A 9.0-TOPS/W Hash-Based Deep Neural Network Accelerator Enabling 128× Model Compression in 10-nm FinFET CMOS
IEEE Solid-State Circuits Letters(2020)
摘要
A 10-nm DNN inference accelerator compresses model size with tabulation hash-based line-grained weight sharing and increases 8bcompute density by 3.4× to 1.6 TOPS/mm
2
. The compressed model DNN implements lightweight hashing circuits to compress fully connected and recurrent neural networks. Optimized shared weight address generation reduces MUX tree area overhead by 40%. Runtime hash table generation and weight mapping circuits enable a peak energy efliciency of 9.0 TOPS/W at 450 mV, 25°C. A 128×-compressed 3-layer long shortterm memory classilies TIMIT phonemes with 85.6% accuracy for a total energy of 14 μJ/classilication, with <; 0.5% degradation in accuracy over an uncompressed network.
更多查看译文
关键词
Deep learning ASIC,deep neural networks (DNNs),hashing,inference,long short-term memory (LSTM),model compression,recurrent neural network (RNN),speech recognition
AI 理解论文
溯源树
样例
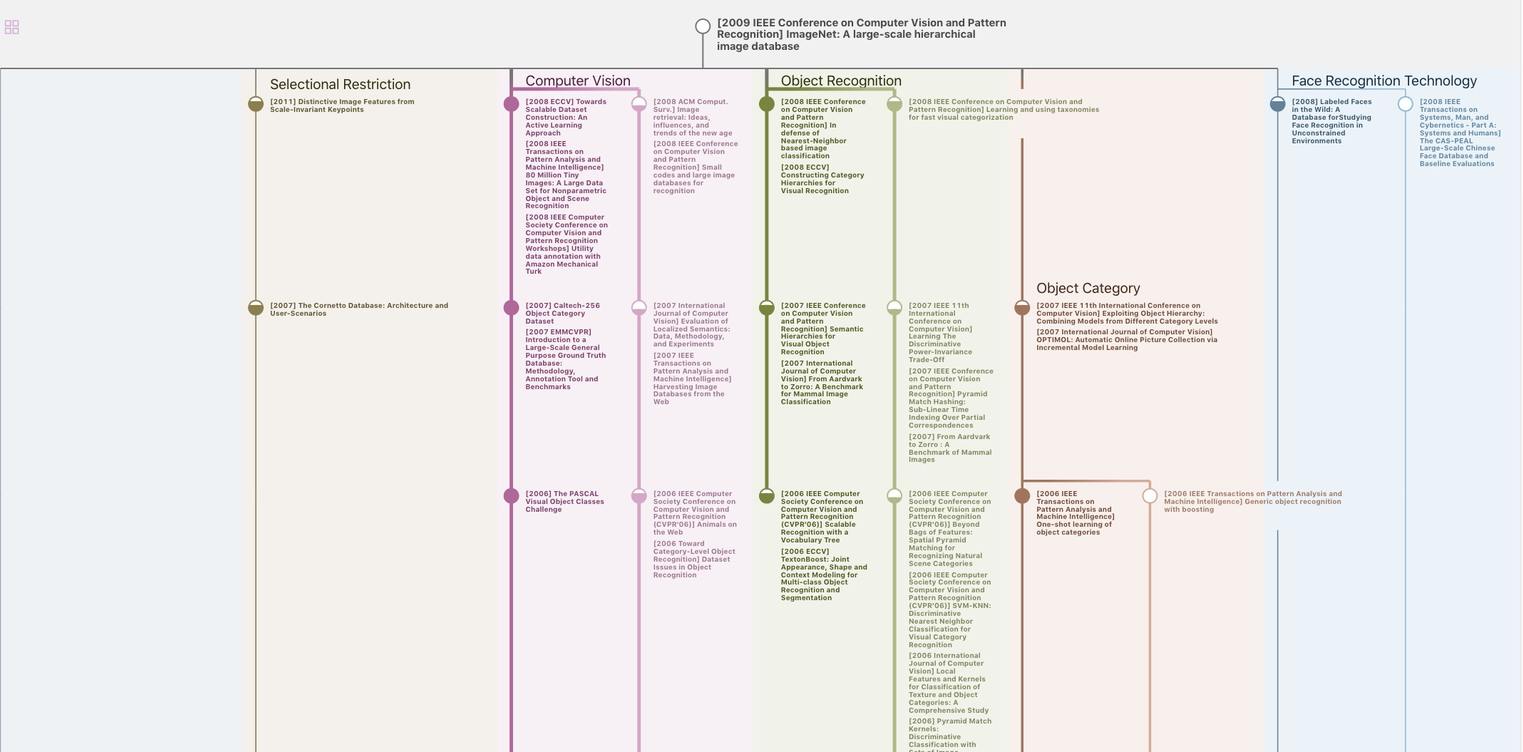
生成溯源树,研究论文发展脉络
Chat Paper
正在生成论文摘要