Classification of Fermi-LAT blazars with Bayesian neural networks
JOURNAL OF COSMOLOGY AND ASTROPARTICLE PHYSICS(2022)
摘要
The use of Bayesian neural networks is a novel approach for the classification of gamma-ray sources. We focus on the classification of Fermi-LAT blazar candidates, which can be divided into BL Lacertae objects and Flat Spectrum Radio Quasars. In contrast to conventional dense networks, Bayesian neural networks provide a reliable estimate of the uncertainty of the network predictions. We explore the correspondence between conventional and Bayesian neural networks and the effect of data augmentation. We find that Bayesian neural networks provide a robust classifier with reliable uncertainty estimates and are particularly well suited for classification problems that are based on comparatively small and imbalanced data sets. The results of our blazar candidate classification are valuable input for population studies aimed at constraining the blazar luminosity function and to guide future observational campaigns.
更多查看译文
关键词
active galactic nuclei, gamma ray experiments, gamma ray theory, Machine learning
AI 理解论文
溯源树
样例
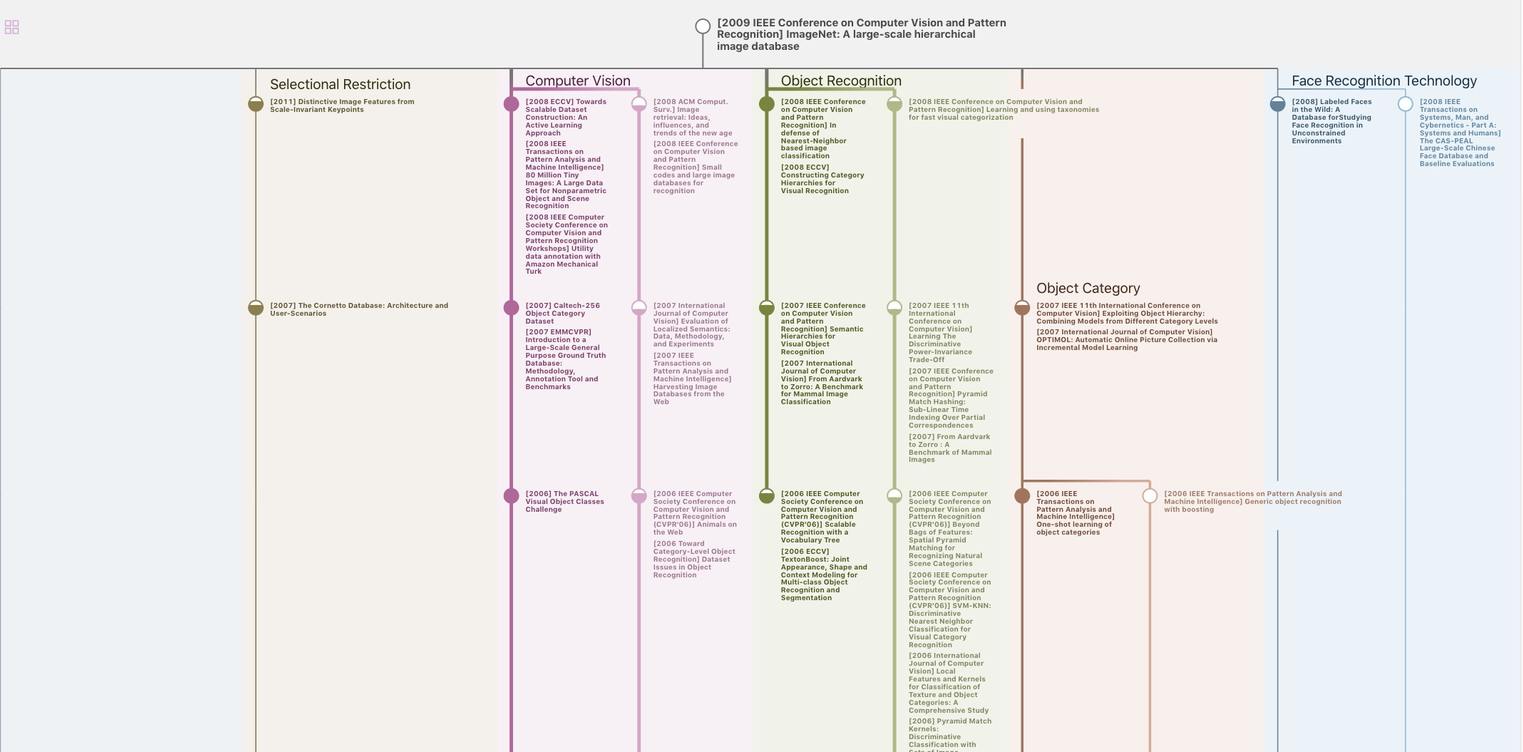
生成溯源树,研究论文发展脉络
Chat Paper
正在生成论文摘要