Two-stage short-term wind power forecasting algorithm using different feature-learning models
user-618b9067e554220b8f259598(2020)
摘要
Two-stage ensemble-based forecasting methods have been studied extensively in the wind power forecasting field. However, deep learning-based wind power forecasting studies have not investigated two aspects. In the first stage, different learning structures considering multiple inputs and multiple outputs have not been discussed. In the second stage, the model extrapolation issue has not been investigated. Therefore, we develop four deep neural networks for the first stage to learn data features considering the input-and-output structure. We then explore the model extrapolation issue in the second stage using different modeling methods. Considering the overfitting issue, we propose a new moving window-based algorithm using a validation set in the first stage to update the training data in both stages with two different moving window processes.Experiments were conducted at three wind farms, and the results demonstrate that the model with single input multiple output structure obtains better forecasting accuracy compared to existing models. In addition, the ridge regression method results in a better ensemble model that can further improve forecasting accuracy compared to existing machine learning methods. Finally, the proposed two-stage forecasting algorithm can generate more accurate and stable results than existing algorithms.
更多查看译文
关键词
Wind power forecasting,Deep neural networks,Ensemble learning,Extrapolation
AI 理解论文
溯源树
样例
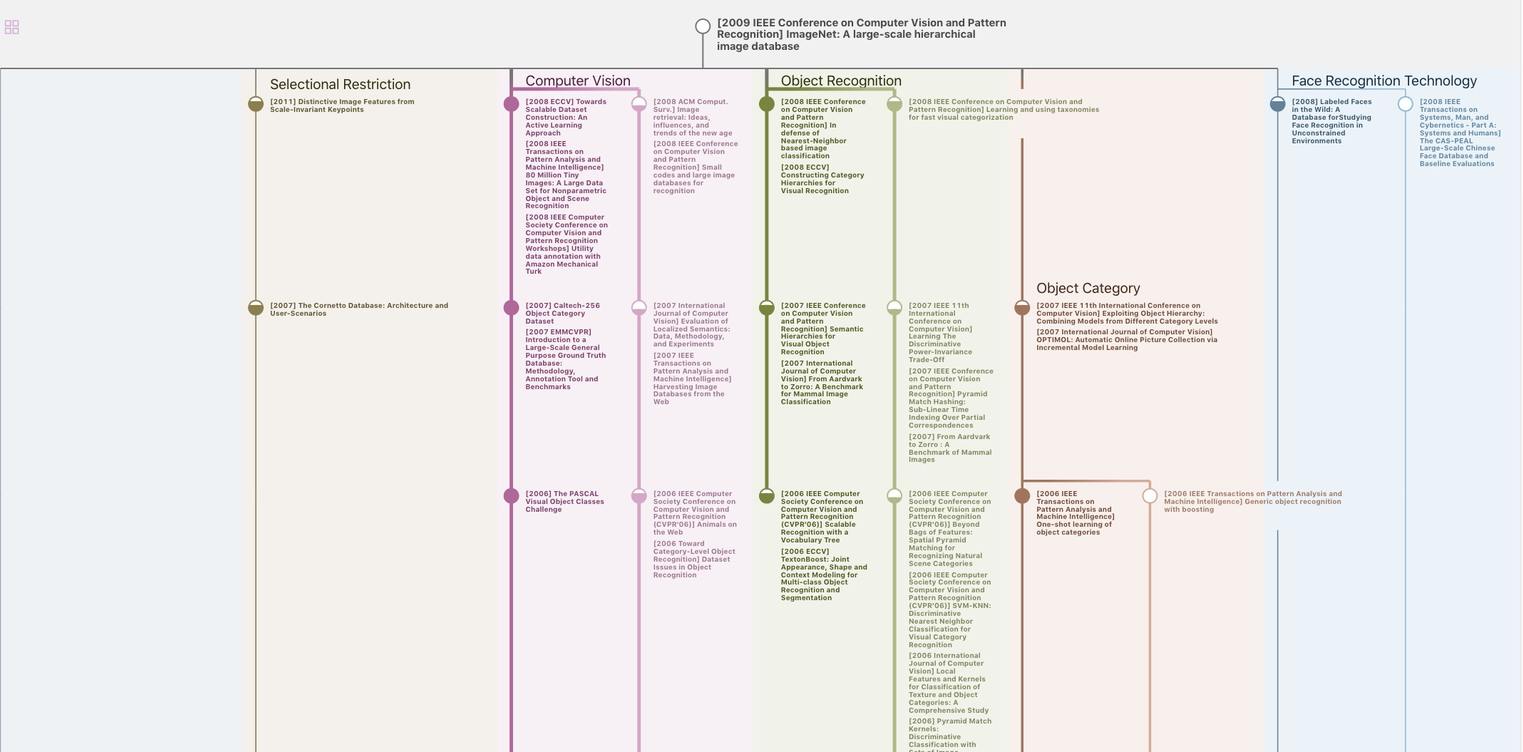
生成溯源树,研究论文发展脉络
Chat Paper
正在生成论文摘要