Adaptive Mimic: Deep Reinforcement Learning of Parameterized Bipedal Walking from Infeasible References
arxiv(2021)
摘要
Not until recently, robust robot locomotion has been achieved by deep reinforcement learning (DRL). However, for efficient learning of parametrized bipedal walking, developed references are usually required, limiting the performance to that of the references. In this paper, we propose to design an adaptive reward function for imitation learning from the references. The agent is encouraged to mimic the references when its performance is low, while to pursue high performance when it reaches the limit of references. We further demonstrate that developed references can be replaced by low-quality references that are generated without laborious tuning and infeasible to deploy by themselves, as long as they can provide a priori knowledge to expedite the learning process.
更多查看译文
关键词
parameterized bipedal,walking,deep reinforcement learning,reinforcement learning
AI 理解论文
溯源树
样例
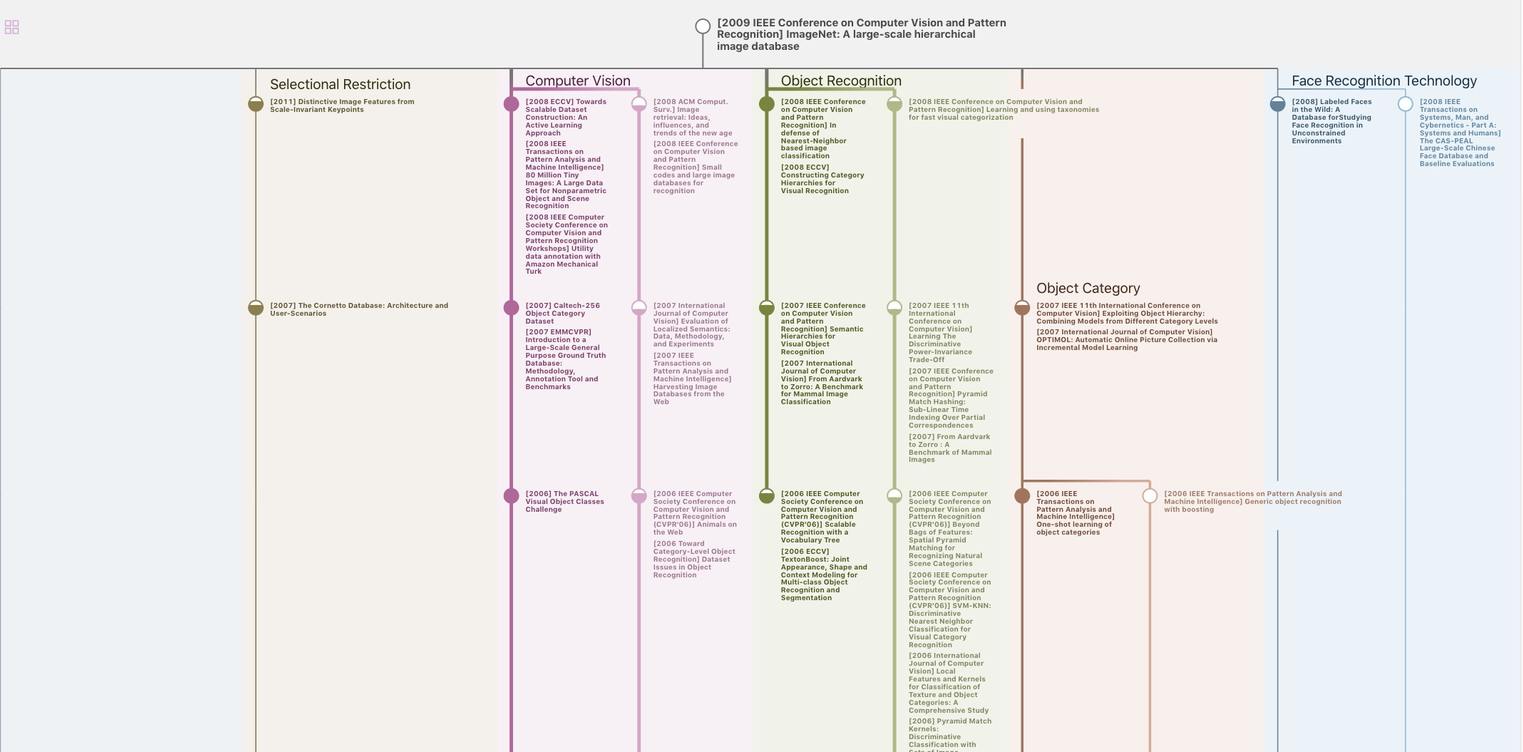
生成溯源树,研究论文发展脉络
Chat Paper
正在生成论文摘要