Quantitative assessment of essential tremor based on machine learning methods using wearable device
BIOMEDICAL SIGNAL PROCESSING AND CONTROL(2022)
摘要
Background: Essential tremor (ET) is a progressive neurological disorder with characteristic motor symptoms. Current clinical assessments are primarily based on expert consultation combined with reviewing patient complaints and physician expertise and diagnostic experience. Research on objective quantification through wearable sensor technology combined with machine learning methods has excellent potential for application. This study automatically rates the severity of symptoms in ET patients using wearable sensors in a standardized scenario. Methods: This study relied on a rigorous clinical trial paradigm and a wearable device based on a nine-axis Inertial Measurement Unit (IMU) to collect a large amount of kinematic data from ET patients and obtain professional physician-supported scale scores. In this paper, hand tremor signals were comprehensively analyzed, and multiple kinematic features in the time and frequency domains were extracted. These features were used to explore different machine learning approaches to automatically and quantitatively assess the disease severity in ET patients. Results: The optimized algorithm AdaBoost has a multi-classification F1 score of up to 97.33%, with 99.64% accuracy and 99.39% specificity, respectively. The model still has a better AUC for predicting a few classes and has the current optimal automatic ET symptom recognition performance. Discussion: These results show that the proposed method is suitable for applying standardized laboratory tests to help clinicians automate the scoring of complex or early ET cases to aid decision-making and improve disease management efficiency.
更多查看译文
关键词
Essential Tremor, Wearable Sensor, Rating of Severity, Machine Learning
AI 理解论文
溯源树
样例
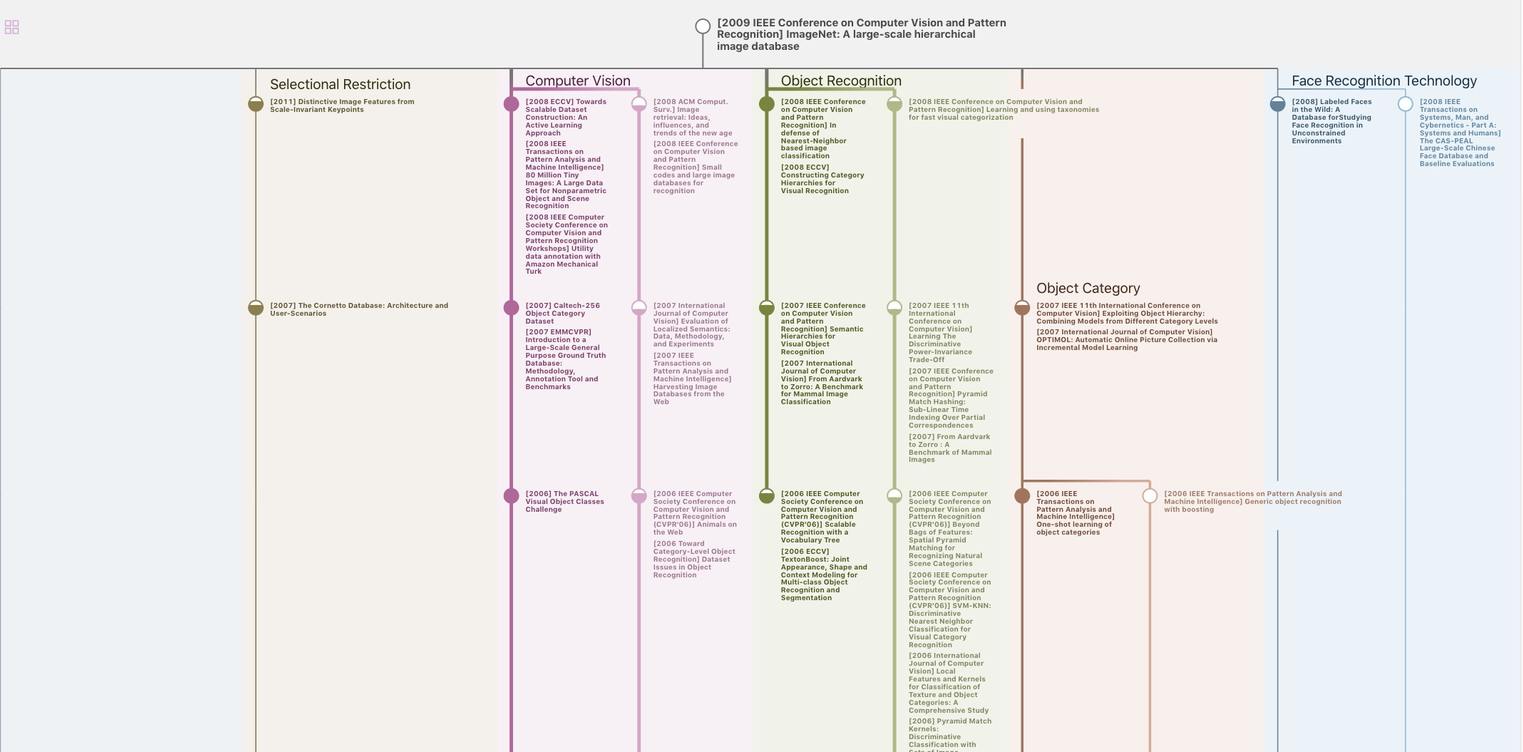
生成溯源树,研究论文发展脉络
Chat Paper
正在生成论文摘要