Causal-based Time Series Domain Generalization for Vehicle Intention Prediction
IEEE International Conference on Robotics and Automation(2022)
摘要
Accurately predicting the possible behaviors of traffic participants is an essential capability for autonomous vehicles. Since autonomous vehicles need to navigate in dynamically changing environments, they are expected to make accurate predictions regardless of where they are and what driving circumstances they encountered. Therefore, generalization capability to unseen domains is crucial for prediction models when autonomous vehicles are deployed in the real world. In this paper, we aim to address the domain generalization problem for vehicle intention prediction tasks and a causal-based time series domain generalization (CTSDG) model is proposed. We construct a structural causal model for vehicle intention prediction tasks to learn an invariant representation of input driving data for domain generalization. We further integrate a recurrent latent variable model into our structural causal model to better capture temporal latent dependencies from time-series input data. The effectiveness of our approach is evaluated via real-world driving data. We demonstrate that our proposed method has consistent improvement on prediction accuracy compared to other state-of-the-art domain generalization and behavior prediction methods.
更多查看译文
关键词
time series domain generalization,vehicle intention
AI 理解论文
溯源树
样例
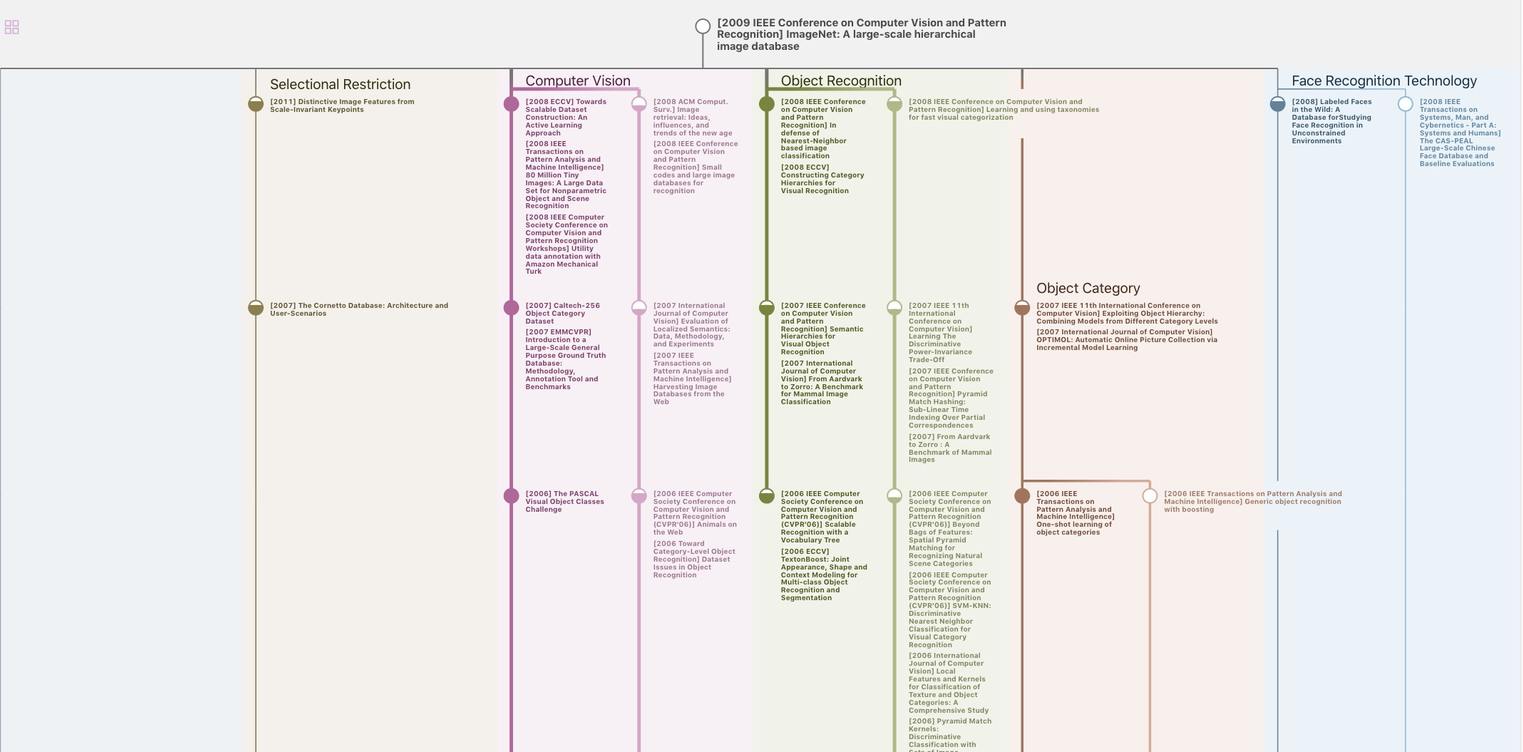
生成溯源树,研究论文发展脉络
Chat Paper
正在生成论文摘要