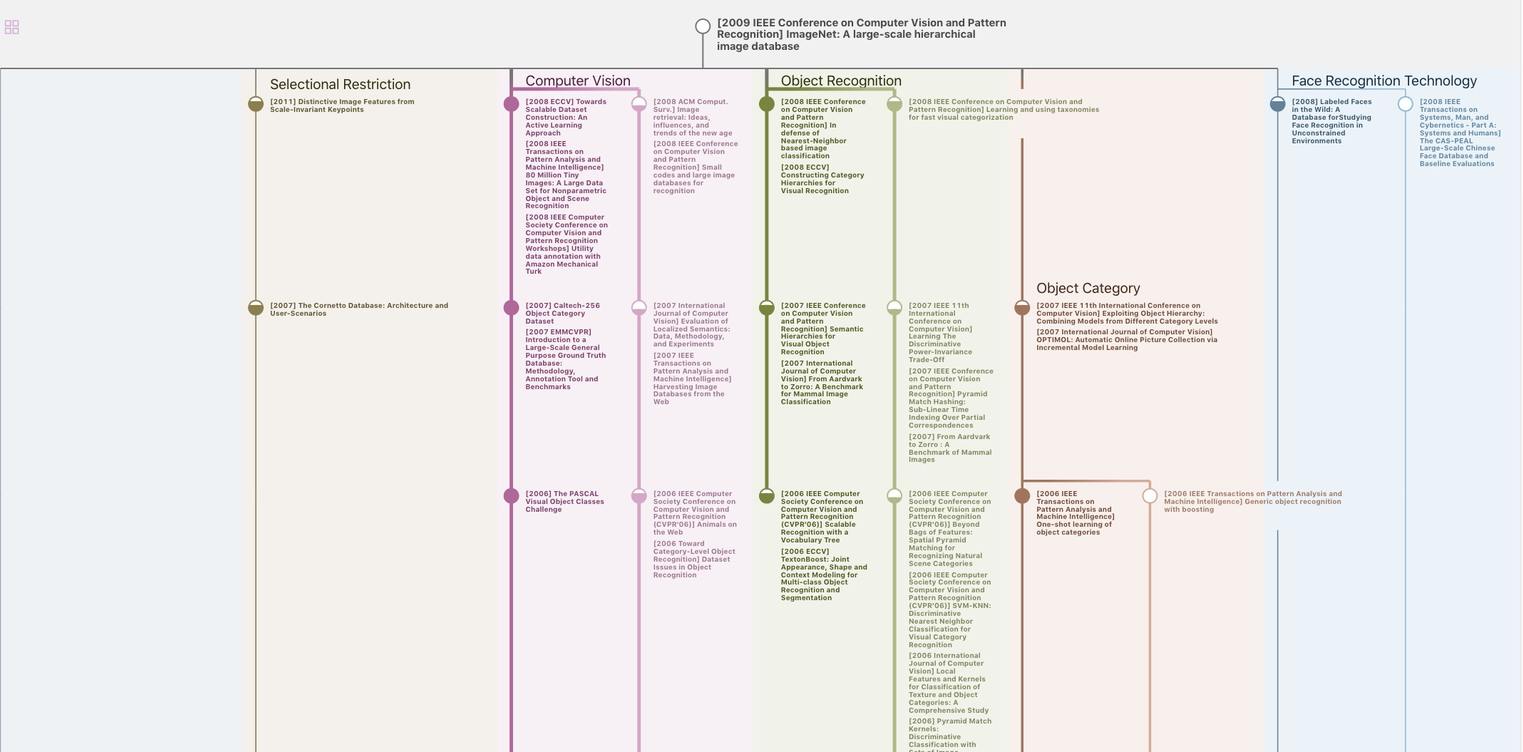
生成溯源树,研究论文发展脉络
Chat Paper
正在生成论文摘要