Remaining useful life prediction using an integrated Laplacian-LSTM network on machinery components
APPLIED SOFT COMPUTING(2021)
摘要
Accurate remaining useful life (RUL) analysis of a machinery system is of great importance. Such systems work in long-term operations in which unexpected failures often occur. Due to the rapid development of computer technology, the deep learning model has supplanted physical-based RUL analysis. The data-driven approach using the deep learning model is capable of providing an accurate RUL analysis. However, an accurate analysis using deep learning comes with challenges and costs. In current RUL analysis practice, the deep learning hyperparameters are manually selected, which hinders the deep network from reaching the local optima. Additionally, current practice uses powerful signal processing methods with complicated prediction indicators. Therefore, a novel methodological step is proposed to tackle this problem by integrating the Laplacian score (LS), random search optimisation and long short-term memory (LSTM). The proposed system, called integrated LaplacianLSTM, produced accurate RUL analyses on the IEEE PHM 2012 Competition and IMS bearing datasets, showing significant improvement in prediction accuracy. This system increases prediction accuracy by 18% compared to other available RUL methods in similar studies. (C) 2021 Published by Elsevier B.V.
更多查看译文
关键词
Remaining useful life prediction, Feature selection, Automated hyperparameter selection, Long short-term memory, Rotating machinery
AI 理解论文
溯源树
样例
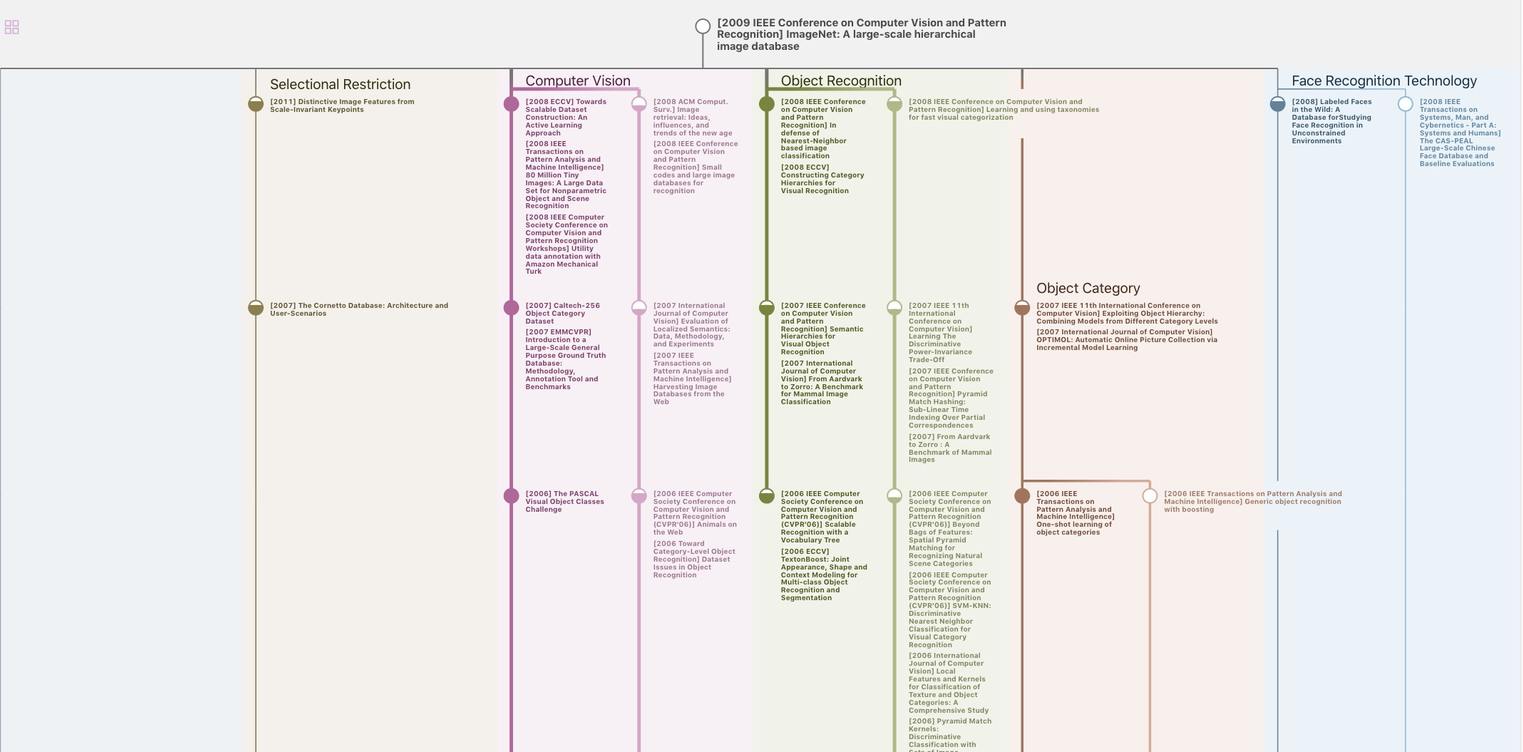
生成溯源树,研究论文发展脉络
Chat Paper
正在生成论文摘要