GANORCON: Are Generative Models Useful for Few-shot Segmentation?
IEEE Conference on Computer Vision and Pattern Recognition(2022)
摘要
Advances in generative modeling based on GANs has motivated the community to find their use beyond image generation and editing tasks. In particular, several recent works have shown that GAN representations can be re-purposed for discriminative tasks such as part segmentation, especially when training data is limited. But how do these improvements stack-up against recent advances in self-supervised learning? Motivated by this we present an alternative approach based on contrastive learning and compare their performance on standard few-shot part segmentation benchmarks. Our experiments reveal that not only do the GAN-based approach offer no significant performance advantage, their multi-step training is complex, nearly an order-of-magnitude slower, and can introduce additional bias. These experiments suggest that the inductive biases of generative models, such as their ability to disentangle shape and texture, are well captured by standard feed-forward networks trained using contrastive learning. These experiments suggest that the inductive biases present in current generative models, such as their ability to disentangle shape and texture, are well captured by standard feed-forward networks trained using contrastive learning.
更多查看译文
关键词
Self-& semi-& meta- Representation learning, Segmentation,grouping and shape analysis
AI 理解论文
溯源树
样例
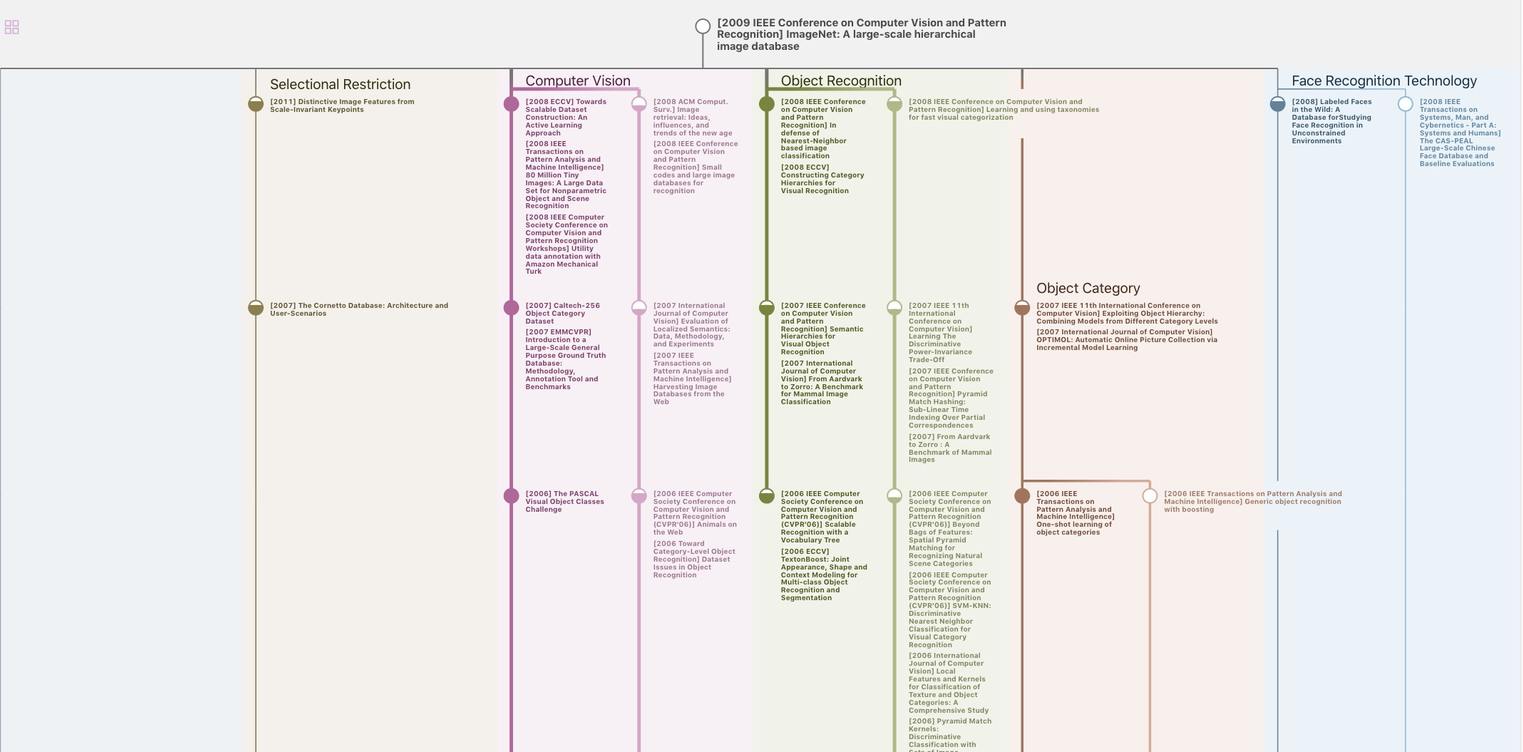
生成溯源树,研究论文发展脉络
Chat Paper
正在生成论文摘要