Learning Query Expansion over the Nearest Neighbor Graph.
British Machine Vision Conference(2021)
摘要
Query Expansion (QE) is a well established method for improving retrieval metrics in image search applications. When using QE, the search is conducted on a new query vector, constructed using an aggregation function over the query and images from the database. Recent works gave rise to QE techniques in which the aggregation function is learned, whereas previous techniques were based on hand-crafted aggregation functions, e.g., taking the mean of the query's nearest neighbors. However, most QE methods have focused on aggregation functions that work directly over the query and its immediate nearest neighbors. In this work, a hierarchical model, Graph Query Expansion (GQE), is presented, which is learned in a supervised manner and performs aggregation over an extended neighborhood of the query, thus increasing the information used from the database when computing the query expansion, and using the structure of the nearest neighbors graph. The technique achieves state-of-the-art results over known benchmarks.
更多查看译文
关键词
query expansion,learning
AI 理解论文
溯源树
样例
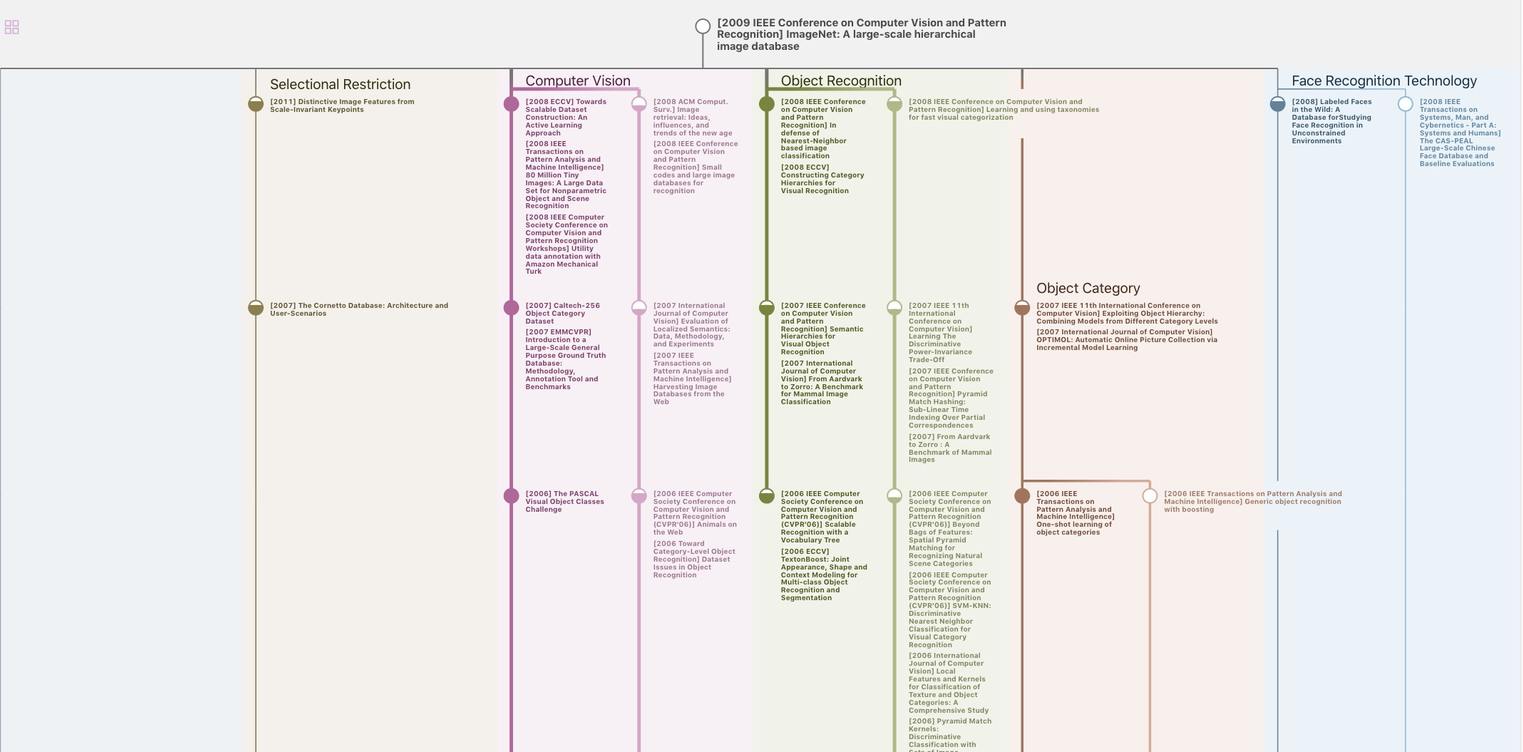
生成溯源树,研究论文发展脉络
Chat Paper
正在生成论文摘要