Automatic detection of obstructive and restrictive lung disease from features extracted from ECG and ECG derived respiration signals
BIOMEDICAL SIGNAL PROCESSING AND CONTROL(2022)
摘要
Early detection of both obstructive and restrictive lung diseases is essential for maintaining proper lung function, which plays a pivotal role in decreasing the escalating number of deaths worldwide. Even though conventional methods are vastly used for diagnosing these two diseases, their use is often associated with high cost, low comfort, high patient-effort dependency, and interference with natural breathing. Consequently, the development of a non-invasive, inexpensive, reliable, and comfortable technique for detecting these respiratory diseases has become an important area of research. Therefore, unobtrusive and automatic identification of these two pulmonary diseases based on ECG and ECG derived respiration (EDR) has been presented in this study. Temporal information was extracted from the morphological variations observed in both ECG and EDR signals to derive distinctive features. Finally, supervised classifiers were used for differentiating the subjects into normal, obstructive, and restrictive categories. Performance of the classifiers evaluated on 90 subjects (both normal and diseased) demonstrated classification accuracy of more than 98 %. Results indicate that the proposed method can be effectively utilized for primary identification of obstructive and restrictive lung diseases.
更多查看译文
关键词
ECG derived respiration, K-Nearest Neighbor, Naive Bayes, Obstructive lung disease, Restrictive lung disease, Support vector machine
AI 理解论文
溯源树
样例
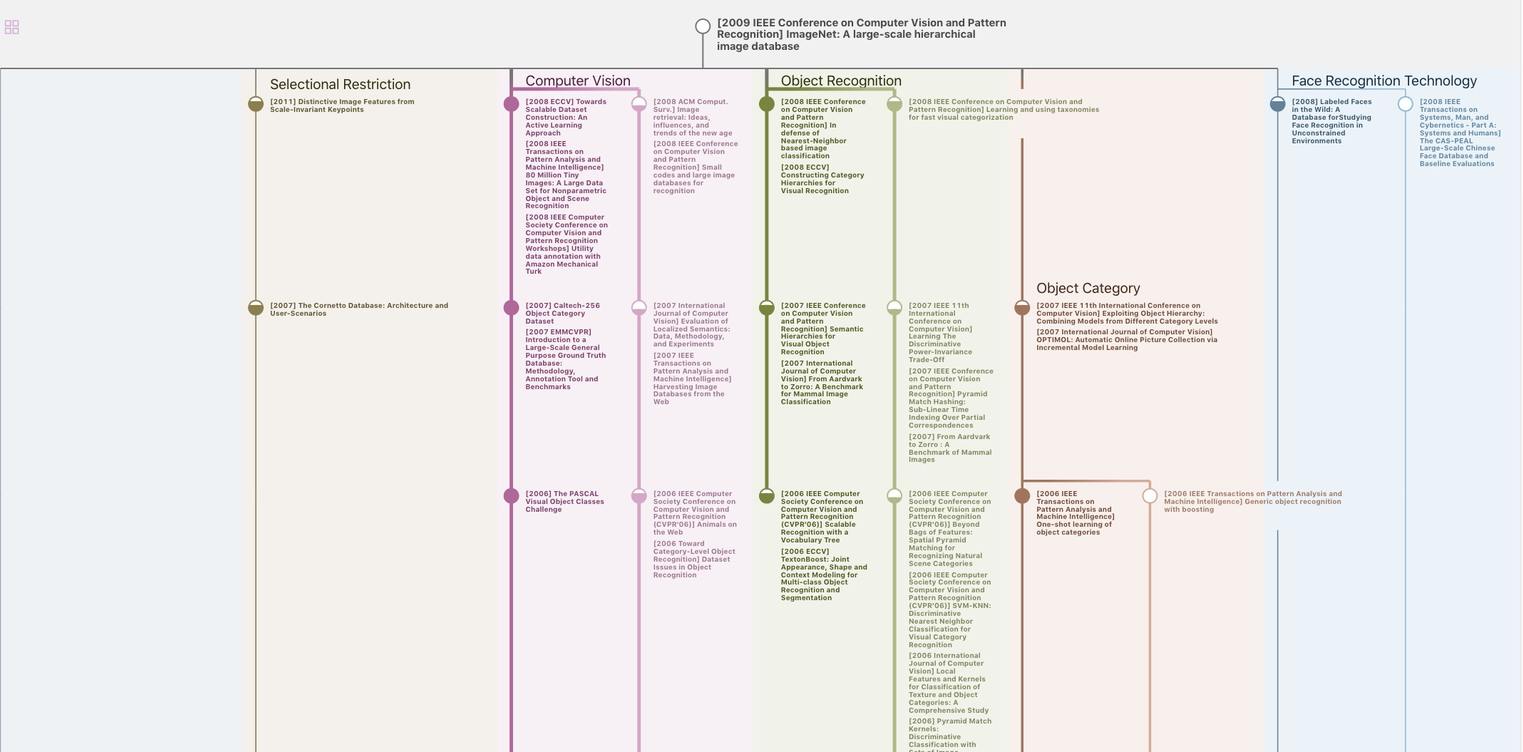
生成溯源树,研究论文发展脉络
Chat Paper
正在生成论文摘要