Generalized deep iterative reconstruction for sparse-view CT imaging
PHYSICS IN MEDICINE AND BIOLOGY(2022)
摘要
Sparse-view CT is a promising approach for reducing the x-ray radiation dose in clinical CT imaging. However, the CT images reconstructed from the conventional filtered backprojection algorithm suffer from severe streaking artifacts. Iterative reconstruction algorithms have been widely adopted to mitigate these streaking artifacts, but they may prolong the CT imaging time due to the intense data-specific computations. Recently, a model-driven deep learning CT image reconstruction method, which unrolls the iterative optimization procedures into a deep neural network, has shown exciting prospects for improving image quality and shortening the reconstruction time. In this work, we explore a generalized unrolling scheme for such an iterative model to further enhance its performance on sparse-view CT imaging. By using it, the iteration parameters, regularizer term, data-fidelity term and even the mathematical operations are all assumed to be learned and optimized via network training. Results from the numerical and experimental sparse-view CT imaging demonstrate that the newly proposed network with the maximum generalization provides the best reconstruction performance.
更多查看译文
关键词
sparse-view CT, image reconstruction, deep learning, model-driven network
AI 理解论文
溯源树
样例
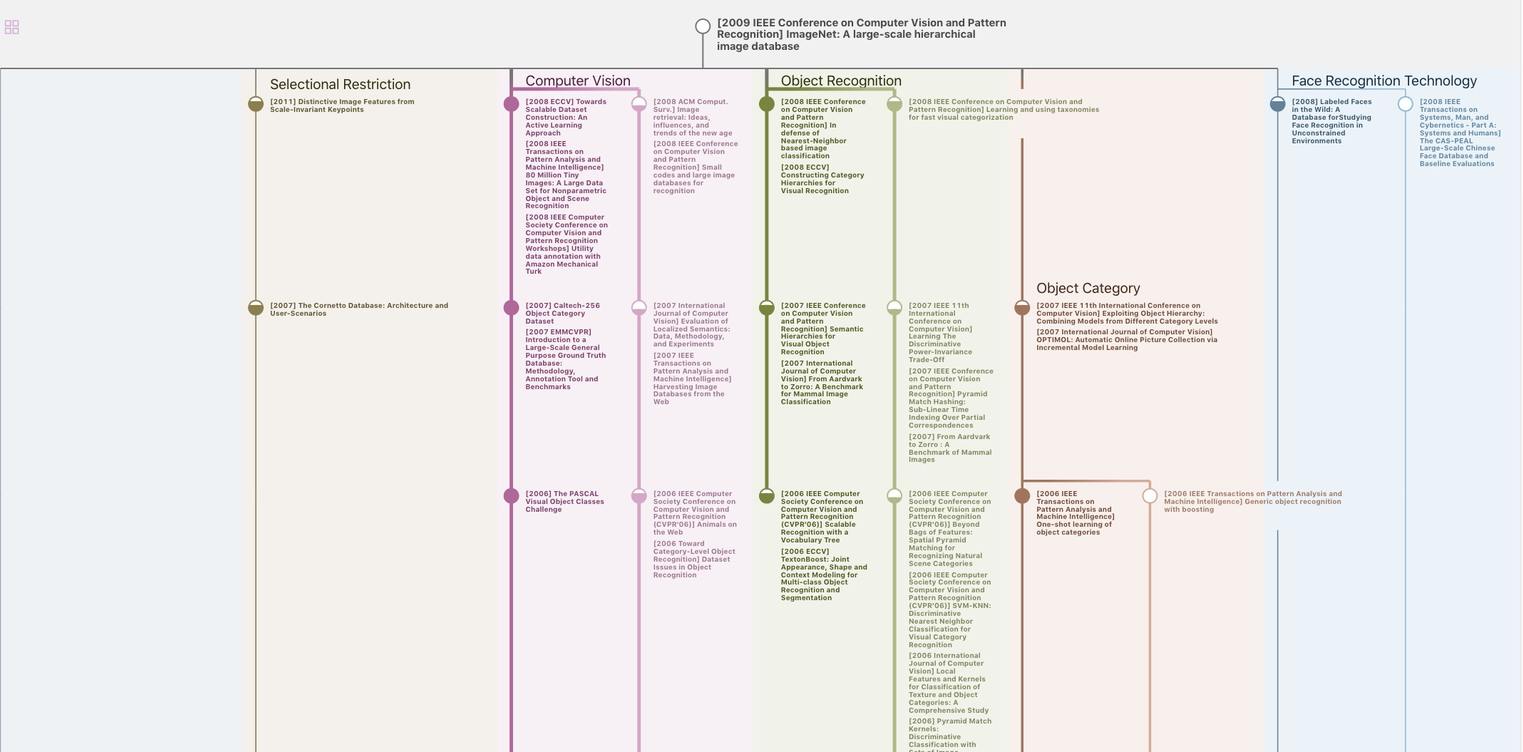
生成溯源树,研究论文发展脉络
Chat Paper
正在生成论文摘要