Aux-AIRL: End-to-End Self-Supervised Reward Learning for Extrapolating beyond Suboptimal Demonstrations
user-618b9067e554220b8f259598(2021)
摘要
Real-world human demonstrations are often suboptimal. How to extrapolate beyond suboptimal demonstration is an important open research question. In this ongoing work, we analyze the success of a previous state-of-the-art self-supervised reward learning method that requires four sequential optimization steps, and propose a simple end-toend imitation learning method Aux-ARIL that extrapolates from suboptimal demonstrations without requiring multiple optimization steps.
更多查看译文
AI 理解论文
溯源树
样例
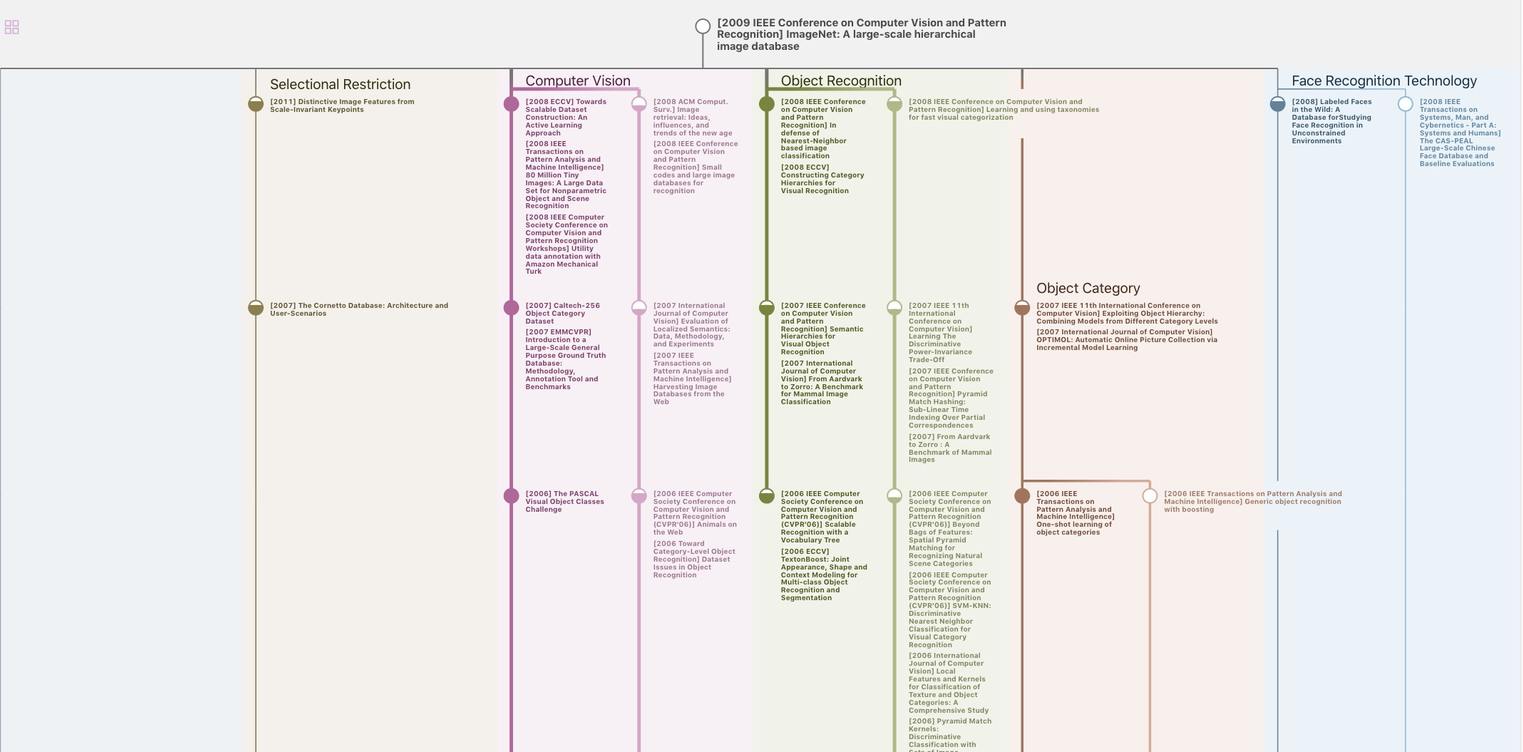
生成溯源树,研究论文发展脉络
Chat Paper
正在生成论文摘要