The Physics of Machine Learning: An Intuitive Introduction for the Physical Scientist
arxiv(2021)
摘要
This article is intended for physical scientists who wish to gain deeper insights into machine learning algorithms which we present via the domain they know best, physics. We begin with a review of two energy-based machine learning algorithms, Hopfield networks and Boltzmann machines, and their connection to the Ising model. This serves as a foundation to understand the phenomenon of learning more generally. Equipped with this intuition we then delve into additional, more "practical," machine learning architectures including feedforward neural networks, convolutional neural networks, and autoencoders. We also provide code that explicitly demonstrates training a neural network with gradient descent.
更多查看译文
关键词
machine learning,physics,intuitive introduction,physical scientist
AI 理解论文
溯源树
样例
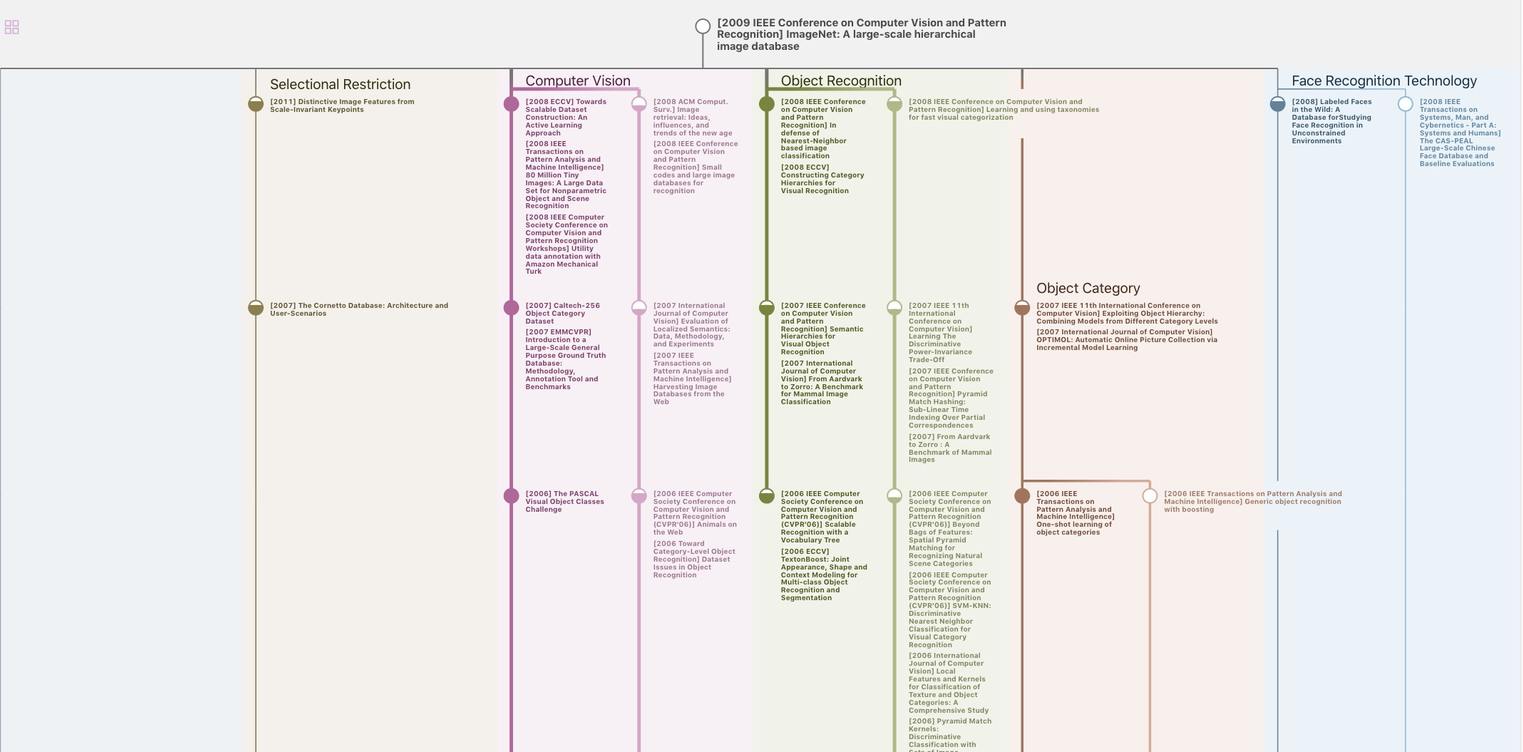
生成溯源树,研究论文发展脉络
Chat Paper
正在生成论文摘要