The Conditional Super Learner
IEEE Transactions on Pattern Analysis and Machine Intelligence(2022)
摘要
Using cross validation to select the best model from a library is standard practice in machine learning. Similarly, meta learning is a widely used technique where models previously developed are combined (mainly linearly) with the expectation of improving performance with respect to individual models. In this article we consider the Conditional Super Learner (CSL), an algorithm that selects the best model candidate from a library of models conditional on the covariates. The CSL expands the idea of using cross validation to select the best model and merges it with meta learning. We propose an optimization algorithm that finds a local minimum to the problem posed and proves that it converges at a rate faster than
$O_p(n^{-1/4})$
. We offer empirical evidence that: (1) CSL is an excellent candidate to substitute stacking and (2) CLS is suitable for the analysis of Hierarchical problems. Additionally, implications for global interpretability are emphasized.
更多查看译文
关键词
Algorithms,Machine Learning
AI 理解论文
溯源树
样例
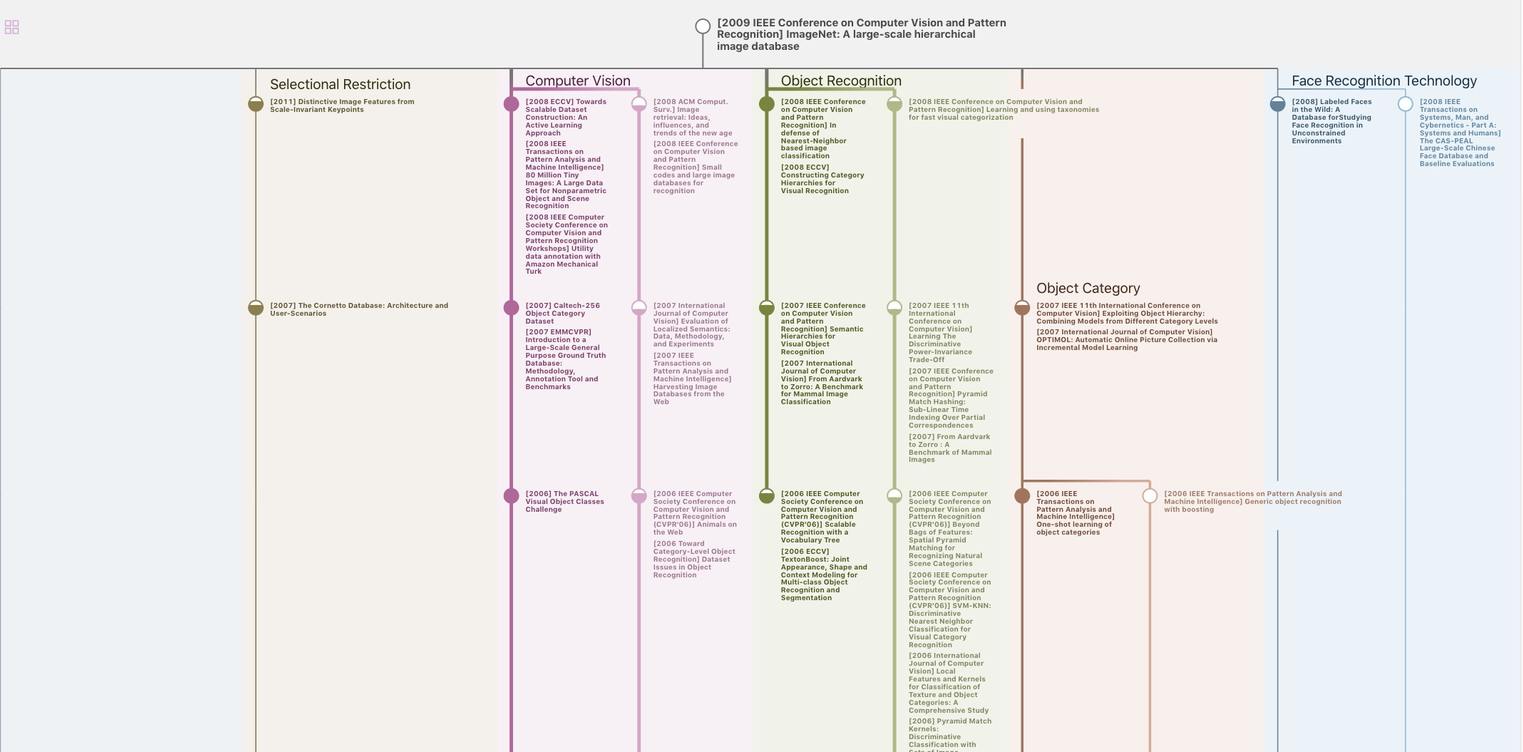
生成溯源树,研究论文发展脉络
Chat Paper
正在生成论文摘要