Core-Concept-Seeded LDA for Ontology Learning
KNOWLEDGE-BASED AND INTELLIGENT INFORMATION & ENGINEERING SYSTEMS (KSE 2021)(2021)
摘要
Ontologies are powerful semantic models applied for various purposes such as improving system interoperability, information retrieval, question answering, etc. However, building domain ontologies remains a challenging task for humans, especially when the concepts and properties are large or evolving, and also when they are built from large-scale textual data. Machine learning allows to automate the building of ontologies from texts. In particular, clustering techniques have a promising ability on the concept formation task by identifying the cluster of semantically closed terms as a concept. However, current works encounter issues in learning relevant domain-specific clusters or in identifying the relevant concept labels for each cluster. To solve these issues, we propose both to use core concepts from a domain ontology as prior knowledge, and to adapt term clustering with seed knowledge-based LDA models in order to take these core concepts into account. First, each topic is associated with a set of seed terms of a single core concept, then the learning is guided by these seeds to gather in the same topic the terms that refer to its core concept. We evaluate our proposal on two textual corpora and compare it to the baselines (LDA, K-means, and SMBM). The results show that our approach performs significantly better than other methods on the class-balanced dataset and works well on the class-imbalanced dataset with a proper number of topics for each core concept. (C) 2021 The Authors. Published by Elsevier B.V. This is an open access article under the CC BY-NC-ND license (https://crativecommons.org/licenses/by-nc-nd/4.0) Peer-review under responsibility of the scientific committee of KES International.
更多查看译文
关键词
Ontology Learning, Core Ontology, LDA, Term Clustering, Seed Knowledge, Prior Knowledge, Semantic Coherence, Word2vec
AI 理解论文
溯源树
样例
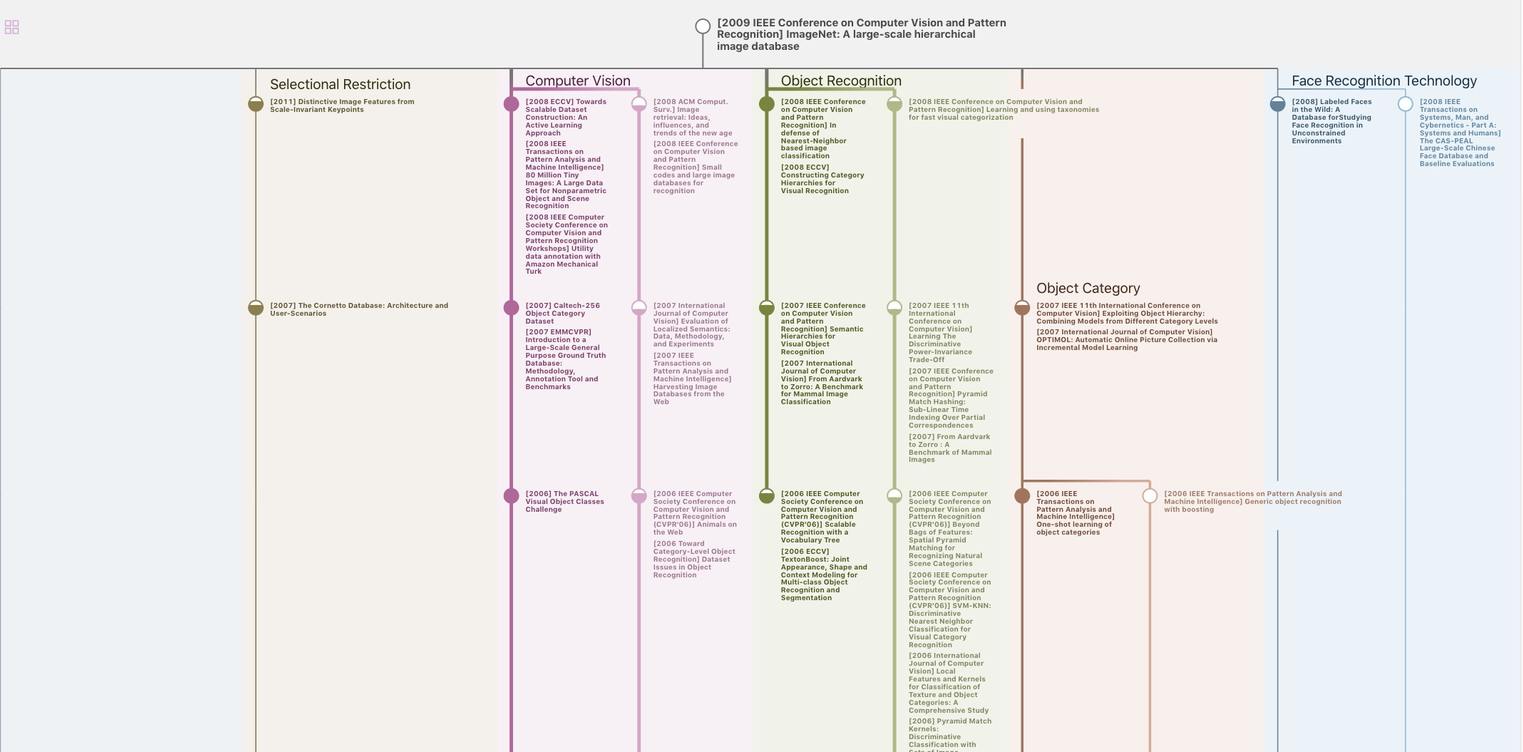
生成溯源树,研究论文发展脉络
Chat Paper
正在生成论文摘要