Seek Common Ground While Reserving Differences: A Model-Agnostic Module for Noisy Domain Adaptation
IEEE Transactions on Multimedia(2022)
摘要
Noisy domain adaptation aims to solve the problem that the source dataset contains noisy labels in domain adaptation. Previous methods handle noisy labels by selecting the small-loss samples with inconsistent predictions between two models and discarding the consistent samples, resulting in many noises contained in the selected samples. By jointly considering the consistent and inconsistent samples, we propose a model-agnostic module, named Seek Common Ground While Reserving Differences (SCGWRD), to reduce the impact of noisy samples. The proposed SCGWRD module consists of Seek Common Ground (SCG) component and Reserve Differences (RD) component by utilizing the outputs of two symmetrical domain adaptation models. As the common samples with consistent predictions between two models are more likely to be clean samples, the SCG component applies the small-loss strategy to select the reliable samples with consistent predictions. Unlike SCG, the RD component maintains the divergences between two models with mutual learning and reduces the effect of noisy data using the samples with different predictions and small losses. Evaluations on three benchmarks demonstrate the effectiveness and robustness of the proposed SCGWRD module for noisy domain adaptation.
更多查看译文
关键词
Noisy domain adaptation,Seek common ground component,Reserve differences component
AI 理解论文
溯源树
样例
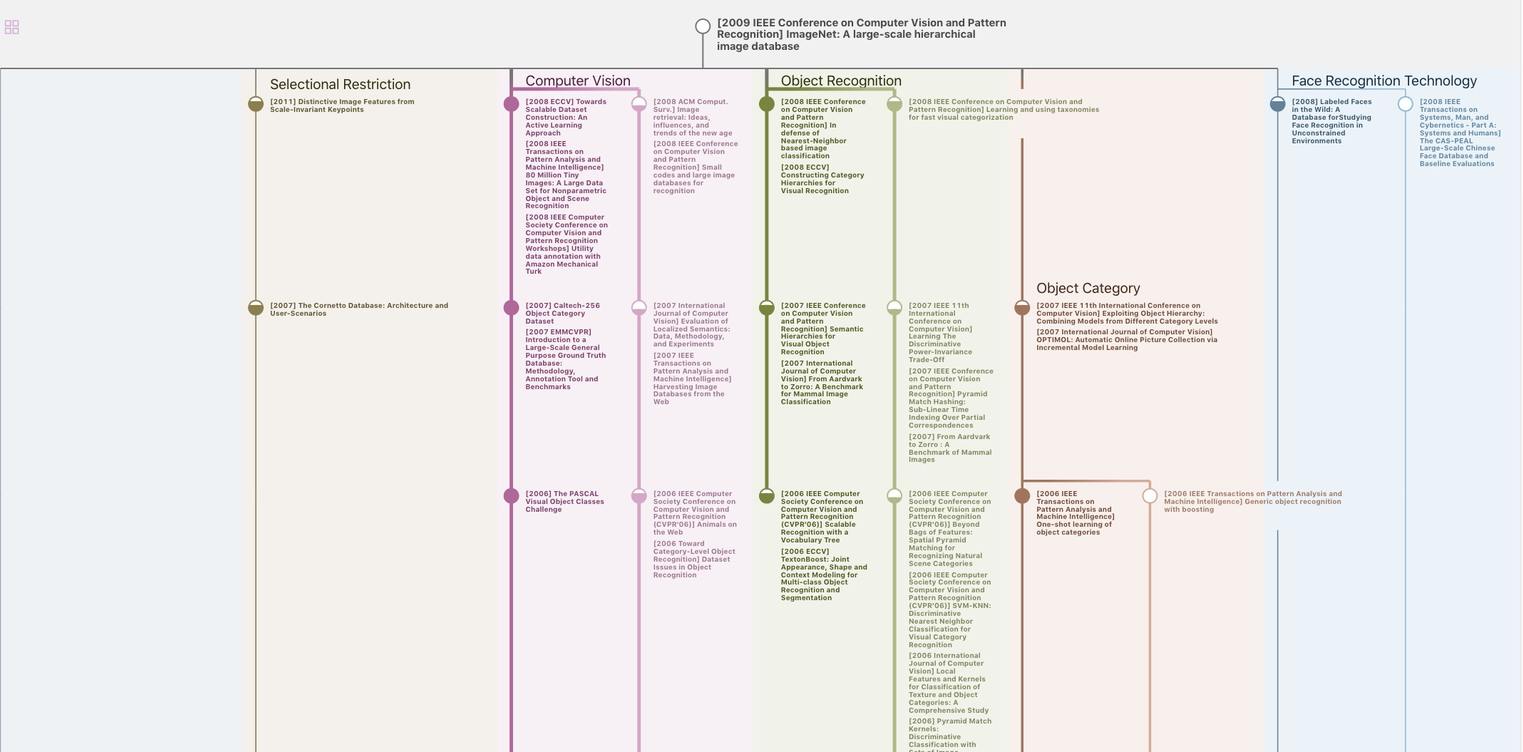
生成溯源树,研究论文发展脉络
Chat Paper
正在生成论文摘要