A Globally Stable LPNN Model for Sparse Approximation.
IEEE transactions on neural networks and learning systems(2023)
摘要
The objective of compressive sampling is to determine a sparse vector from an observation vector. This brief describes an analog neural method to achieve the objective. Unlike previous analog neural models which either resort to the l(1)-norm approximation or are with local convergence only, the proposed method avoids any approximation of the l(1)-norm term and is probably capable of leading to the optimum solution. Moreover, its computational complexity is lower than that of the other three comparison analog models. Simulation results show that the error performance of the proposed model is comparable to several state-of-the-art digital algorithms and analog models and that its convergence is faster than that of the comparison analog neural models.
更多查看译文
关键词
Basis pursuit (BP),Lagrange programming neural network (LPNN),locally competitive algorithm (LCA),projection theorem,sparse approximation
AI 理解论文
溯源树
样例
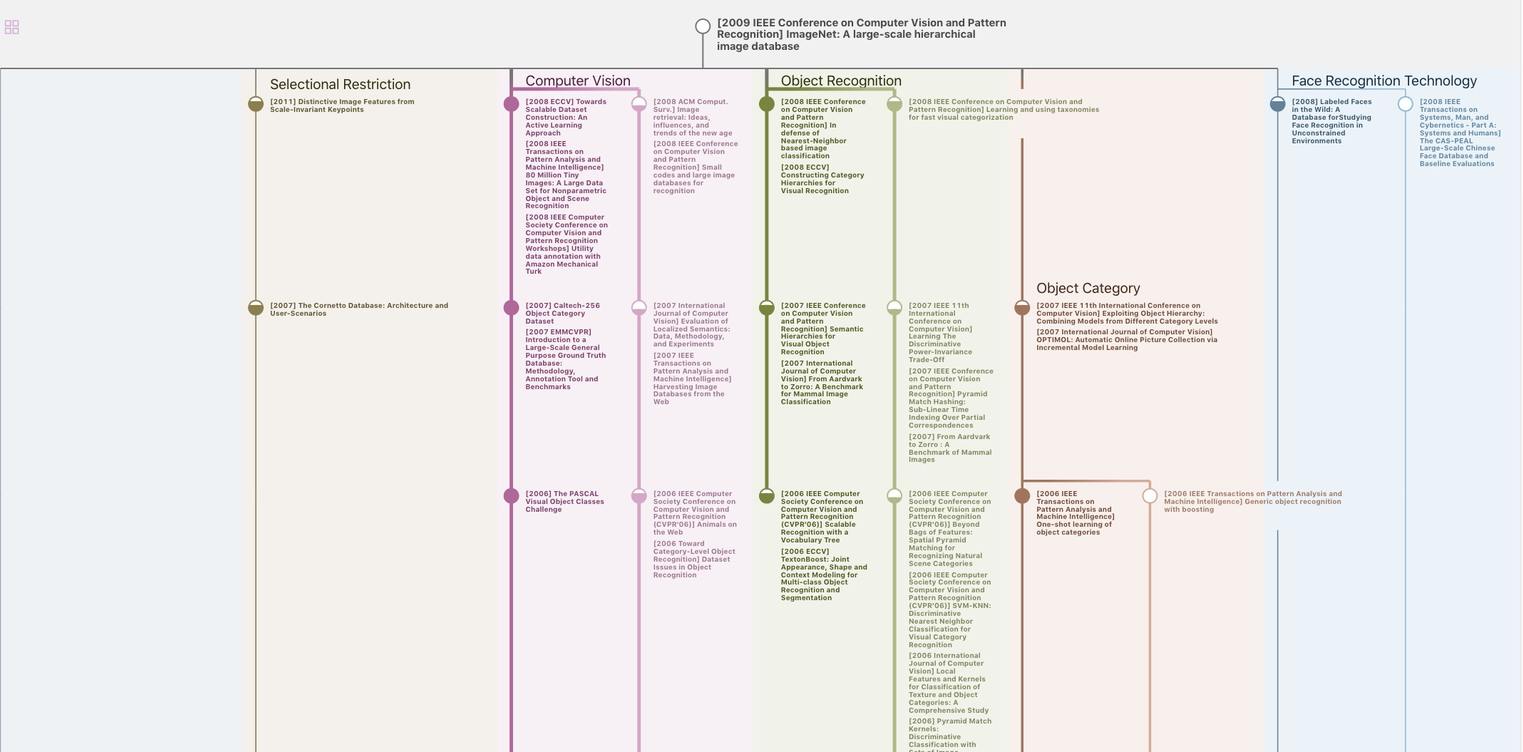
生成溯源树,研究论文发展脉络
Chat Paper
正在生成论文摘要