Analyzing the impact of class transitions on the design of pattern recognition-based myoelectric control schemes
BIOMEDICAL SIGNAL PROCESSING AND CONTROL(2022)
摘要
Despite continued efforts to improve classification accuracy, it has been reported that offline accuracy is a poor indicator of the usability of pattern recognition-based myoelectric control. One potential source of this disparity is the existence of transitions between contraction classes that happen during regular use and are reported to be problematic for pattern recognition systems. Nevertheless, these transitions are often ignored or undefined during both the training and testing processes. In this work, we propose a set of metrics for analyzing the transitions that occur during the voluntary changes between contraction classes during continuous control. These metrics quantify the common types of errors that occur during transitions and compare them to existing metrics that apply only to the steady-state portions of the data. We then use these metrics to analyze transition char-acteristics of 6 commonly used classifiers on a novel dataset that includes continuous transitions between all combinations of seven different contraction classes. Results show that a linear discriminant classifier consistently outperforms other conventional classifiers during both transitions and steady-state conditions, despite having an almost identical offline performance. Results also show that, although offline training metrics correlate with steady-state performance, they do not correlate with transition performance. These insights suggest that the proposed set of metrics could provide a shift in perspective on the way pattern recognition systems are evaluated and provide a more representative picture of a classifier's performance, potentially narrowing the gap between offline performance and online usability.
更多查看译文
关键词
Surface Electromyography (SEMG), SEMG pattern recognition, Transition metrics, Usability, Offline accuracy
AI 理解论文
溯源树
样例
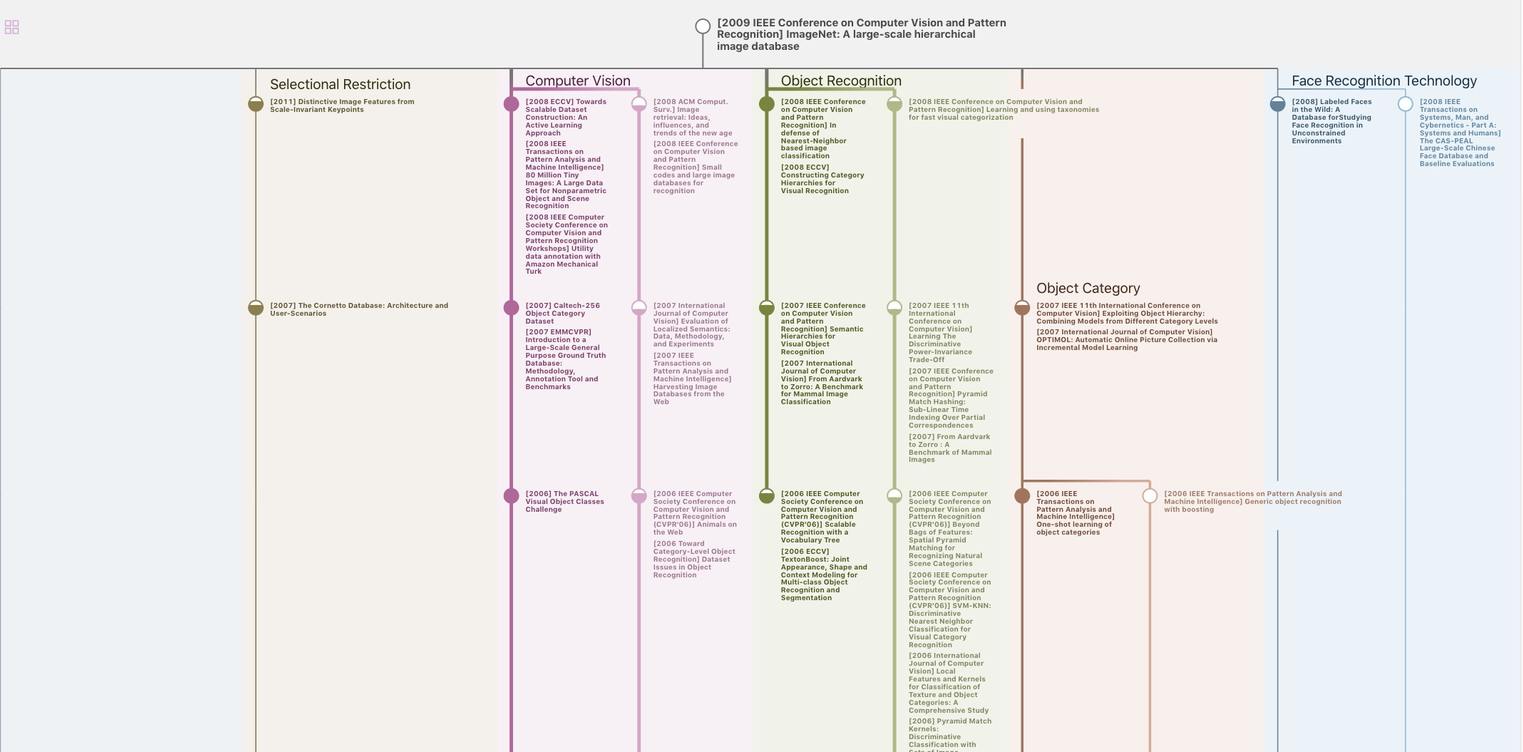
生成溯源树,研究论文发展脉络
Chat Paper
正在生成论文摘要