High-precision inversion of dynamic radiography using hydrodynamic features
OPTICS EXPRESS(2022)
摘要
While radiography is routinely used to probe complex, evolving density fields in research areas ranging from materials science to shock physics to inertial confinement fusion and other national security applications, complications resulting from noise, scatter, complex beam dynamics, etc. prevent current methods of reconstructing density from being accurate enough to identify the underlying physics with sufficient confidence. In this work, we show that using only features that are robustly identifiable in radiographs and combining them with the underlying hydrodynamic equations of motion using a machine learning approach of a conditional generative adversarial network (cGAN) provides a new and effective approach to determine density fields from a dynamic sequence of radiographs. In particular, we demonstrate the ability of this method to outperform a traditional, direct radiograph to density reconstruction in the presence of scatter, even when relatively small amounts of scatter are present. Our experiments on synthetic data show that the approach can produce high quality, robust reconstructions. We also show that the distance (in feature space) between a testing radiograph and the training set can serve as a diagnostic of the accuracy of the reconstruction. (C) 2022 Optica Publishing Group under the terms of the Optica Open Access Publishing Agreement
更多查看译文
关键词
dynamic radiography,high-precision
AI 理解论文
溯源树
样例
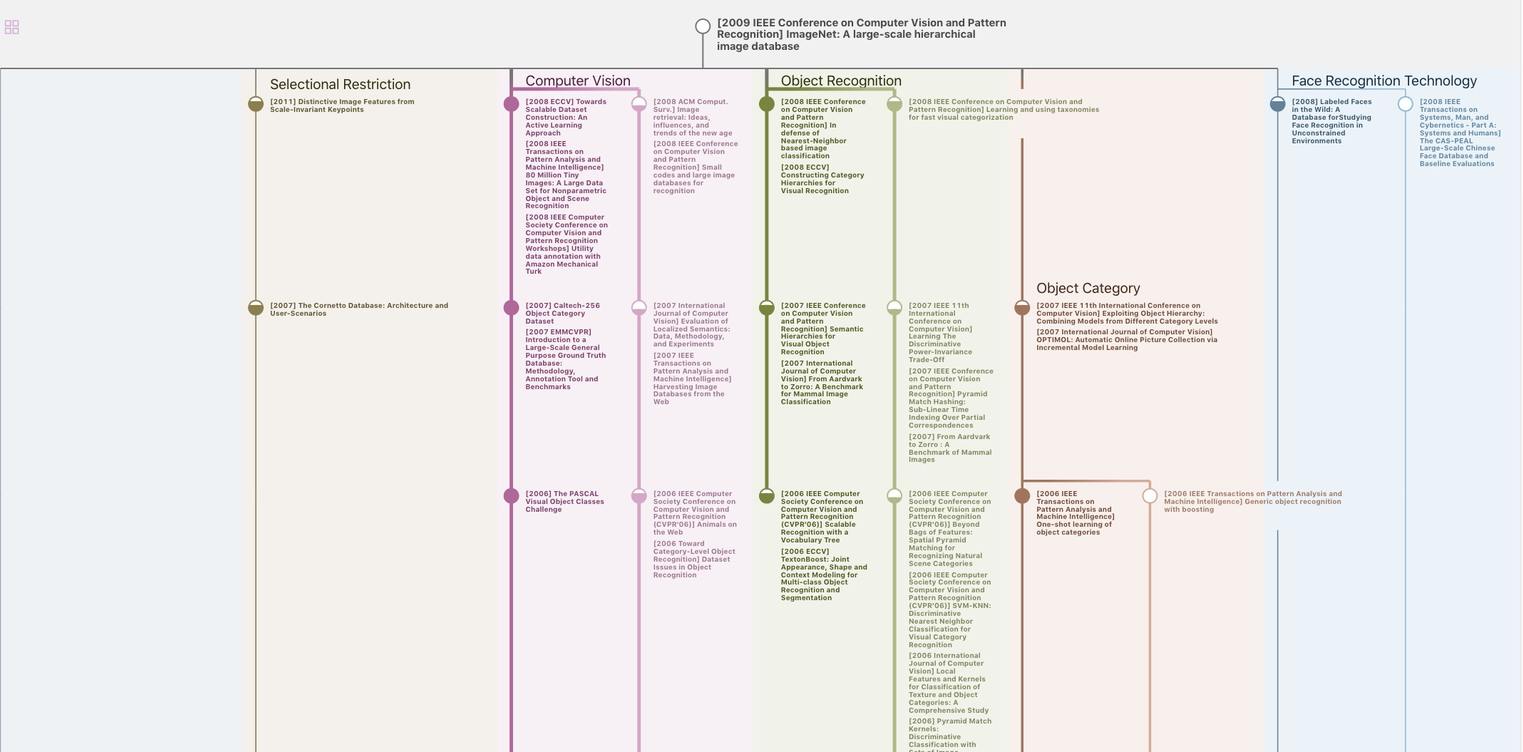
生成溯源树,研究论文发展脉络
Chat Paper
正在生成论文摘要