Safe Reinforcement Learning by Imagining the Near Future.
Annual Conference on Neural Information Processing Systems(2021)
摘要
Safe reinforcement learning is a promising path toward applying reinforcement learning algorithms to real-world problems, where suboptimal behaviors may lead to actual negative consequences. In this work, we focus on the setting where unsafe states can be avoided by planning ahead a short time into the future. In this setting, a model-based agent with a sufficiently accurate model can avoid unsafe states.We devise a model-based algorithm that heavily penalizes unsafe trajectories, and derive guarantees that our algorithm can avoid unsafe states under certain assumptions. Experiments demonstrate that our algorithm can achieve competitive rewards with fewer safety violations in several continuous control tasks.
更多查看译文
关键词
safe reinforcement learning,reinforcement learning,future
AI 理解论文
溯源树
样例
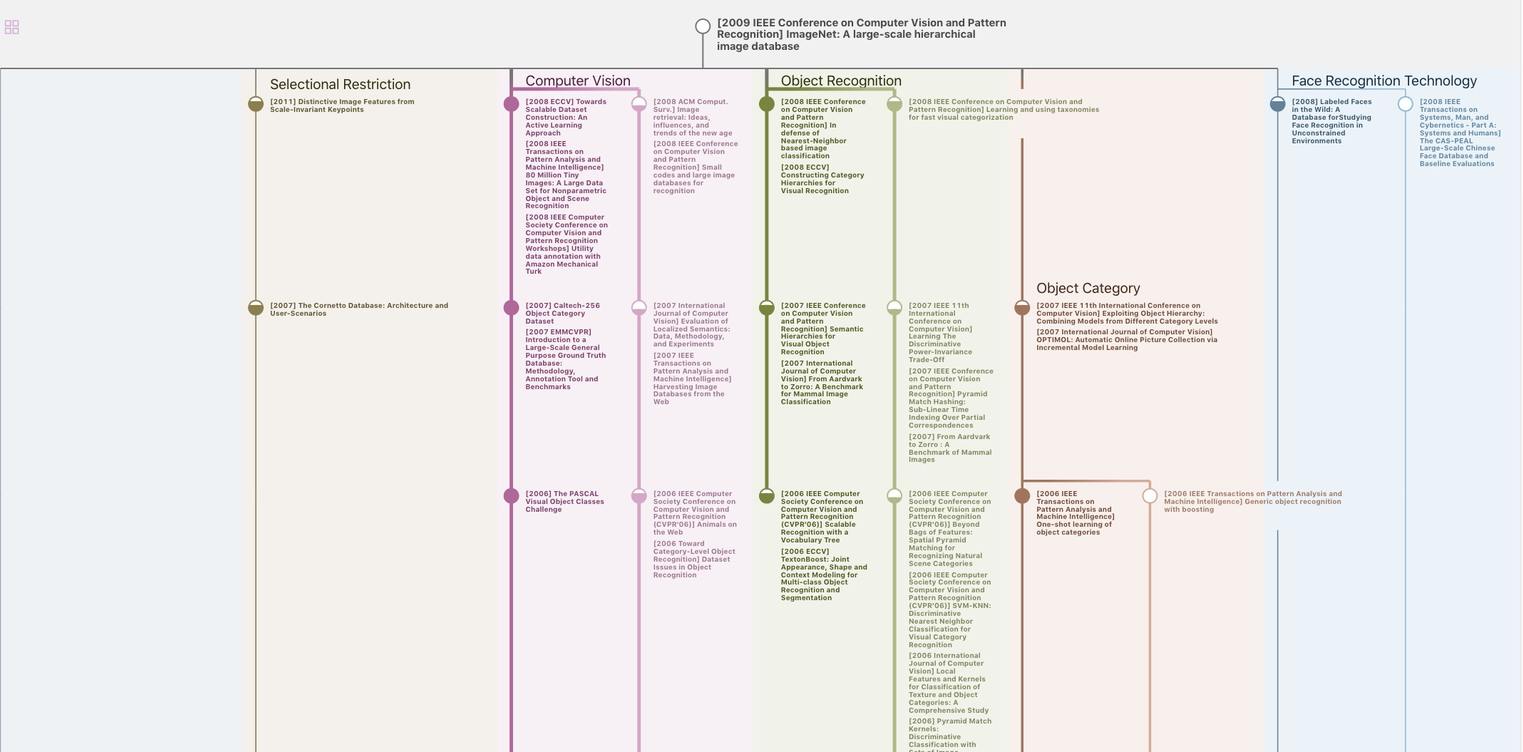
生成溯源树,研究论文发展脉络
Chat Paper
正在生成论文摘要