Adaptive smoothing mini-batch stochastic accelerated gradient method for nonsmooth convex stochastic composite optimization
arxiv(2021)
摘要
This paper considers a class of convex constrained nonsmooth convex stochastic composite optimization problems whose objective function is given by the summation of a differentiable convex component, together with a general nonsmooth but convex component. The nonsmooth component is not required to have easily obtainable proximal operator, or have the max structure that the smoothing technique in [Nesterov, 2005] can be used. In order to solve such type problems, we propose an adaptive smoothing mini-batch stochastic accelerated gradient (AdaSMSAG) method, which combines the stochastic approximation method, the Nesterov's accelerated gradient method, and the smoothing methods that allow general smoothing approximations. Convergence of the method is established. Moreover, the order of the worst-case iteration complexity is better than that of the state-of-the-art stochastic approximation methods. Numerical results are provided to illustrate the efficiency of the proposed AdaSMSAG method for a risk management in portfolio optimization and a family of Wasserstein distributionally robust support vector machine problems with real data.
更多查看译文
关键词
gradient method,optimization,stochastic,composite,mini-batch
AI 理解论文
溯源树
样例
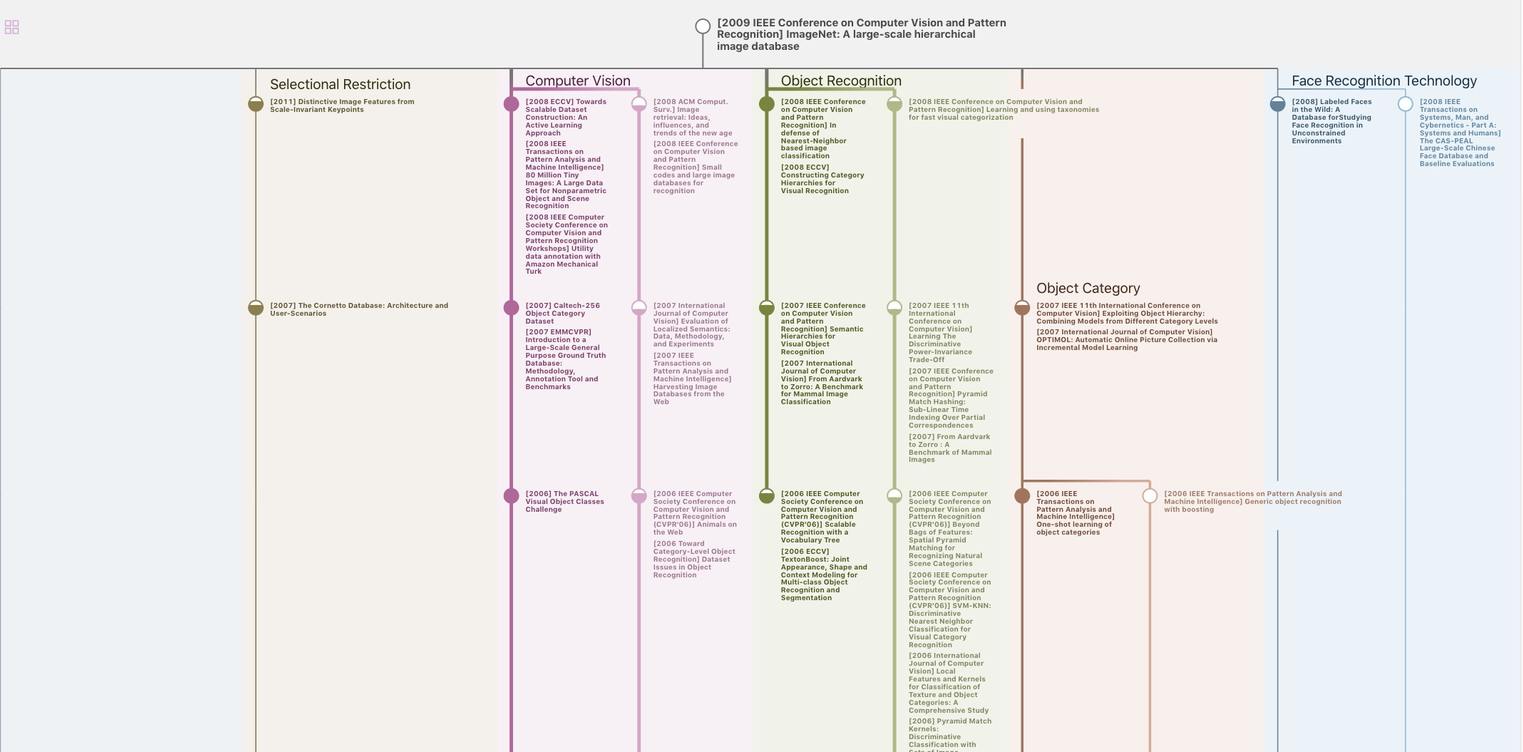
生成溯源树,研究论文发展脉络
Chat Paper
正在生成论文摘要