TiWS-iForest: Isolation forest in weakly supervised and tiny ML scenarios
Information Sciences(2022)
摘要
Unsupervised anomaly detection tackles the problem of finding anomalies inside datasets without the labels availability; since data tagging is typically hard or expensive to obtain, such approaches have seen huge applicability in recent years. In this context, Isolation Forest is a popular algorithm able to define an anomaly score by means of an ensemble of peculiar trees called isolation trees. These are built using a random partitioning procedure that is extremely fast and cheap to train. However, we find that the standard algorithm might be improved in terms of memory requirements, latency and performances; this is of particular importance in low resources scenarios and in TinyML implementations on ultra-constrained microprocessors. Moreover, Anomaly Detection approaches currently do not take advantage of weak supervisions: being typically consumed in Decision Support Systems, feedback from the users, even if rare, can be a valuable source of information that is currently unexplored. Beside showing iForest training limitations, we propose here TiWS-iForest, an approach that, by leveraging weak supervision is able to reduce Isolation Forest complexity and to enhance detection performances. We showed the effectiveness of TiWS-iForest on real word datasets and we share the code in a public repository to enhance reproducibility.
更多查看译文
关键词
Anomaly detection,Decision support systems,Isolation forest,Outlier detection,TinyML,Weakly supervised
AI 理解论文
溯源树
样例
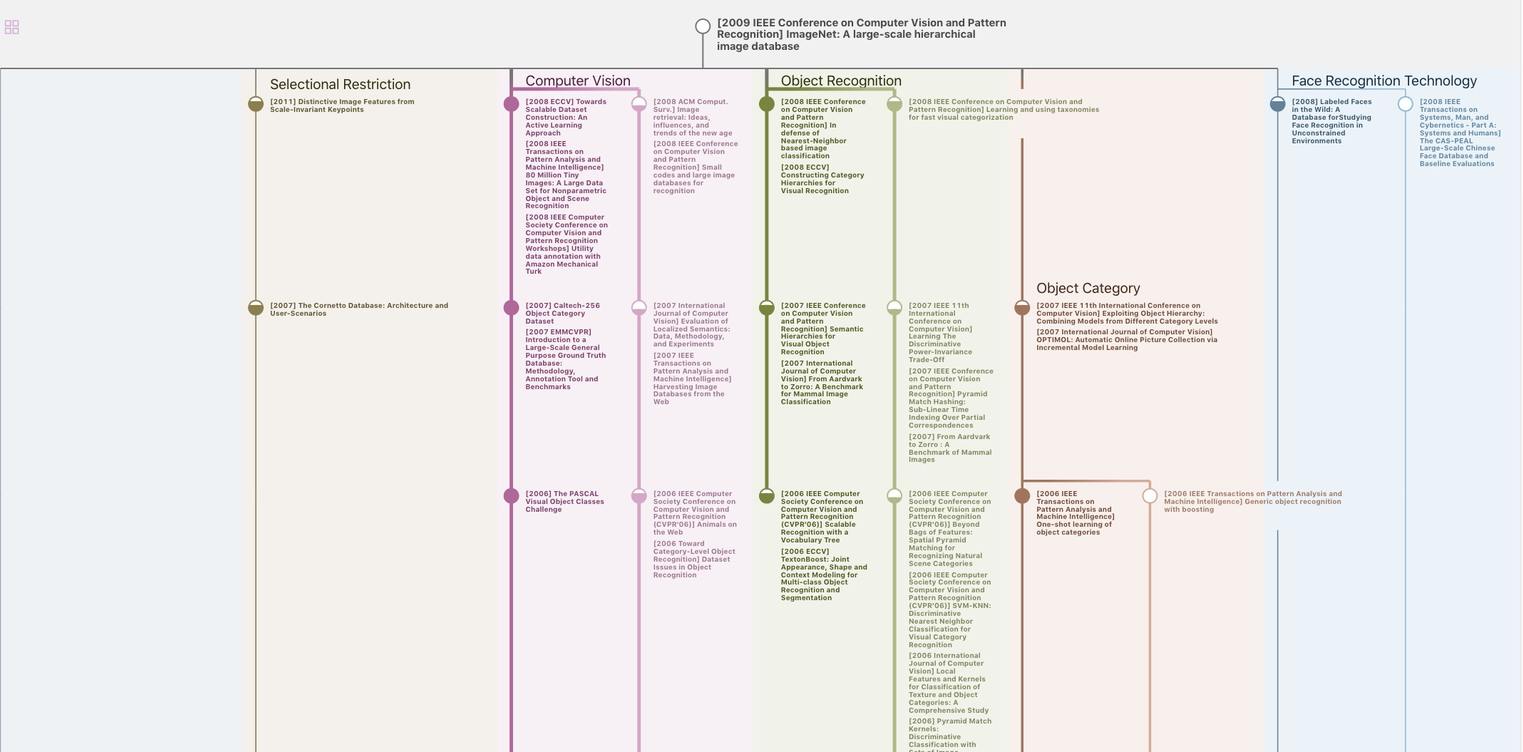
生成溯源树,研究论文发展脉络
Chat Paper
正在生成论文摘要