Learning Spatial-Temporal Graphs for Active Speaker Detection
arxiv(2021)
摘要
We address the problem of active speaker detection through a new framework, called SPELL, that learns long-range multimodal graphs to encode the inter-modal relationship between audio and visual data. We cast active speaker detection as a node classification task that is aware of longer-term dependencies. We first construct a graph from a video so that each node corresponds to one person. Nodes representing the same identity share edges between them within a defined temporal window. Nodes within the same video frame are also connected to encode inter-person interactions. Through extensive experiments on the Ava-ActiveSpeaker dataset, we demonstrate that learning graph-based representation, owing to its explicit spatial and temporal structure, significantly improves the overall performance. SPELL outperforms several relevant baselines and performs at par with state of the art models while requiring an order of magnitude lower computation cost.
更多查看译文
关键词
detection,spatial-temporal
AI 理解论文
溯源树
样例
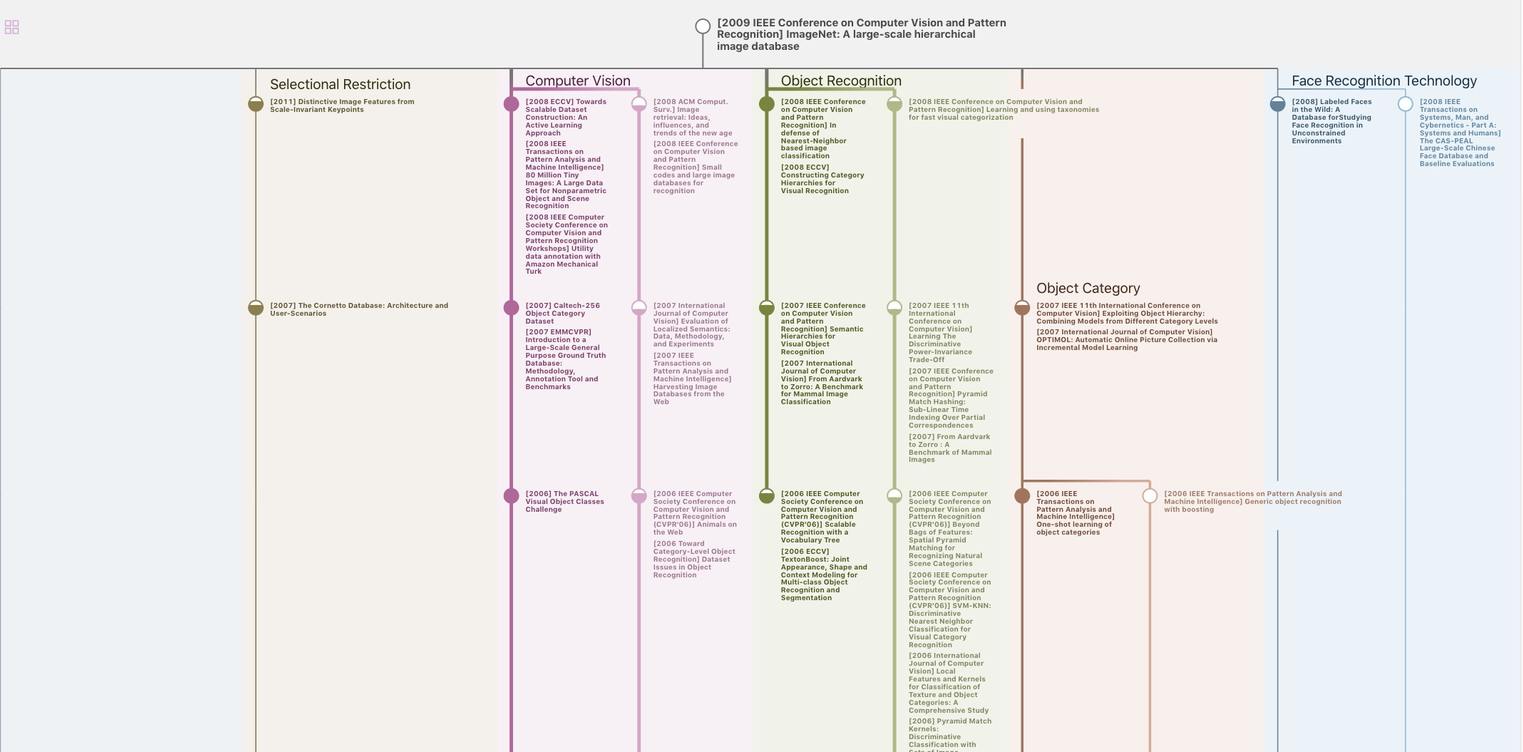
生成溯源树,研究论文发展脉络
Chat Paper
正在生成论文摘要