QUILT: quantify, infer and label the thermal efficiency of heating and cooling residential homes
Embedded Network Sensor Systems(2021)
摘要
ABSTRACTThe European Parliament have set a target of at least a 32.5% improvement in energy efficiency by 2030. In the EU residential sector, heating and cooling account for half of the energy usage alone, hence there is a need to make homes more thermally efficient. However, there are currently a lack of tools to evaluate the thermal efficiency of homes at scale as previous methods have been intrusive, costly, or inaccurate. More recent approaches have shown that the thermal efficiency of homes can be inferred with non-intrusive methods using only smart-meter and weather data. However, to deploy such algorithms at scale, steps must be taken to quantify in which scenarios it works, and to account for the uncertainty of the inferred thermal efficiency. To address this, we propose QUILT, a non-intrusive, data-driven framework to quantify the thermal efficiency of a home, grounded in physical models of heat flow, which works with heating and cooling systems. A Bayesian model is used to account for the uncertainty associated with data sources and parameters in the thermal model. QUILT is evaluated on real data to demonstrate its feasibility in real world scenarios, and also on simulated data used by the U.S. Department of Energy to evaluate the performance in scenarios not currently captured in real-world datasets. Our experiments quantify the improvements compared to the current state-of-the-art approach by reducing the mean absolute percentage error from 21.6% to 15.6% when inferring the heating power loss coefficient (HPLC), which defines how efficiently a building maintains a heated state given the external temperature. Furthermore, the term cooling power loss coefficient (CPLC) is introduced which is the equivalent to HPLC when the building is in a cooled state, and the feasibility of inferring this metric with QUILT is shown. The analysis also highlights the importance of identifying when the heating is on, and how the HPLC or CPLC can be inferred within a week if the occupants are on holiday given the right weather conditions.
更多查看译文
关键词
Thermal efficiency, smart meters, thermal modelling
AI 理解论文
溯源树
样例
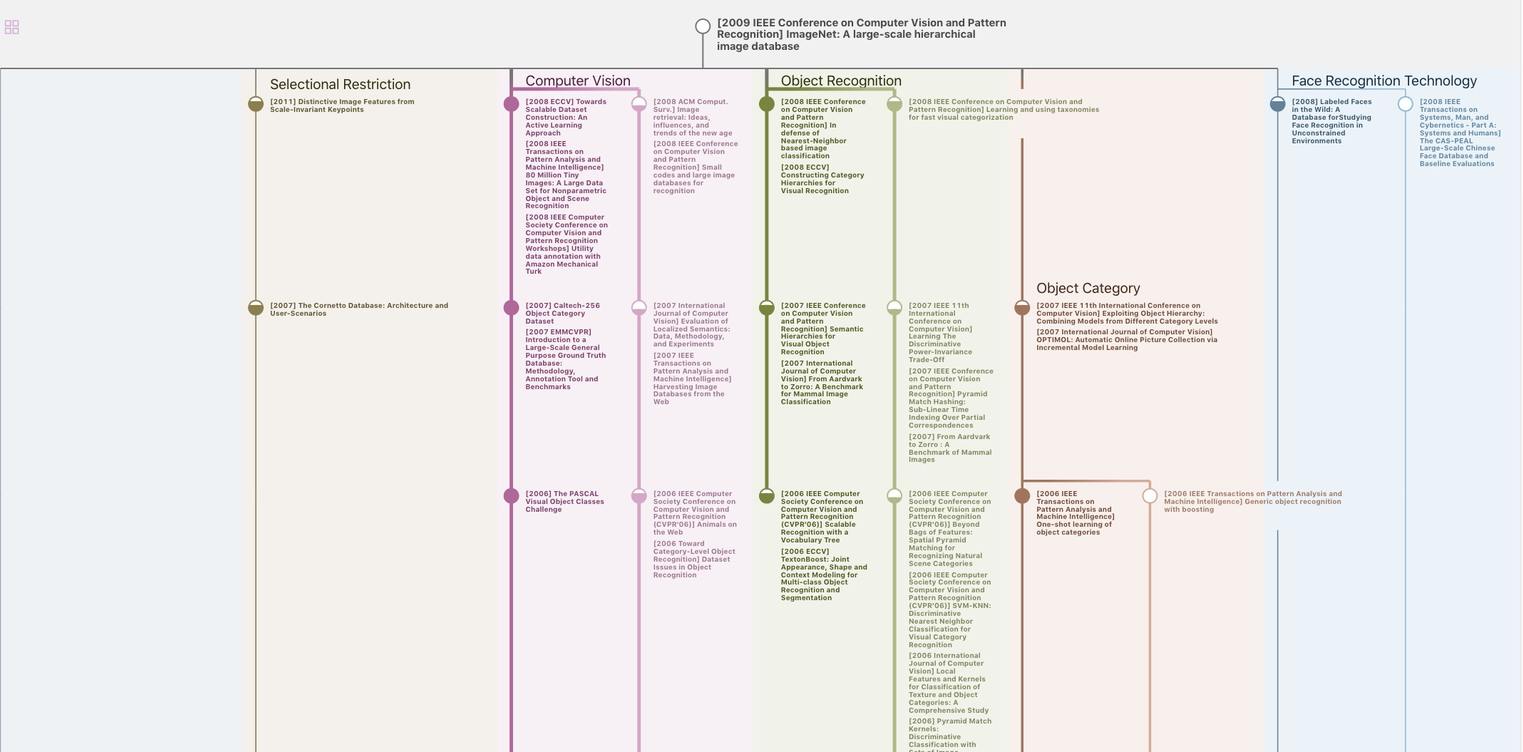
生成溯源树,研究论文发展脉络
Chat Paper
正在生成论文摘要