AWESOME: Empowering Scalable Data Science on Social Media Data with an Optimized Tri-Store Data System
arxiv(2022)
摘要
Modern data science applications increasingly use heterogeneous data sources and analytics. This has led to growing interest in polystore systems, especially analytical polystores. In this work, we focus on emerging multi-data model analytics workloads over social media data that fluidly straddle relational, graph, and text analytics. Instead of a generic polystore, we build a "tri-store" system that is more aware of the underlying data models to better optimize execution to improve scalability and runtime efficiency. We name our system AWESOME (Analytics WorkbEnch for SOcial MEdia). It features a powerful domain-specific language named ADIL. ADIL builds on top of underlying query engines (e.g., SQL and Cypher) and features native data types for succinctly specifying cross-engine queries and NLP operations, as well as automatic in-memory and query optimizations. Using real-world tri-model analytical workloads and datasets, we empirically demonstrate the functionalities of AWESOME for scalable data science over social media data and evaluate its efficiency.
更多查看译文
关键词
scalable data science,social media data,social media,tri-store
AI 理解论文
溯源树
样例
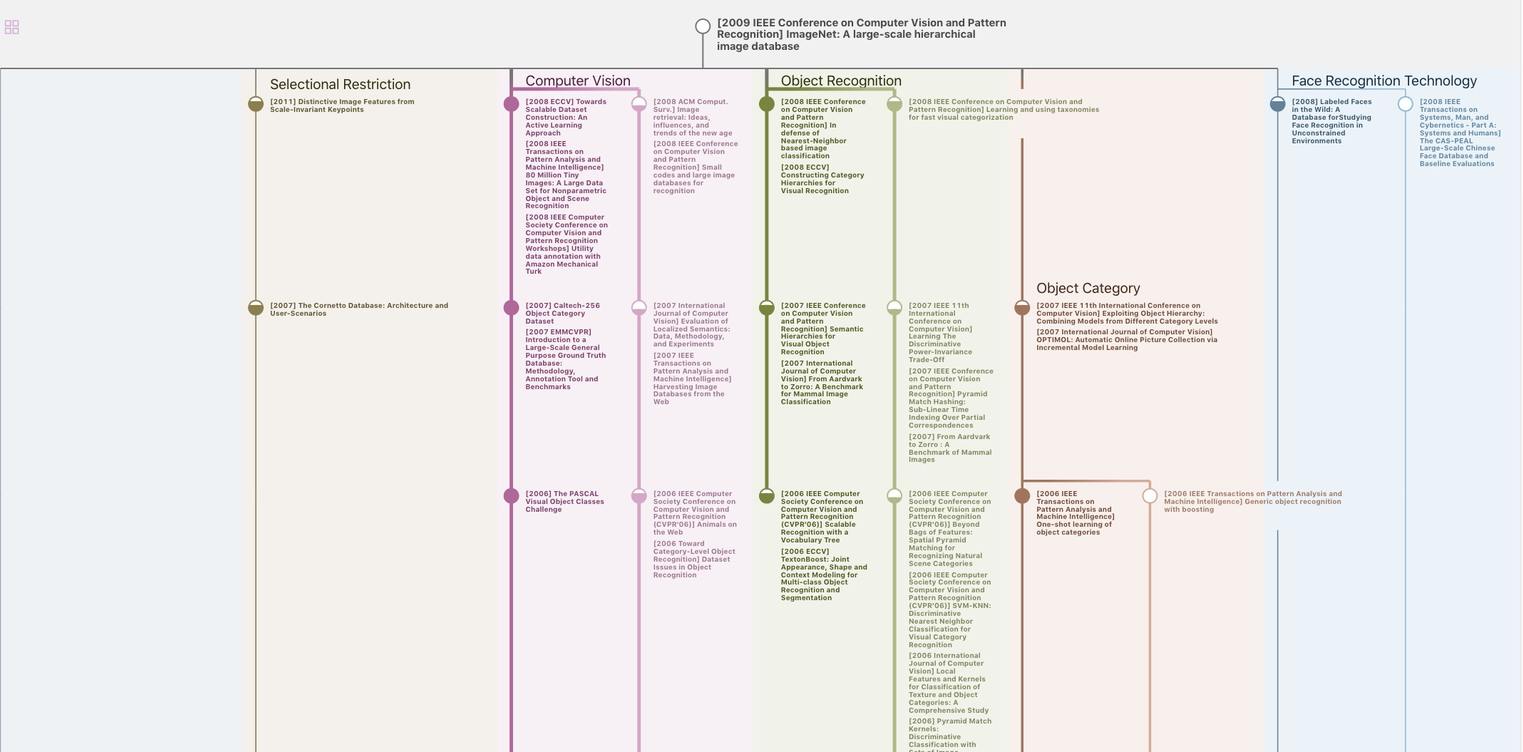
生成溯源树,研究论文发展脉络
Chat Paper
正在生成论文摘要