Left ventricular non-compaction cardiomyopathy automatic diagnosis using a deep learning approach
COMPUTER METHODS AND PROGRAMS IN BIOMEDICINE(2022)
摘要
Background and Objective: Left ventricular non-compaction (LVNC) is an uncommon cardiomyopathy characterised by a thick and spongy left ventricle wall caused by the high presence of trabeculae (hypertrabeculation). Recently, the percentage of the trabecular volume to the total volume of the external wall of the left ventricle (V T %) has been proposed to diagnose this illness. Methods: This paper presents the use of a deep learning-based method to measure the (V T %) value and diagnose this rare cardiomyopathy. The population used in this research was composed of 277 patients suffering from hypertrophic cardiomyopathy. 134 patients only suffered hypertrophic cardiomyopathy, and 143 also suffered left ventricular non-compaction. Our deep learning solution is based on a 2D U-Net. This artificial neural network (ANN) was trained on short-axis magnetic resonance imaging to segment the left ventricle's internal cavity, external wall, and trabecular tissue. 5-fold cross-validation was performed to ensure the robustness of the results. The Dice coefficient of the three classes was computed as a measure of the precision of the segmentation. Based on this segmentation, the percentage of the trabecular volume (VT%) was computed. Two specialist cardiologists rated the segmentation produced by the neural network for 25 patients to evaluate the clinical validity of the outputs. The computed VT% was used to automatically diagnose the 277 patients depending on whether or not a given threshold was exceeded. A receiver operating characteristic analysis was also performed. Results: According to the cross-validation results, the average and standard deviation of the Dice coefficient for the internal cavity, external wall, and trabeculae were 0.96 +/- 0.00, 0.89 +/- 0.00, and 0.84 +/- 0.00, respectively. The cardiologists rated 99.5% of the evaluated segmentations as clinically valid for diagnosis, outperforming existing automatic traditional tools. The area under the ROC curve was 0.94 (95% confidence interval, 0.91-0.96). The accuracy, sensitivity, and specificity values of diagnosis using a threshold of 25% were 0.87, 0.93, and 0.80, respectively. Conclusions: The U-Net neural network can achieve excellent results in the delineation of different cardiac structures of short-axis cardiac MRI. The high-quality segmentation allows for the correct measurement of left ventricular hyper-trabeculation and a definitive diagnosis of LVNC illness. Using this kind of solution could lead to more objective and faster analysis, reducing human error and time spent by cardiologists. (C) 2021 Elsevier B.V. All rights reserved.
更多查看译文
关键词
Deep learning, Left ventricular non-compaction, MRI Image segmentation, Hyper-trabeculation, Convolutional neural network
AI 理解论文
溯源树
样例
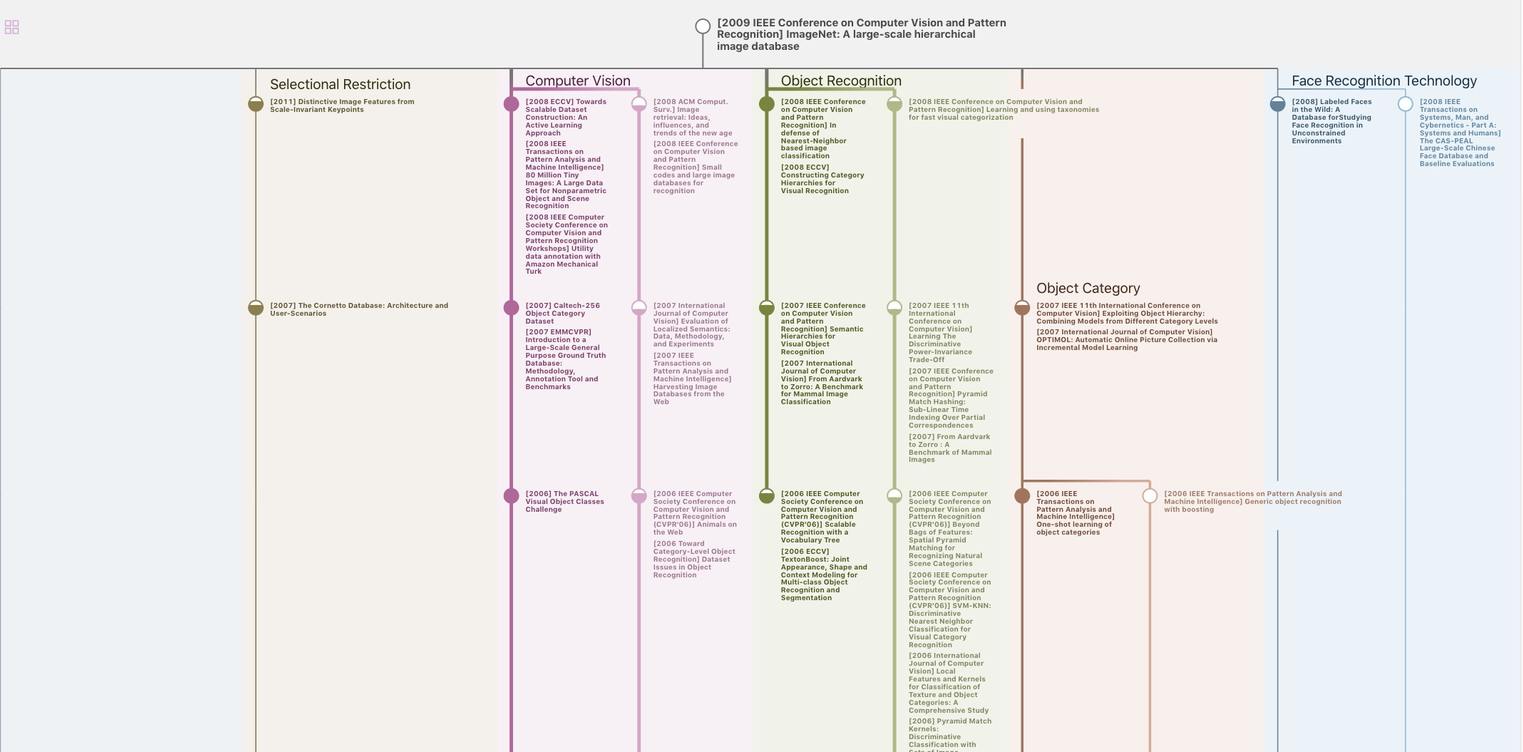
生成溯源树,研究论文发展脉络
Chat Paper
正在生成论文摘要