BE-BLC: BERT-ELMO-Based Deep Neural Network Architecture for English Named Entity Recognition Task
KNOWLEDGE-BASED AND INTELLIGENT INFORMATION & ENGINEERING SYSTEMS (KSE 2021)(2021)
摘要
One of the most important factors which considerably affects the quality of the neural sequence labeling model is the selection and encoding of input features to generate rich semantic and grammatical word representation vectors. Recently, pre-training text encoders have significantly ameliorated the performance on various Natural Language Processing (NLP) tasks. In this paper, we introduce a deep neural network model to address a challenging task of the sequence labeling problem, the task of named entity recognition. The model consists of three sub-networks to fully exploit current Google's representations of the most powerful NLP transfer learning BERT and ELMO model, as well as the traditional state-of-the-art BiLSTM-CRF (BLC) model. The model outperforms the state-of-the-art systems on CoNLL-2003 by achieving a 95.56% F1-score and getting results comparable to the previous best-performing system models on OntoNotes 5.0 by achieving an 89.21% F1-score without using any external knowledge. (C) 2021 The Authors. Published by Elsevier B.V. This is an open access article under the CC BY-NC-ND license (https://crativecommons.org/licenses/by-nc-nd/4.0) Peer-review under responsibility of the scientific committee of KES International.
更多查看译文
关键词
Named entity recognition, Deep learning, BERT, ELMO, Transfer learning, Bi-directional long short-term memory, Conditional random field
AI 理解论文
溯源树
样例
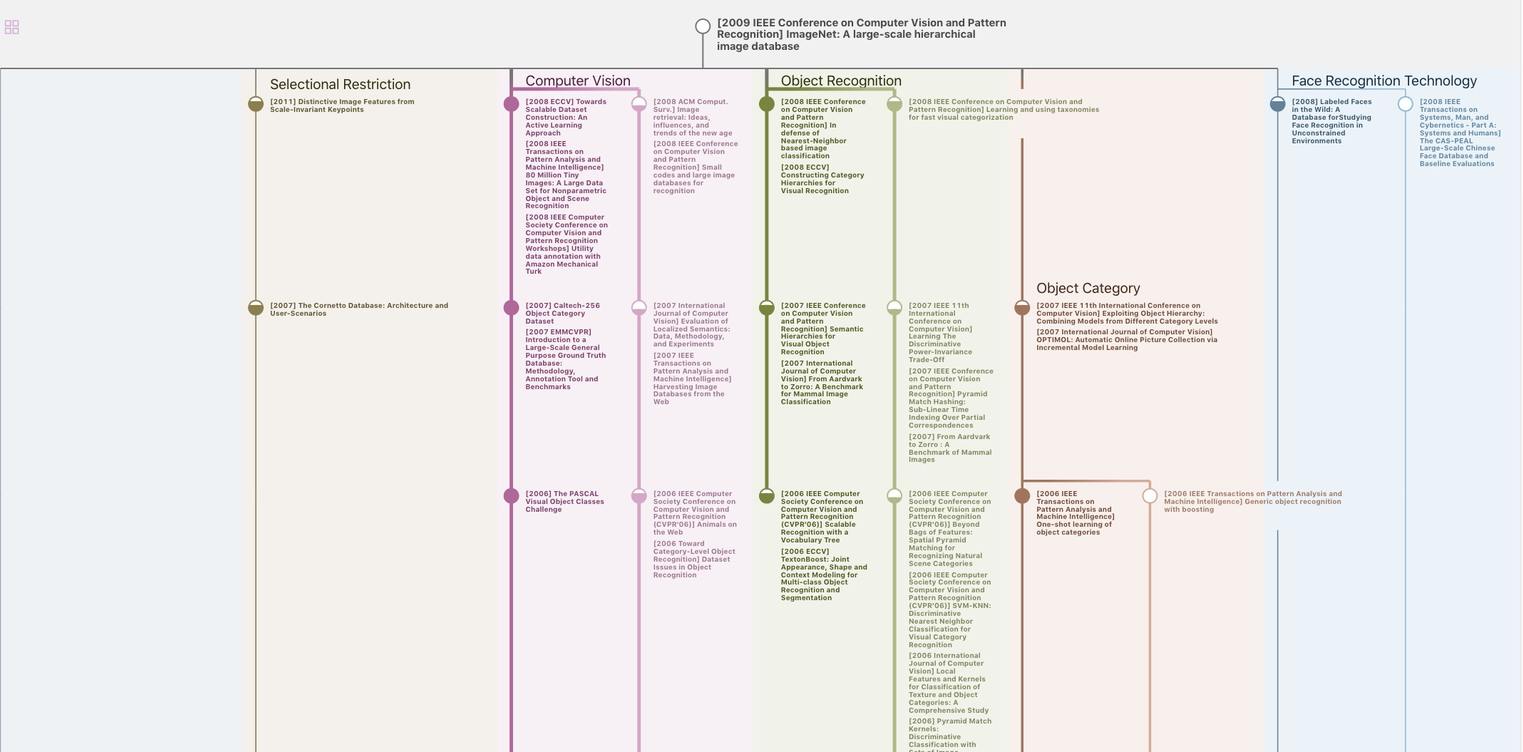
生成溯源树,研究论文发展脉络
Chat Paper
正在生成论文摘要