Adversarial Attacks against a Satellite-borne Multispectral Cloud Detector
arxiv(2021)
摘要
Data collected by Earth-observing (EO) satellites are often afflicted by cloud cover. Detecting the presence of clouds -- which is increasingly done using deep learning -- is crucial preprocessing in EO applications. In fact, advanced EO satellites perform deep learning-based cloud detection on board the satellites and downlink only clear-sky data to save precious bandwidth. In this paper, we highlight the vulnerability of deep learning-based cloud detection towards adversarial attacks. By optimising an adversarial pattern and superimposing it into a cloudless scene, we bias the neural network into detecting clouds in the scene. Since the input spectra of cloud detectors include the non-visible bands, we generated our attacks in the multispectral domain. This opens up the potential of multi-objective attacks, specifically, adversarial biasing in the cloud-sensitive bands and visual camouflage in the visible bands. We also investigated mitigation strategies against the adversarial attacks. We hope our work further builds awareness of the potential of adversarial attacks in the EO community.
更多查看译文
关键词
satellite-borne
AI 理解论文
溯源树
样例
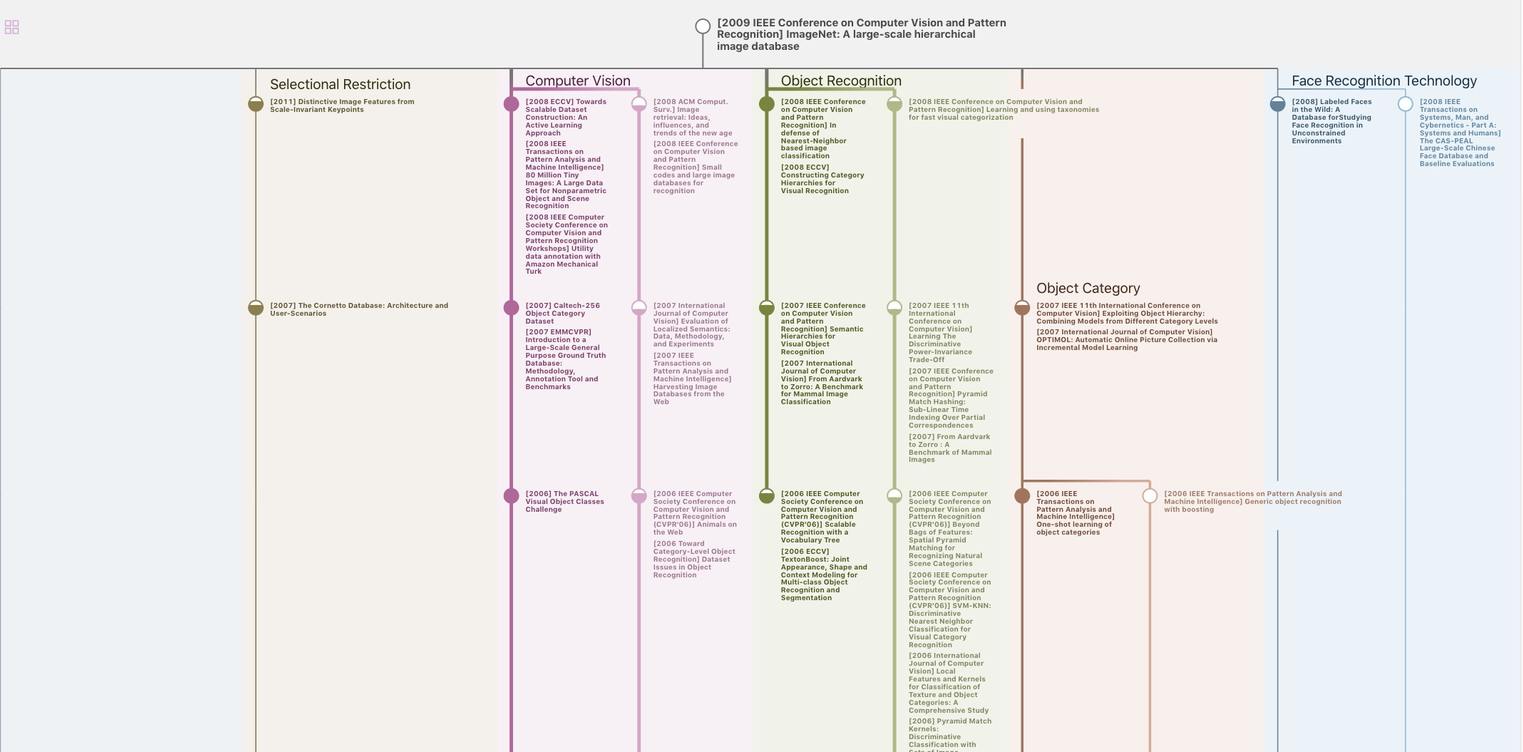
生成溯源树,研究论文发展脉络
Chat Paper
正在生成论文摘要