Associative memories using complex-valued Hopfield networks based on spin-torque oscillator arrays.
Neuromorph. Comput. Eng.(2022)
摘要
Simulations of complex-valued Hopfield networks based on spin-torque oscillators can recover phase-encoded images. Sequences of memristor-augmented inverters provide tunable delay elements that implement complex weights by phase shifting the oscillatory output of the oscillators. Pseudo-inverse training suffices to store at least 12 images in a set of 192 oscillators, representing 16$\times$12 pixel images. The energy required to recover an image depends on the desired error level. For the oscillators and circuitry considered here, 5 % root mean square deviations from the ideal image require approximately 5 $\mu$s and consume roughly 130 nJ. Simulations show that the network functions well when the resonant frequency of the oscillators can be tuned to have a fractional spread less than $10^{-3}$, depending on the strength of the feedback.
更多查看译文
关键词
hopfield networks,complex-valued,spin-torque
AI 理解论文
溯源树
样例
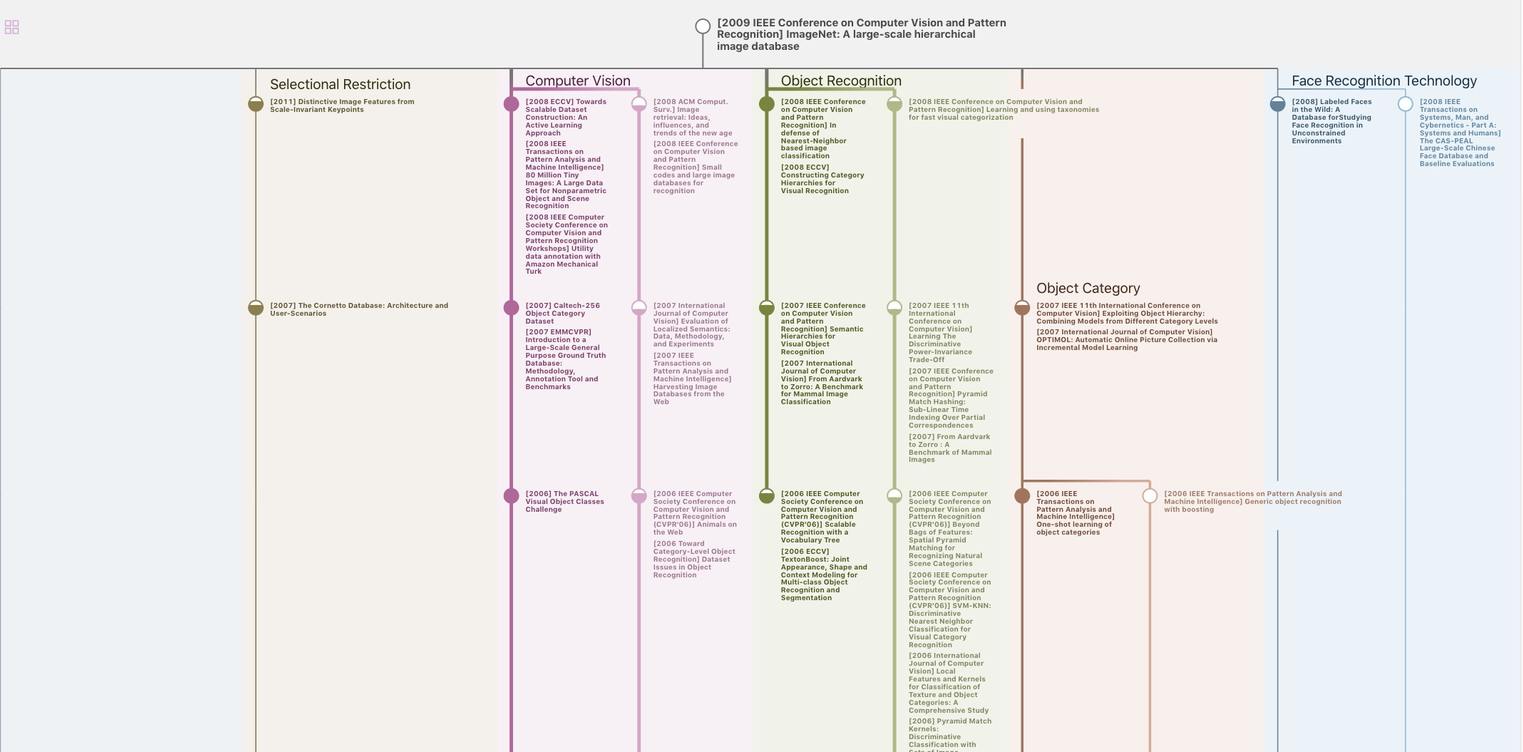
生成溯源树,研究论文发展脉络
Chat Paper
正在生成论文摘要