Marble: collaborative scheduling of batteryless sensors with meta reinforcement learning
Embedded Network Sensor Systems(2021)
摘要
ABSTRACTBatteryless energy-harvesting sensing systems are attractive for low maintenance but face challenges in real-world applications due to low quality of service from sporadic and unpredictable energy availability. To overcome this challenge, recent data-driven energy management techniques optimize energy usage to maximize application performance even in low harvested energy scenarios by learning energy availability patterns in the environment. These techniques require prior knowledge of the environment in which the sensor nodes are deployed to work correctly. In the absence of historical data, the application performance deteriorates. To overcome this challenge, we describe here the use of meta reinforcement learning to increase the application performance of newly deployed batteryless sensor nodes without historical data. Our system, called Marble, exploits information from other sensor node locations to expedite the learning of newly deployed sensor nodes, and improves application performance in the initial period of deployment. Our evaluation using real-world data traces shows that Marble detects up to 66% more events in low lighting conditions, and up to 25.6% more events on average on the first 3 days of deployment compared to the state-of-the-art.1
更多查看译文
关键词
Batteryless, Wireless Sensor Network, Energy Harvesting, Smart Buildings, Deep Reinforcement Learning, Collaborative Learning, Perpetual Operations
AI 理解论文
溯源树
样例
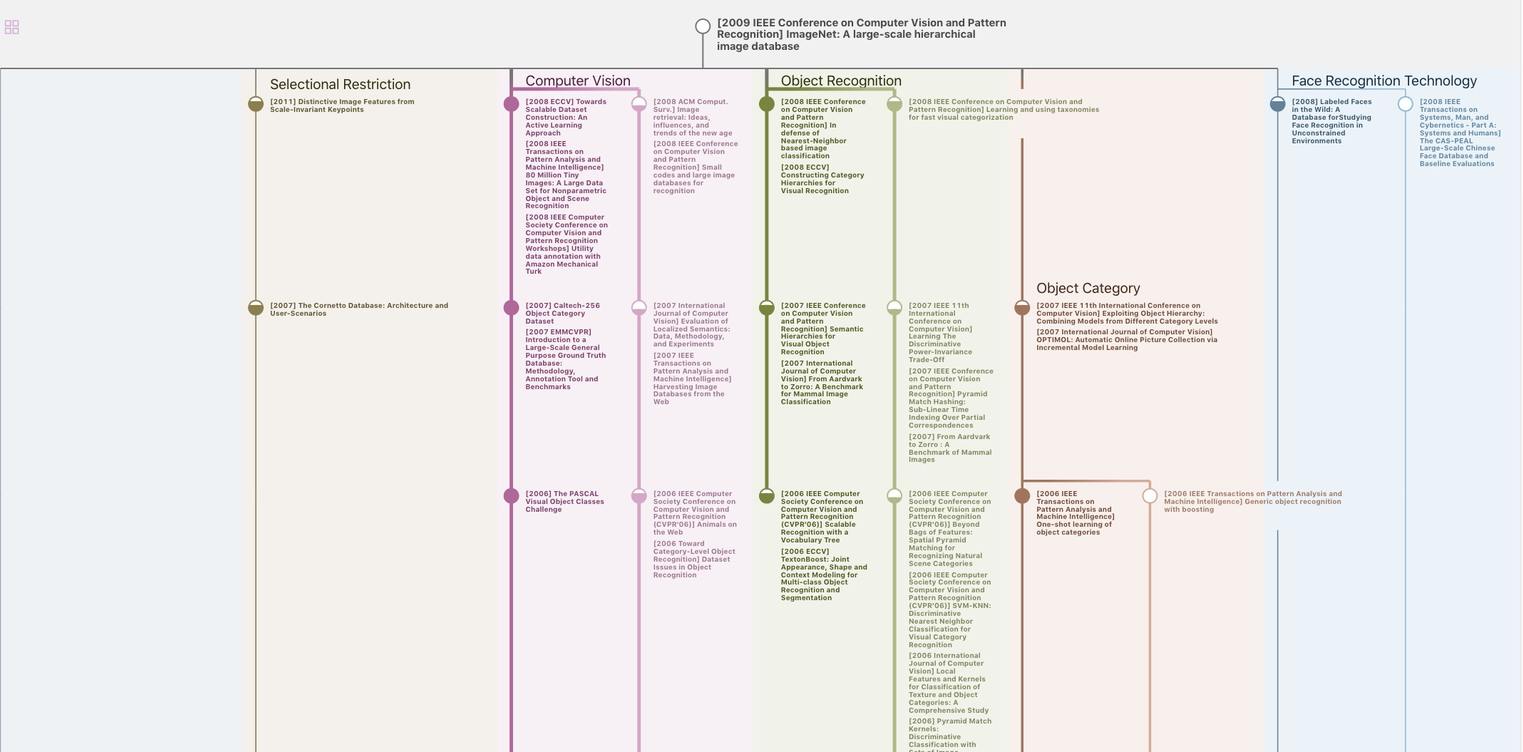
生成溯源树,研究论文发展脉络
Chat Paper
正在生成论文摘要